Hypergraph-based image search reranking with elastic net regularized regression
MULTIMEDIA TOOLS AND APPLICATIONS(2020)
摘要
Image search reranking is emerging as an effective technique to refine the text-based image search results using visual information. In this paper, we introduce a novel hypergraph-based image search reranking method that accounts for both relevance and diversity of search results. Namely, the text-based image search results are taken as vertices in a probabilistic regression-based hypergraph model and reranking is formulated as a hypergraph ranking problem with absorbing nodes. More specifically, to discover related samples and characterize the relationships among them, we bring the Elastic Net regularized regression model into the hypergraph construction. Exceeding the conventional hypergraph construction schemes, our scheme is able to describe the high-order relationships and the local manifold structure among visual samples while ensuring the datum-adaptiveness. Afterward, we apply a hypergraph-based ranking with absorbing nodes to ensure a diversified reranking. That is, during the reranking process, previously-ranked samples are transformed into absorbing nodes at each iteration, thereby redundant ones are prevented from receiving high ranking scores. Extensive experiments on real-world data from Flickr suggest our proposed reranking method achieves promising results compared to existing reranking methods.
更多查看译文
关键词
Image search,Visual reranking,Hypergraph,Elastic net,Regularized regression
AI 理解论文
溯源树
样例
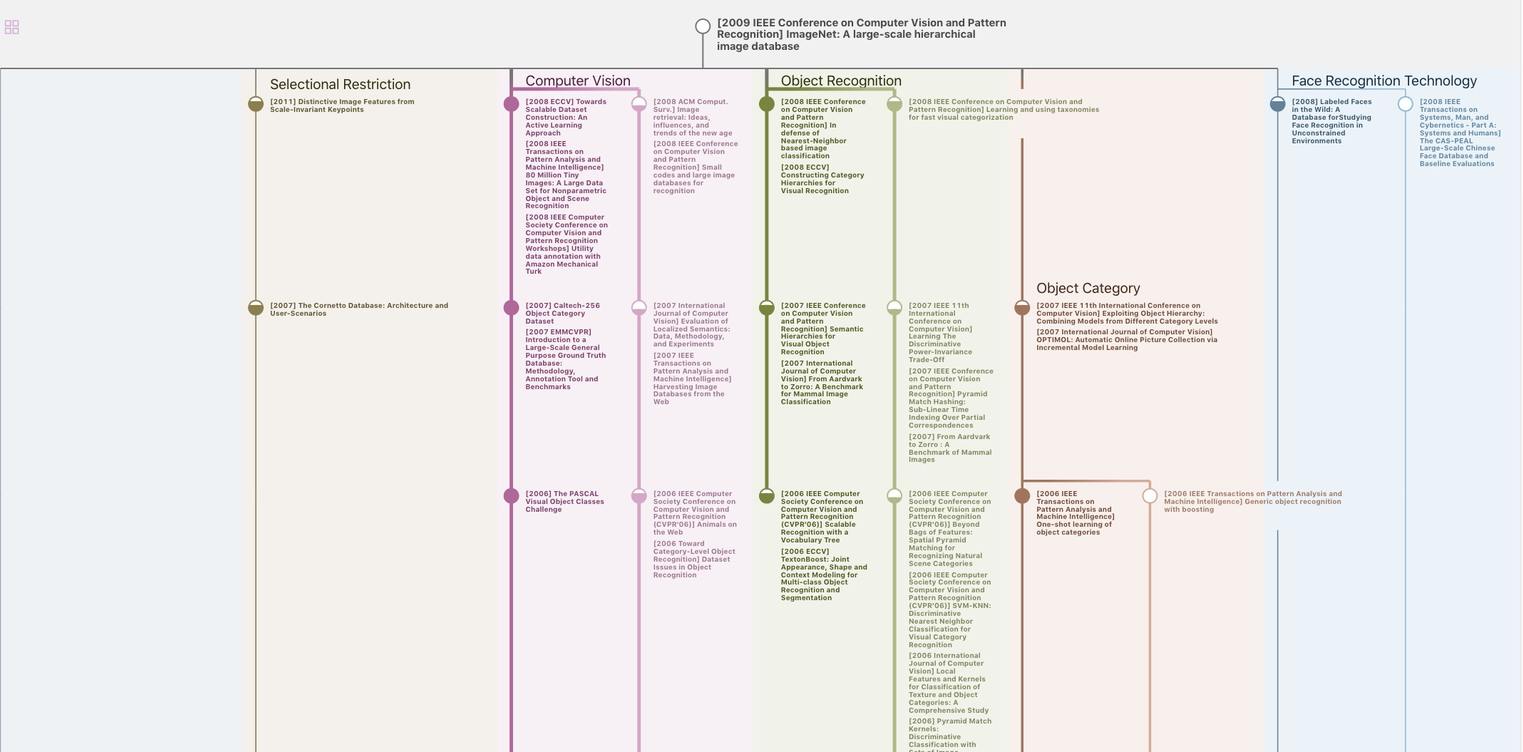
生成溯源树,研究论文发展脉络
Chat Paper
正在生成论文摘要