Performance limits of one-bit compressive classification.
SIGNAL PROCESSING(2021)
摘要
Classification is an important task in the fields of signal processing and machine learning. Recently, compressive classification (CC) appears to enable signal classification directly with the compressive measurements, which are acquired by the technique of compressive sensing (CS). However, the existing works of CC ignore the practical quantization operation. This paper studies the one-bit CC based on one-bit quantized compressive measurements, which is appealing in saving the transmission and storage bits in many applications when the bandwidth and energy are constrained. For the signals that are not necessarily sparse as well as exactly sparse, we provide the performance limits of one-bit CC. We first analyze the Chernoff distance and Kullback-Leibler (KL) distance between two probability density functions under any two hypotheses. The results are then used to derive the upper and lower bounds on the probability of misclassification in the high-dimensional setting. The analytical results show that one-bit quantized CC has the same performance trend as conventional not-quantized CC when the number of measurements increases. (C) 2020 Elsevier B.V. All rights reserved.
更多查看译文
关键词
Compressive sensing (CS),Compressive classification (CC),One-bit CS,One-bit CC,Performance limits
AI 理解论文
溯源树
样例
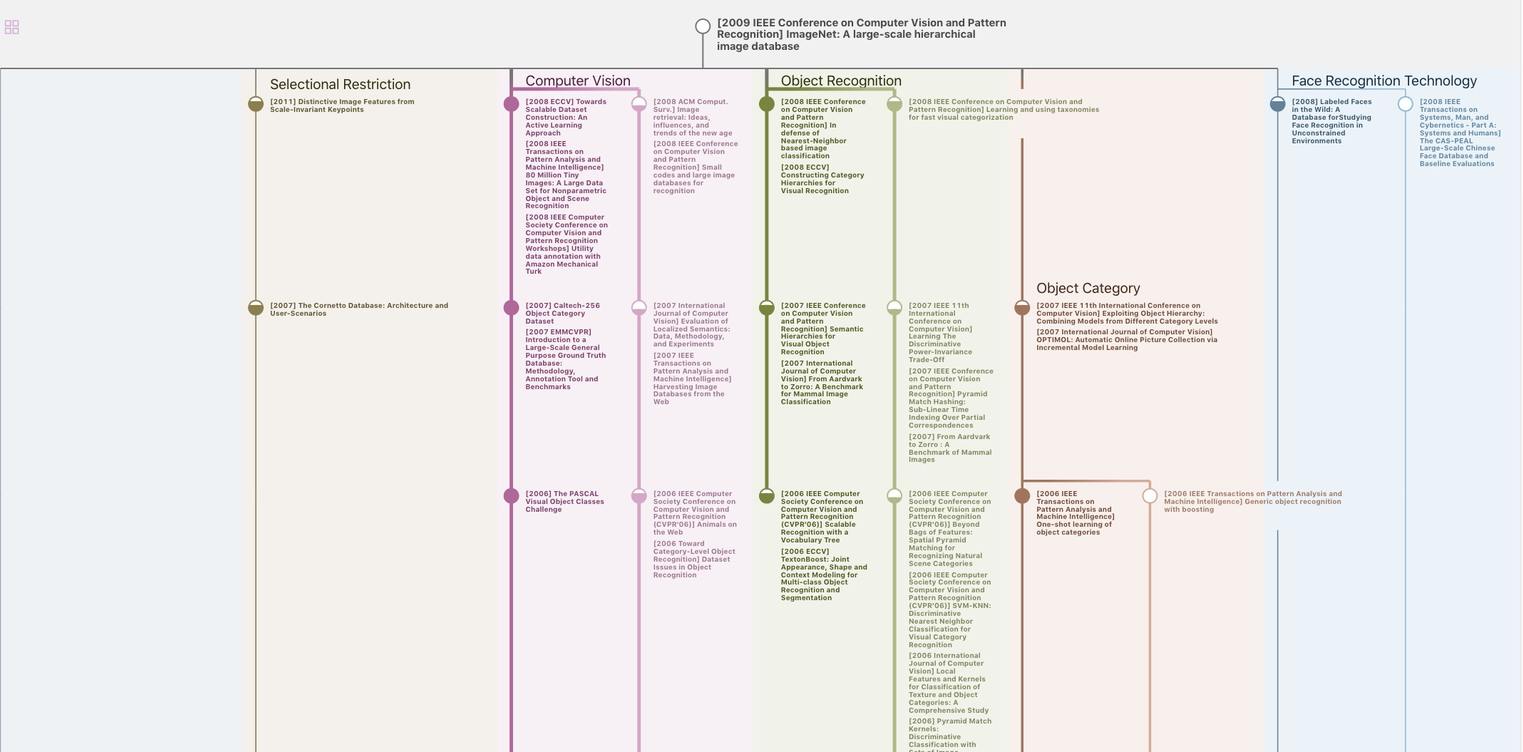
生成溯源树,研究论文发展脉络
Chat Paper
正在生成论文摘要