One-Dimensional Convolutional Neural Networks For High-Resolution Range Profile Recognition Via Adaptively Feature Recalibrating And Automatically Channel Pruning
INTERNATIONAL JOURNAL OF INTELLIGENT SYSTEMS(2021)
摘要
High-resolution range profile (HRRP) has obtained intensive attention in radar target recognition and convolutional neural networks (CNNs) are among predominant approaches to deal with HRRP recognition problems. However, most CNNs are designed by the rule-of-thumb and suffer from much more computational complexity. Aiming at enhancing the channels of one-dimensional CNN (1D-CNN) for extracting efficient structural information oftargets form HRRP and reducing the computation complexity, we propose a novel framework for HRRP-based target recognition based on 1D-CNN with channel attention and channel pruning. By introducing an aggregation-perception-recalibration (APR) block for channel attention to the 1D-CNN backbone, channels in each 1D convolutional layer can adaptively learn to recalibrate the extracted features for enhancing the structural information captured from HRRP. To avoid rule-of-thumb design and reduce the computation complexity of 1D-CNN, we proposed a new method incorporated withthe global best leading artificial bee colony (GBL-ABC) to prune the original network based on the lottery ticket hypothesis in an automatic and heuristic manner. The extensive experimental results on the measured data illustrate that the proposed algorithm achievesthe superiorrecognition rate by combing APR and GBL-ABC simultaneously.
更多查看译文
关键词
channel attention, channel pruning, convolution neural networks, global best leading artificial bee colony, high-resolution range profile
AI 理解论文
溯源树
样例
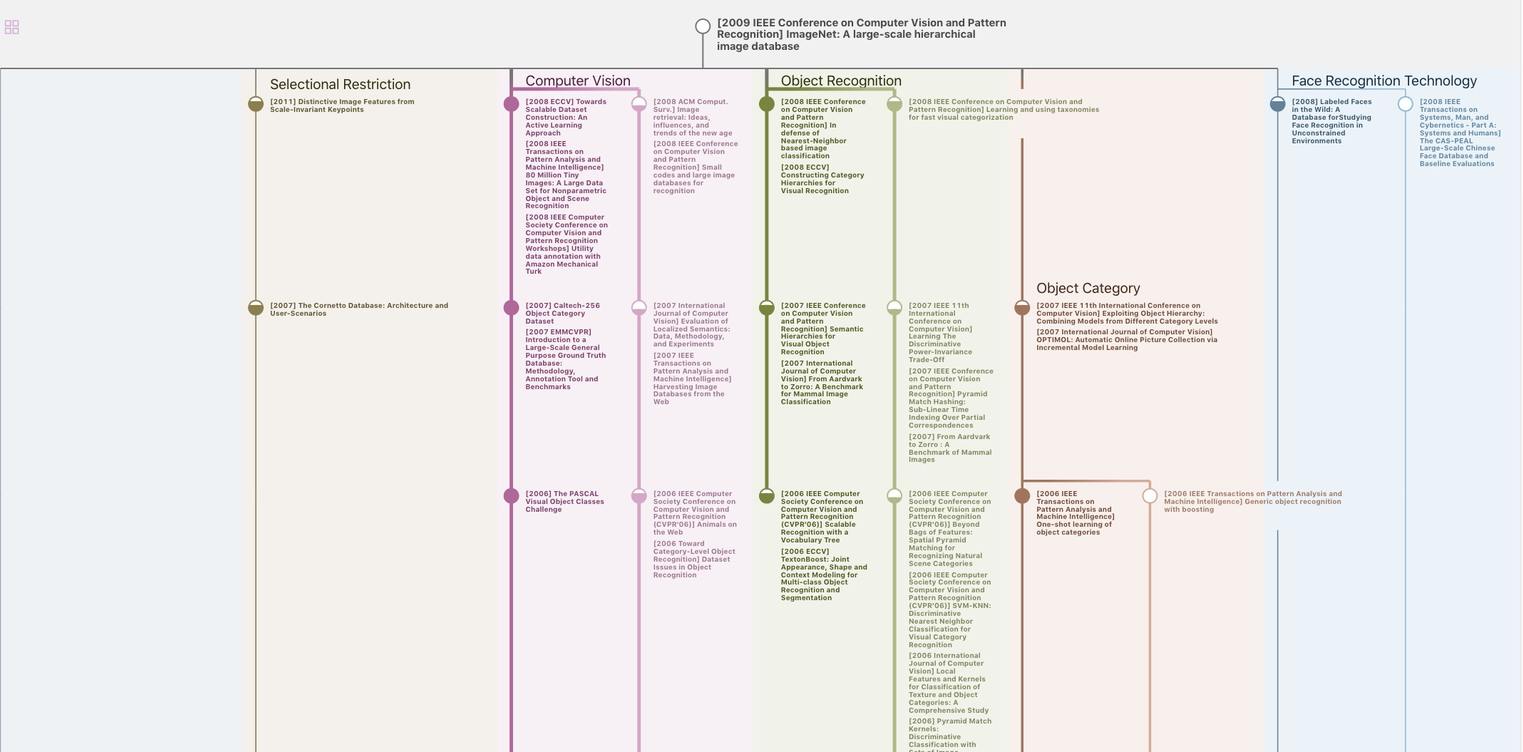
生成溯源树,研究论文发展脉络
Chat Paper
正在生成论文摘要