Nonconvex Rectangular Matrix Completion via Gradient Descent Without ℓ ₂,∞ Regularization
IEEE Transactions on Information Theory(2020)
摘要
The analysis of nonconvex matrix completion has recently attracted much attention in the community of machine learning thanks to its computational convenience. Existing analysis on this problem, however, usually relies on ℓ
2
,
∞
projection or regularization that involves unknown model parameters, although they are observed to be unnecessary in numerical simulations. In this paper, we extend the analysis of the vanilla gradient descent for positive semidefinite matrix completion in the literature to the rectangular case, and more significantly, improve the required sampling rate from O(poly(κ)μ
3
r
3
log
3
n/n) to O(μ
2
r
2
κ
14
log n/n). Our technical ideas and contributions are potentially useful in improving the leave-one-out analysis in other related problems.
更多查看译文
关键词
Matrix completion,nonconvex optimization
AI 理解论文
溯源树
样例
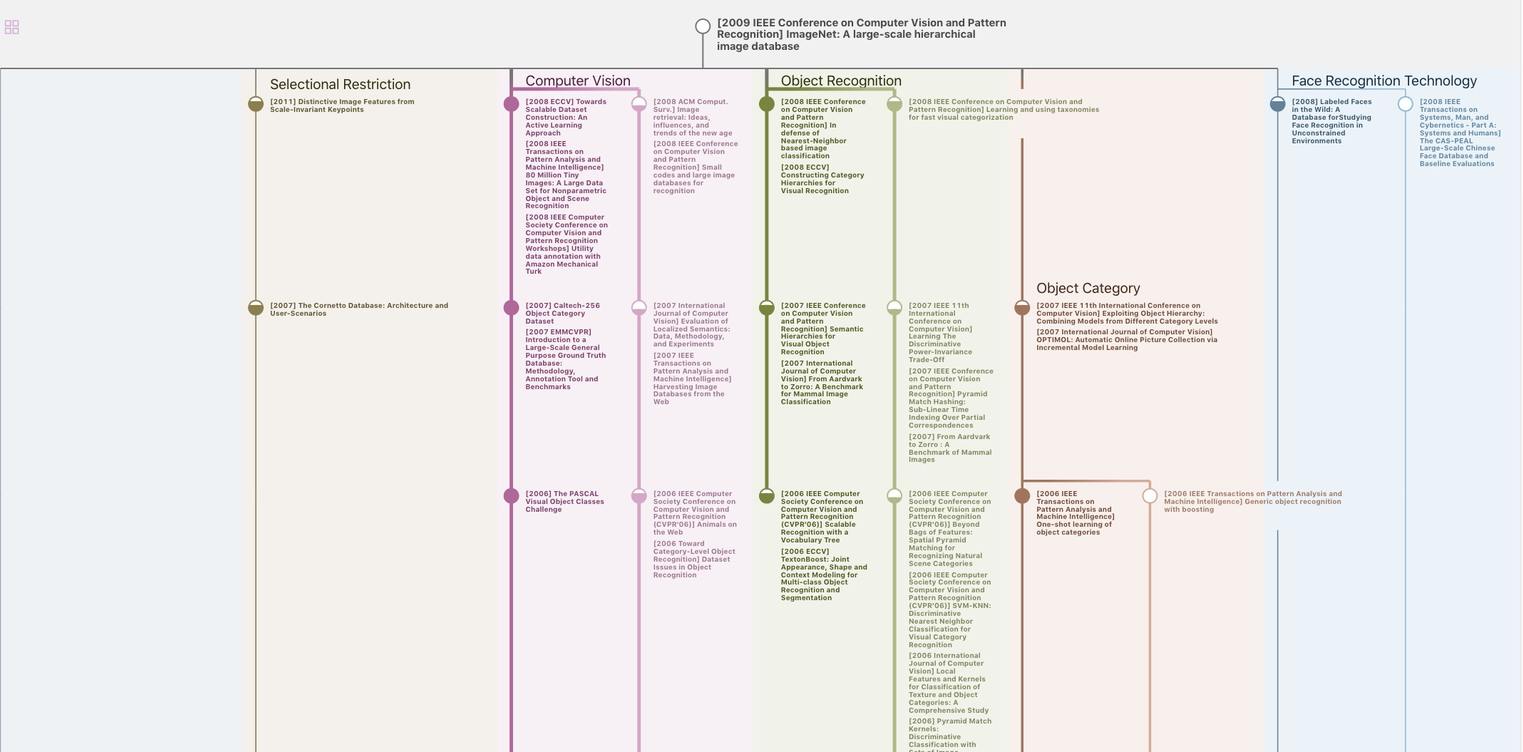
生成溯源树,研究论文发展脉络
Chat Paper
正在生成论文摘要