Chinese Agricultural Diseases And Pests Named Entity Recognition With Multi-Scale Local Context Features And Self-Attention Mechanism
COMPUTERS AND ELECTRONICS IN AGRICULTURE(2020)
摘要
Chinese named entity recognition is a crucial initial step of information extraction in the field of agricultural diseases and pests. This step aims to identify named entities related to agricultural diseases and pests from unstructured texts but presents challenges. The available corpus in this domain is limited, and most existing named entity recognition methods only focus on the global context information but neglect potential local context features, which are also equally important for named entity recognition. To solve the above problems and tackle the named entity recognition task in this paper, an available corpus toward agricultural diseases and pests, namely AgCNER, which contains 11 categories and 34,952 samples, was established. Compared with the corpora in the same field, this corpus has additional categories and more sample sizes. Then, a novel Chinese named entity recognition model via joint multi-scale local context features and the self-attention mechanism was proposed. The original Bi-directional Long Short-Term Memory and Conditional Random Field model (BiLSTM-CRF) was improved by fusing the multi-scale local context features extracted by Convolutional Neural Network (CNN) with different kernel sizes. The self-attention mechanism was also used to break the limitation of BiLSTM-CRF in capturing long-distance dependencies and further improve the model performance. The performance of the proposed model was evaluated on three corpora, namely AgCNER, Resume, and MSRA, which achieved the optimal F1-values of 94.15%, 94.56%, and 90.55%, respectively. Experimental results in many aspects illustrated the effective performance of the proposed model in this paper.
更多查看译文
关键词
Chinese agricultural diseases and pests named entity recognition, Corpus, Multi-scale local context features, Convolutional neural networks, Self-attention mechanism
AI 理解论文
溯源树
样例
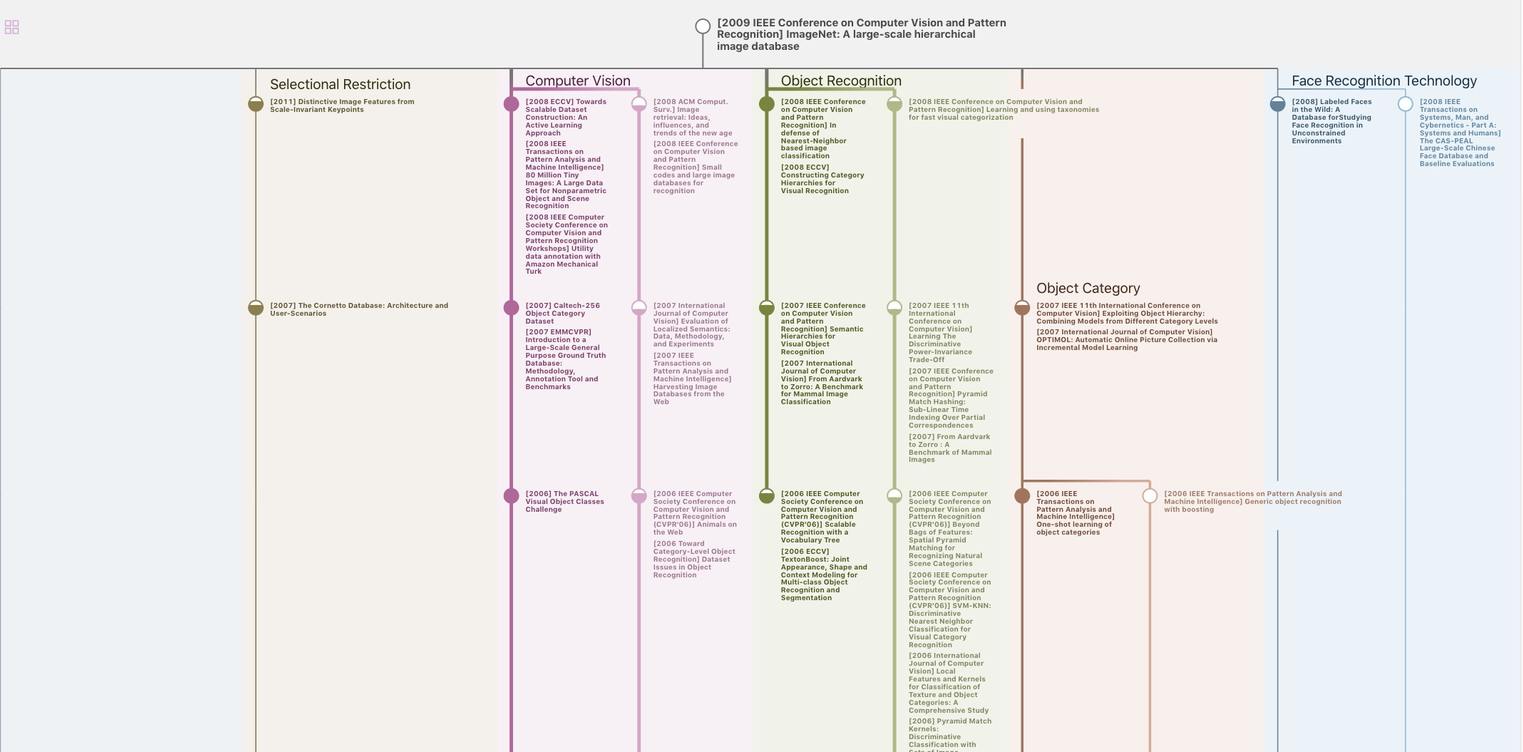
生成溯源树,研究论文发展脉络
Chat Paper
正在生成论文摘要