From Mobility Data To Habits And Common Pathways
EXPERT SYSTEMS(2020)
摘要
Many aspects of our lives are associated with places and the activities we perform on a daily basis. Most of them are recurrent and demand displacement of the individual between regular places like going to work, school or other important personal locations. To accomplish these recurrent daily activities, people tend to follow regular paths with similar temporal and spatial characteristics, especially because humans are frequently looking for uniformity to support their decisions and make their actions easier or even automatic. In this work, we propose a method for discovering common pathways across users' habits from human mobility data. By using a density-based clustering algorithm, we identify the most preferable locations the users visit, we apply a Gaussian mixture model over these places to automatically separate among all traces, the trajectories that follow patterns in order to discover the representations of individual's habits. By using the longest common sub-sequence algorithm, we search for the trajectories that are more similar over the set of users' habits trips by considering the distance that pairs of users or habits share on the same path. The proposed method is evaluated over two real-world GPS datasets and the results show that the approach is able to detect the most important places in a user's life, detect the routine activities and identify common routes between users that have similar habits paving the way for research techniques in carpooling, recommendation and prediction systems.
更多查看译文
关键词
Gaussian mixture model, habits, longest common sub-sequence, mobility, pattern, spatio-temporal clustering
AI 理解论文
溯源树
样例
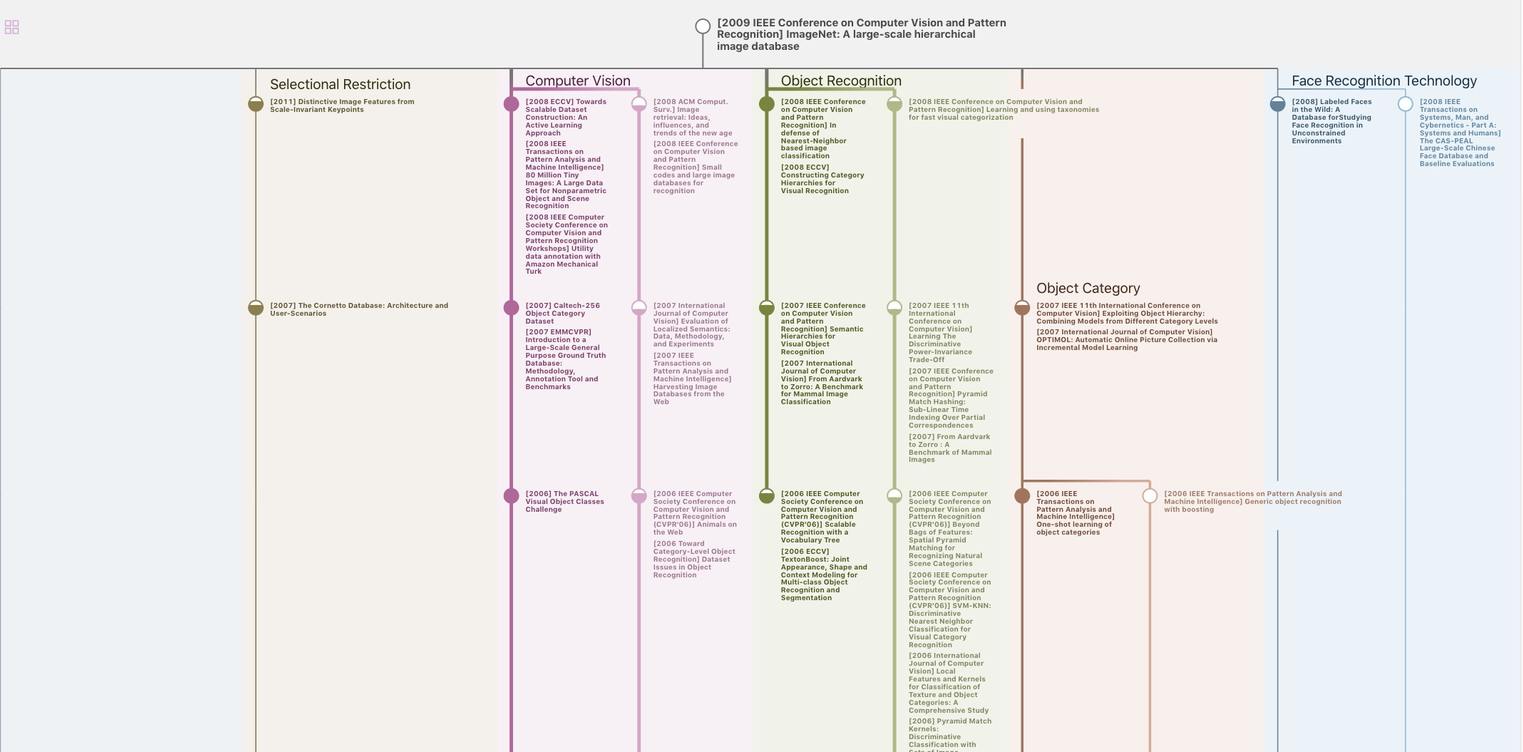
生成溯源树,研究论文发展脉络
Chat Paper
正在生成论文摘要