Design and analysis of a synthetic prediction market using dynamic convex sets
arxiv(2021)
摘要
We present a synthetic prediction market whose agent purchase logic is defined using a sigmoid transformation of a convex semi-algebraic set defined in feature space. Asset prices are determined by a logarithmic scoring market rule. Time varying asset prices affect the structure of the semi-algebraic sets leading to time-varying agent purchase rules. We show that under certain assumptions on the underlying geometry, the resulting synthetic prediction market can be used to arbitrarily closely approximate a binary function defined on a set of input data. We also provide sufficient conditions for market convergence and show that under certain instances markets can exhibit limit cycles in asset spot price. We provide an evolutionary algorithm for training agent parameters to allow a market to model the distribution of a given data set and illustrate the market approximation using three open source data sets. Results are compared to standard machine learning methods.
更多查看译文
关键词
Prediction market,Machine learning,Dynamical systems
AI 理解论文
溯源树
样例
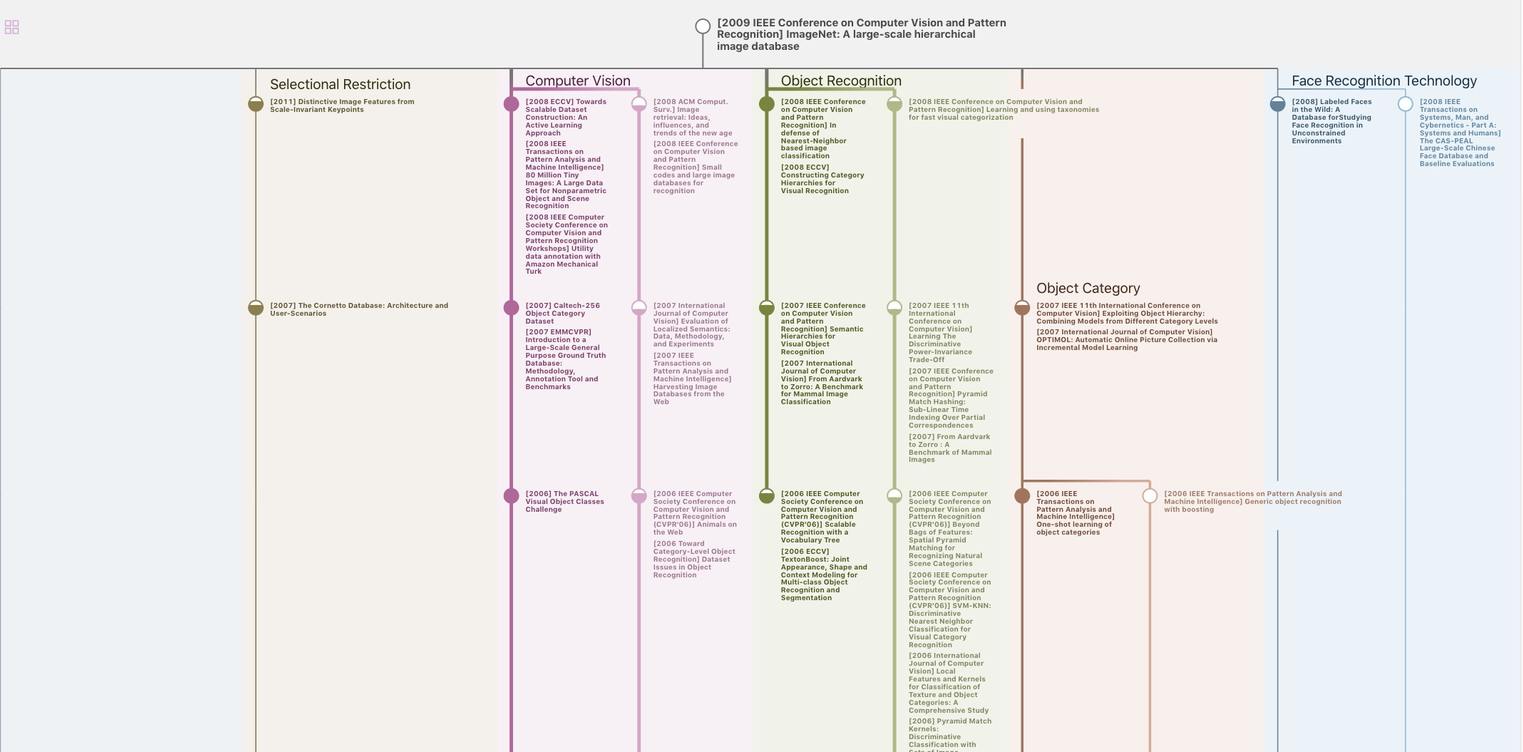
生成溯源树,研究论文发展脉络
Chat Paper
正在生成论文摘要