Extreme clustering – A clustering method via density extreme points
Information Sciences(2021)
摘要
Peak clustering, a density based clustering method, has shown remarkable performance in clustering analysis of data. In reality, peak clustering suffers from two major drawbacks: (i) when the difference in cluster sample density is significant, it becomes difficult for peak clustering to find cluster centres in low density clusters. (ii) in some cases, it will incorrectly detect many normal points as noises. In this paper, we propose a new extreme clustering method to overcome the drawbacks of peak clustering. The theme of extreme clustering is to identify density extreme points to find cluster centres. In addition, a noise detection module is also introduced to identify noisy data points from the clustering results. As a result, the extreme clustering is robust to datasets with different density distributions. Experiments and validations, on over 40 datasets, show that extreme clustering can not only inherit the cluster validity of peak clustering, but also overcome its shortages with significant performance gain. Case studies on real-world haze analysis also demonstrate the performance of extreme clustering method in finding some main haze origins in a Chinese city.
更多查看译文
关键词
Density peak clustering,Extreme point,Density,Clustering,Haze analysis
AI 理解论文
溯源树
样例
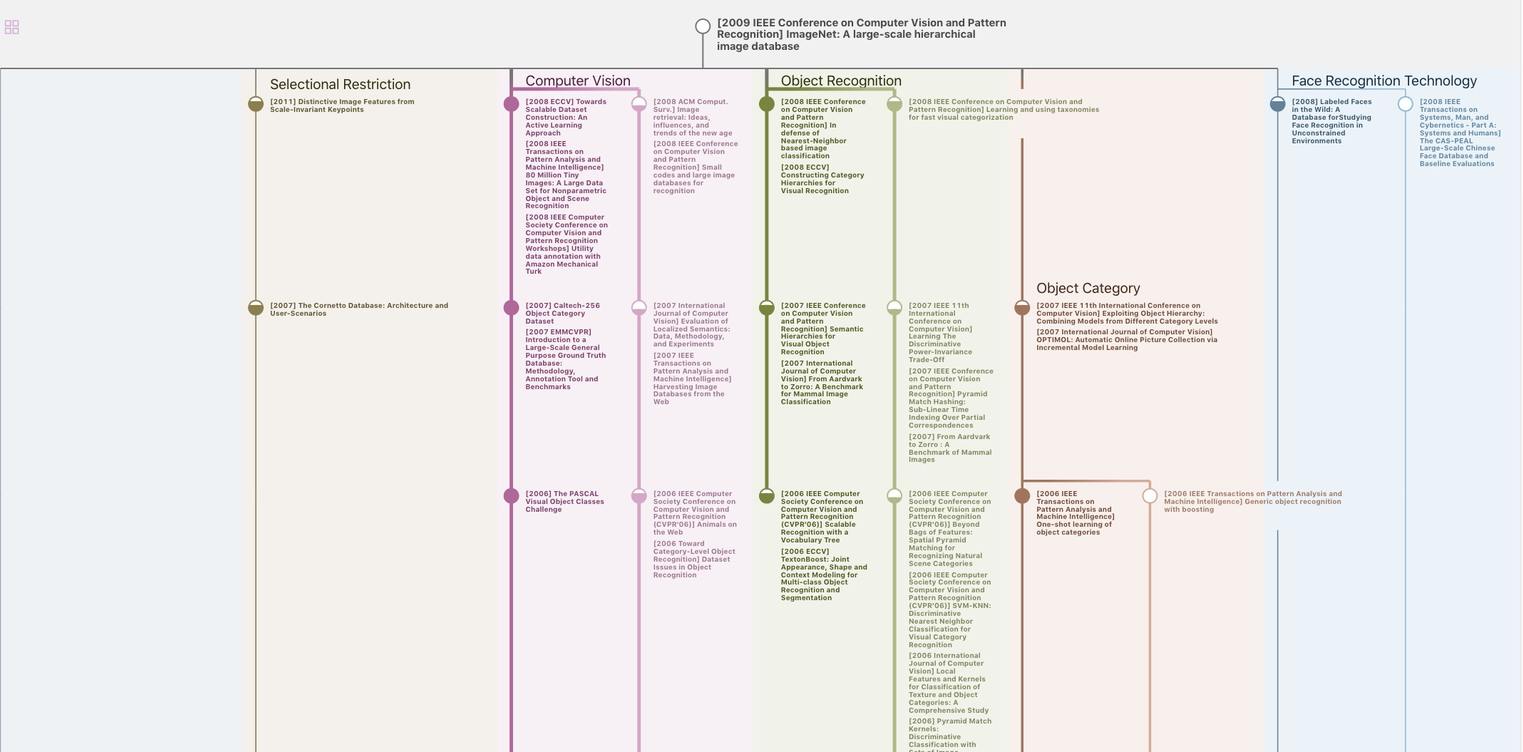
生成溯源树,研究论文发展脉络
Chat Paper
正在生成论文摘要