A Novel Deep Forest-Based Active Transfer Learning Method for PolSAR Images.
REMOTE SENSING(2020)
摘要
The information extraction of polarimetric synthetic aperture radar (PolSAR) images typically requires a great number of training samples; however, the training samples from historical images are less reusable due to the distribution differences. Consequently, there is a significant manual cost to collecting training samples when processing new images. In this paper, to address this problem, we propose a novel active transfer learning method, which combines active learning and the deep forest model to perform transfer learning. The main idea of the proposed method is to gradually improve the performance of the model in target domain tasks with the increase of the levels of the cascade structure. More specifically, in the growing stage, a new active learning strategy is used to iteratively add the most informative target domain samples to the training set, and the augmented features generated by the representation learning capability of the deep forest model are used to improve the cross-domain representational capabilities of the feature space. In the filtering stage, an effective stopping criterion is used to adaptively control the complexity of the model, and two filtering strategies are used to accelerate the convergence of the model. We conducted experiments using three sets of PolSAR images, and the results were compared with those of four existing transfer learning algorithms. Overall, the experimental results fully demonstrated the effectiveness and robustness of the proposed method.
更多查看译文
关键词
PolSAR,reusability of training samples,transfer learning,active learning,deep forest
AI 理解论文
溯源树
样例
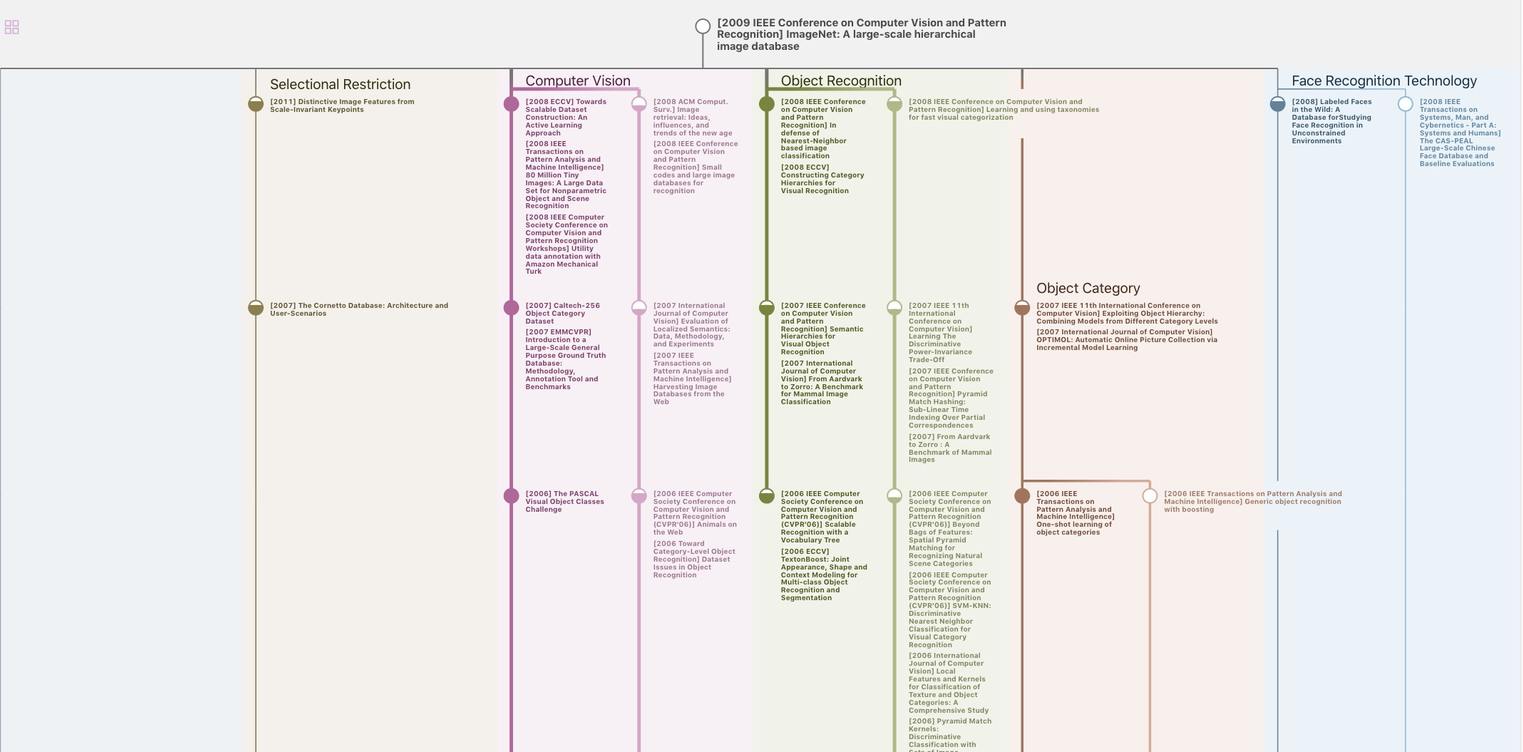
生成溯源树,研究论文发展脉络
Chat Paper
正在生成论文摘要