Providing a Hybrid Approach for Detecting Malicious Traffic on the Computer Networks Using Convolutional Neural Networks
2020 28TH IRANIAN CONFERENCE ON ELECTRICAL ENGINEERING (ICEE)(2020)
摘要
With the growth of the Internet, computer networks have become an important tool for communication between human societies. Nowadays, all the activities of the people, especially financial, medical and military activities are carried out over the Internet and that has made cyber attacks a significant improvement. Hackers' motivation has also shifted to network-based activities. Therefore, one of the major challenges is detecting and preventing network-based cyber attacks. Given the remarkable ability of deep learning algorithms, the purpose of this study is to present a hybrid approach using Convolutional Neural Network (CNN) and Long Short Term Memory networks (LSTM) to improve the performance of Intrusion Detection Systems (IDS). In previous studies, whilst discriminating between normal and abnormal traffic has been achieved reasonable accuracy the precision of multi-class classification was not optimal. The aim of this study is to provide a method to accurately classify malicious traffics according to attack types. In this study, the results are validated on NSL-KDD and CICIDS2017 datasets. Multiple classification accuracy for the NSL-KDD and CICIDS2017 datasets are 98.1 and 96.7, respectively.
更多查看译文
关键词
Convolutional Neural Networks, Deep Learning, Intrusion Detection Systems, Long Short Term Memory
AI 理解论文
溯源树
样例
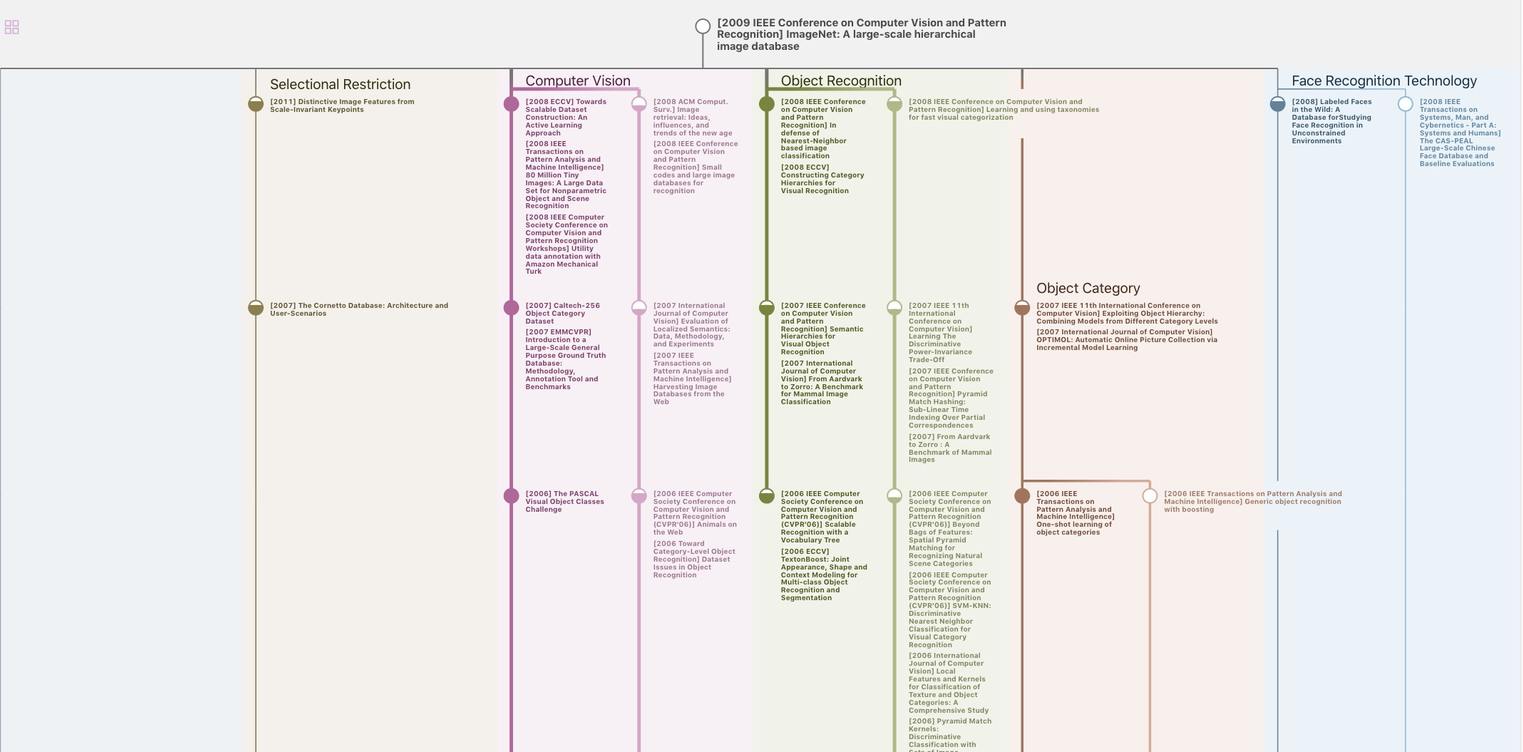
生成溯源树,研究论文发展脉络
Chat Paper
正在生成论文摘要