Multispectral LiDAR-Based Estimation of Surface Fuel Load in a Dense Coniferous Forest.
REMOTE SENSING(2020)
摘要
Surface fuel load (SFL) constitutes one of the most significant fuel components and is used as an input variable in most fire behavior prediction systems. The aim of the present study was to investigate the potential of discrete-return multispectral Light Detection and Ranging (LiDAR) data to reliably predict SFL in a coniferous forest characterized by dense overstory and complex terrain. In particular, a linear regression analysis workflow was employed with the separate and combined use of LiDAR-derived structural and pulse intensity information for the load estimation of the total surface fuels and individual surface fuel types. Following a leave-one-out cross-validation (LOOCV) approach, the models developed from the different sets of predictor variables were compared in terms of their estimation accuracy. LOOCV indicated that the predictive models produced by the combined use of structural and intensity metrics significantly outperformed the models constructed with the individual sets of metrics, exhibiting an explained variance (R-2) between 0.59 and 0.71 (relative Root Mean Square Error (RMSE) 19.3-37.6%). Overall, the results of this research showcase that both structural and intensity variables provided by multispectral LiDAR data are significant for surface fuel load estimation and can successfully contribute to effective pre-fire management, including fire risk assessment and behavior prediction in case of a fire event.
更多查看译文
关键词
surface fuel load,remote sensing,airborne LiDAR,multiple regression
AI 理解论文
溯源树
样例
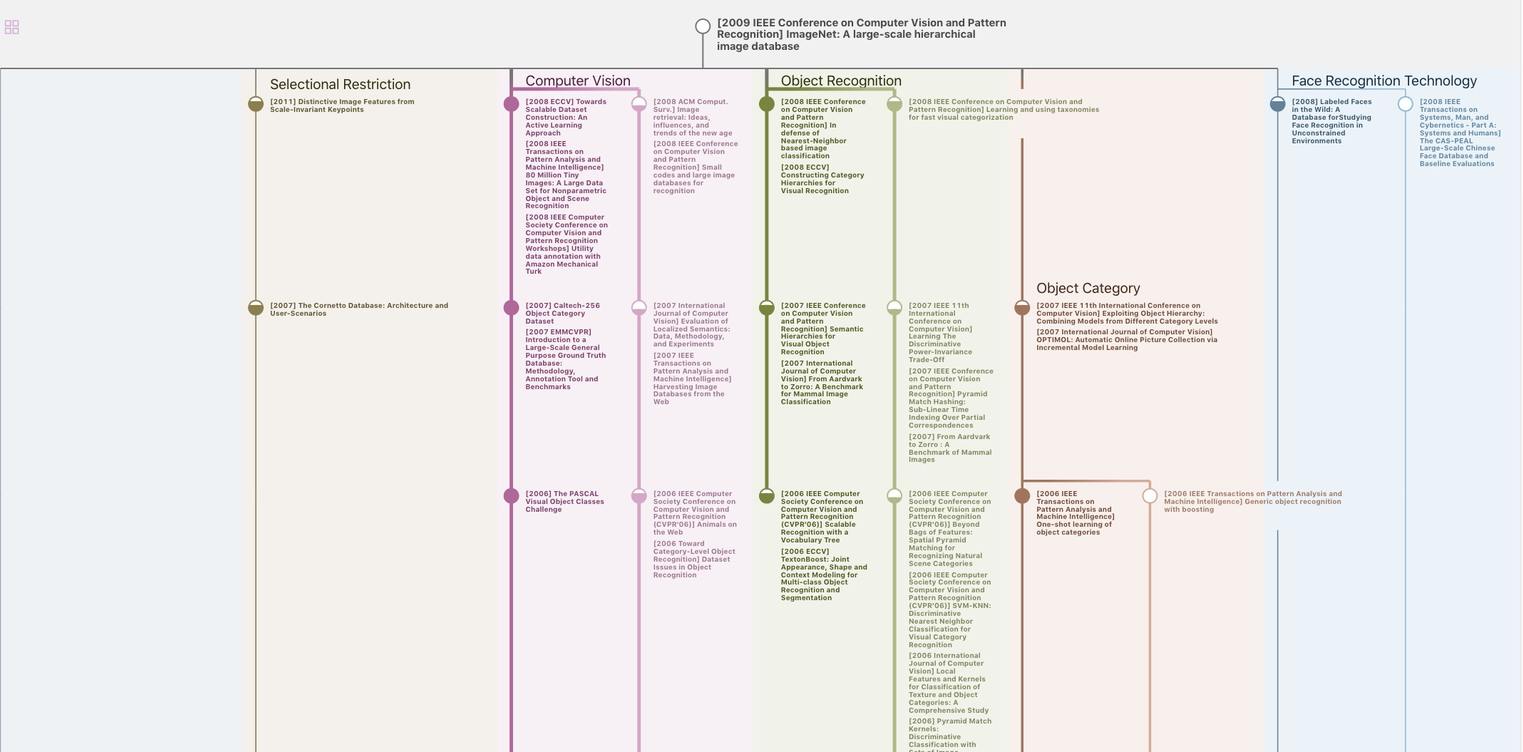
生成溯源树,研究论文发展脉络
Chat Paper
正在生成论文摘要