Machine Learning Based Classification Accuracy of Encrypted Service Channels: Analysis of Various Factors
JOURNAL OF NETWORK AND SYSTEMS MANAGEMENT(2020)
摘要
Visibility into network traffic is a key requirement for different security and network monitoring tools. Recent trends in the evolution of Internet traffic present a challenge for traditional traffic analysis methods to achieve accurate classification of Internet traffic including Voice over IP (VoIP), text messaging, video, and audio services among others. A key aspect of this trend is the rising levels of encrypted multiple service channels where the payload is opaque to middleboxes in the network. In such scenarios, traditional approaches such as Deep Packet Inspection (DPI) or examination of Port numbers are unable to achieve the classification accuracy required. This work investigates Machine Learning-based network traffic classifiers as a means of accurately classifying encrypted multiple service channels. The study carries out a thorough study which (i) proposes and evaluates two machine learning-based frameworks for multiple service channels analysis; (ii) undertakes feature engineering to identify the minimum number of features required to obtain high accuracy while reducing the effects of over-fitting; (iii) explores the portability and robustness of the frameworks trained models under different network conditions: location, time, and volume; and (iv) collects and analyzes a large-scale dataset including nine classes of services, for benchmarking purposes.
更多查看译文
关键词
Multiple service channels,Encrypted traffic classification,Encrypted traffic analysis,Feature selection,Robust traffic classifier,Machine Learning based traffic analysis
AI 理解论文
溯源树
样例
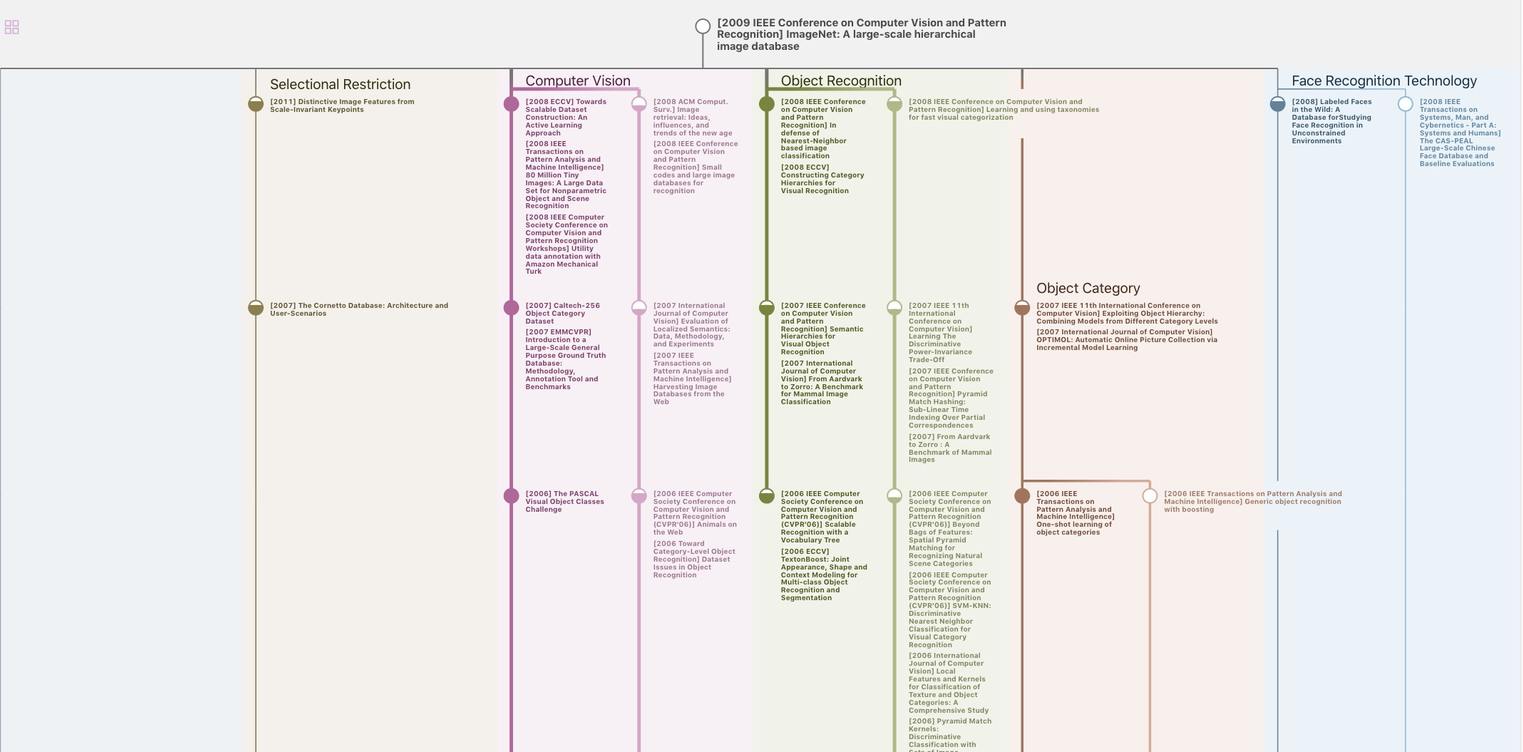
生成溯源树,研究论文发展脉络
Chat Paper
正在生成论文摘要