Automatic Extraction and Filtering of OpenStreetMap Data to Generate Training Datasets for Land Use Land Cover Classification.
REMOTE SENSING(2020)
摘要
This paper tests an automated methodology for generating training data from OpenStreetMap (OSM) to classify Sentinel-2 imagery into Land Use/Land Cover (LULC) classes. Different sets of training data were generated and used as inputs for the image classification. Firstly, OSM data was converted into LULC maps using the OSM2LULC_4T software package. The Random Forest classifier was then trained to classify a time-series of Sentinel-2 imagery into 8 LULC classes with samples extracted from: (1) The LULC maps produced by OSM2LULC_4T (TD0); (2) the TD1 dataset, obtained after removing mixed pixels from TD0; (3) the TD2 dataset, obtained by filtering TD1 using radiometric indices. The classification results were generalized using a majority filter and hybrid maps were created by merging the classification results with the OSM2LULC outputs. The accuracy of all generated maps was assessed using the 2018 official "Carta de Ocupacao do Solo" (COS). The methodology was applied to two study areas with different characteristics. The results show that in some cases the filtering procedures improve the training data and the classification results. This automated methodology allowed the production of maps with overall accuracy between 55% and 78% greater than that of COS, even though the used nomenclature includes classes that can be easily confused by the classifiers.
更多查看译文
关键词
land use land cover,training data,OpenStreetMap,Sentinel-2,COS (Carta de Ocupaç,ã,o do Solo),volunteered geographical information (VGI)
AI 理解论文
溯源树
样例
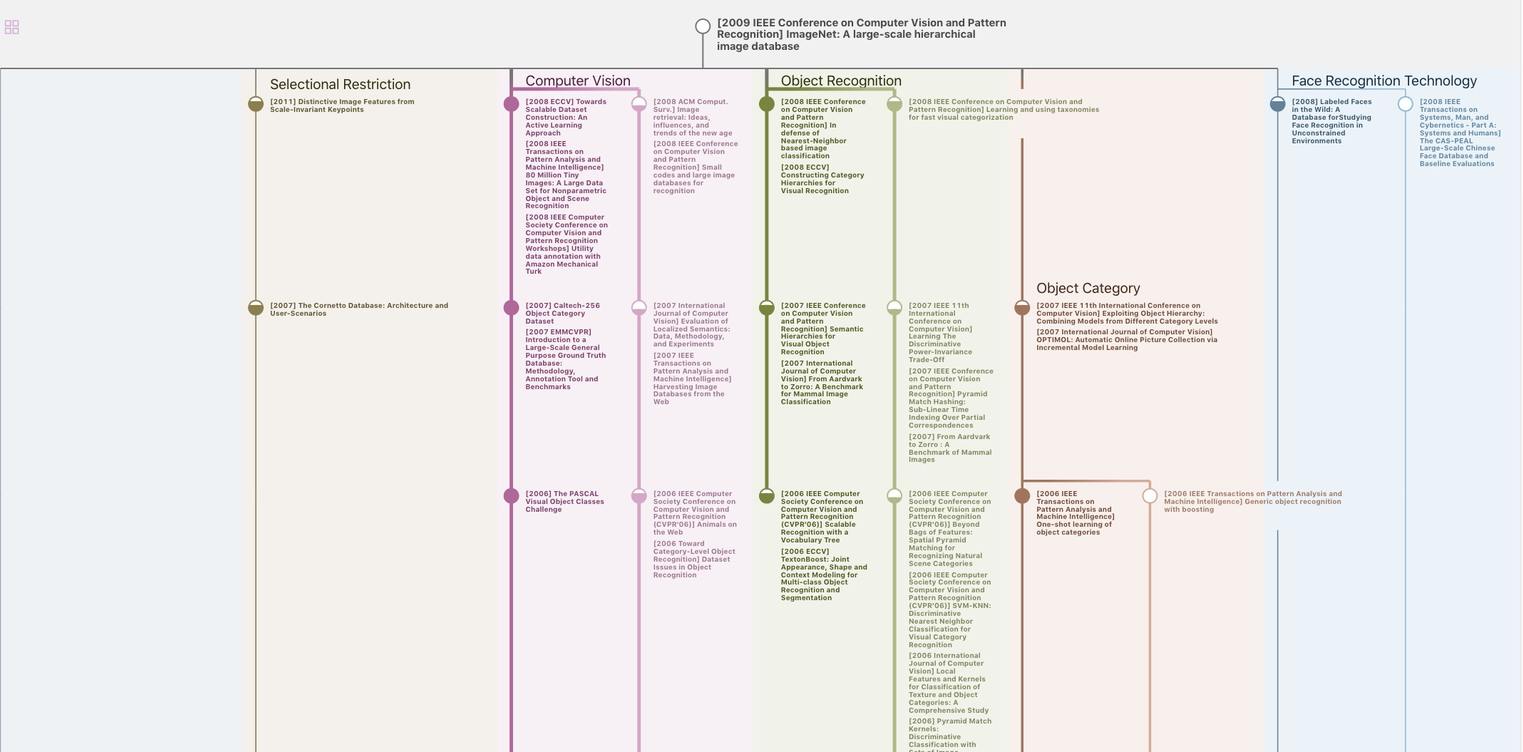
生成溯源树,研究论文发展脉络
Chat Paper
正在生成论文摘要