An Exploratory Analysis Of Data Noisy Scenarios In A Pareto-Front Based Dynamic Feature Selection Method
APPLIED SOFT COMPUTING(2021)
摘要
Feature selection has become a mandatory step in several data exploration and Machine Learning applications since data quality can have a strong impact in the performance of machine learning models. Many feature selection strategies have been developed in the past decades, using different criteria to select the most relevant features. The use of dynamic feature selection, however, has showed that the use of multiple simultaneously criteria to determine the best attribute subset for similar instances can deliver encouraging results. In this context, this paper proposes to analyze the performance of a pareto-front based dynamic feature selection (PF-DFS) method under data noise scenarios. In order to do this, we intentionally added noise in 15 datasets and evaluated the PF-DFS performance in order to measure its stability under two different data noise scenarios. The obtained results are compared to some state-of-the-art algorithms and show that, in terms of accuracy, the PF-DFS method is more robust to the other methods for the majority of the analyzed scenarios.
更多查看译文
关键词
Feature selection, Clustering algorithms, Pareto-front selection, Noisy data
AI 理解论文
溯源树
样例
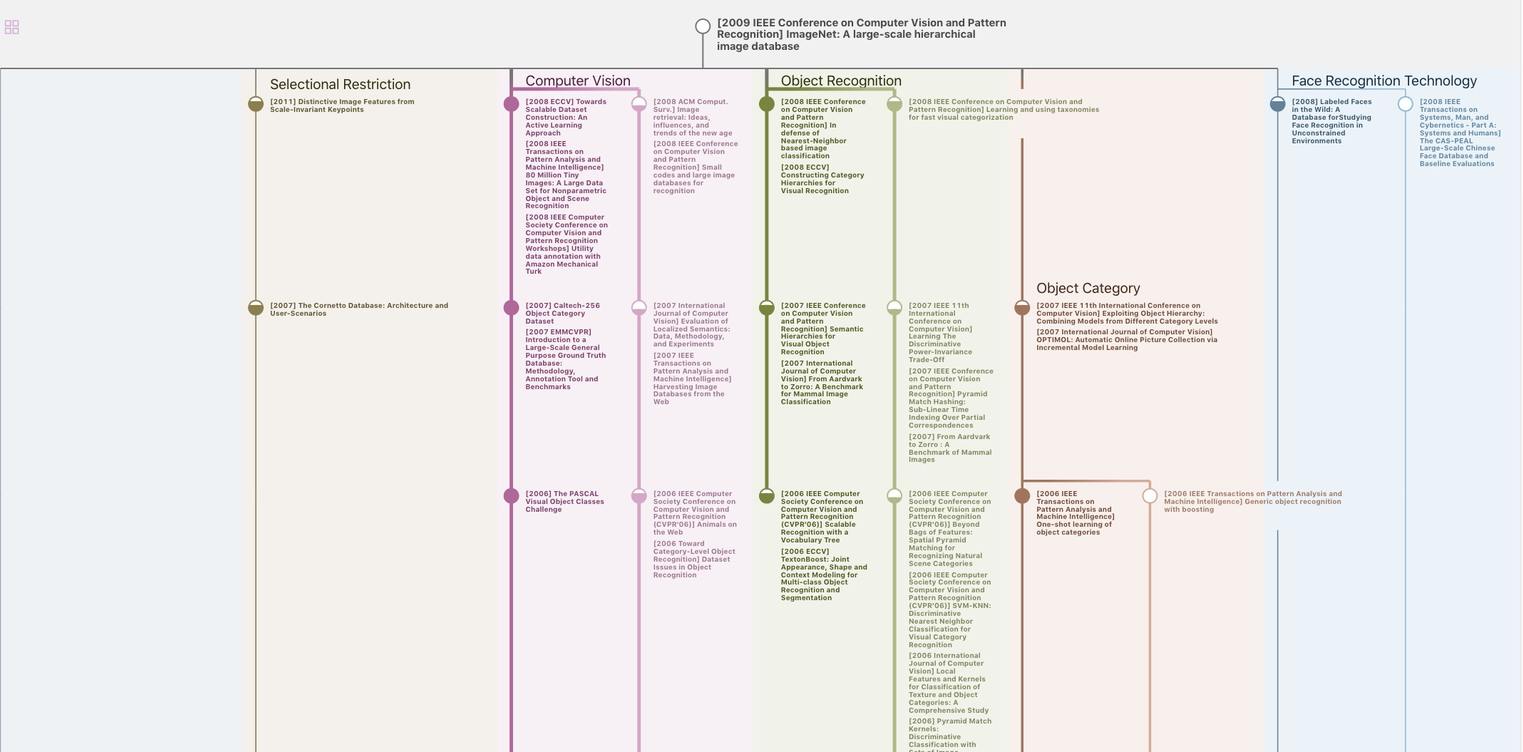
生成溯源树,研究论文发展脉络
Chat Paper
正在生成论文摘要