An Effective Semi-Supervised Multi-Label Least Squares Twin Support Vector Machine
IEEE ACCESS(2020)
摘要
Multi-label twin support vector machine (MLTSVM), being an effective multi-label classifier based on twin support vector machine (TSVM), has been widely studied and applied due to its excellent classification performance. However, there are some disadvantages in classical MLTSVM: (a) MLTSVM needs to solve a series of quadratic programming problems (QPPs), which makes its learning speed lower. (b) For multi-label learning problems, it is very difficult to obtain all labels of all samples. In fact, the datasets that we can obtain only contain a small amount of labeled samples and a large amount of partially labeled and unlabeled samples. However, MLTSVM can only use expensive labeled samples and ignore cheap unlabeled and partially labeled samples. For the drawbacks, we propose a novel semi-supervised multi-label least squares twin support vector machine, called SS-MLLSTSVM. Firstly, to speed up solving, SS-MLLSTSVM introduces the least squares idea into each sub-classifier of MLTSVM, which makes each sub-classifier only need to solve a system of linear equations, instead of one QPP. Secondly, SS-MLLSTSVM can make full use of the geometric information in unlabeled and partially labeled samples by introducing manifold regularization term into each sub-classifier. The experimental results on the benchmark datasets show that, compared with the existing multi-label classification algorithms, our SS-MLLSTSVM has better classification performance.
更多查看译文
关键词
Training, Support vector machines, Manifolds, Quadratic programming, Licenses, Benchmark testing, Semisupervised learning, Twin support vector machine, least squares, multi-label learning, semi-supervised learning
AI 理解论文
溯源树
样例
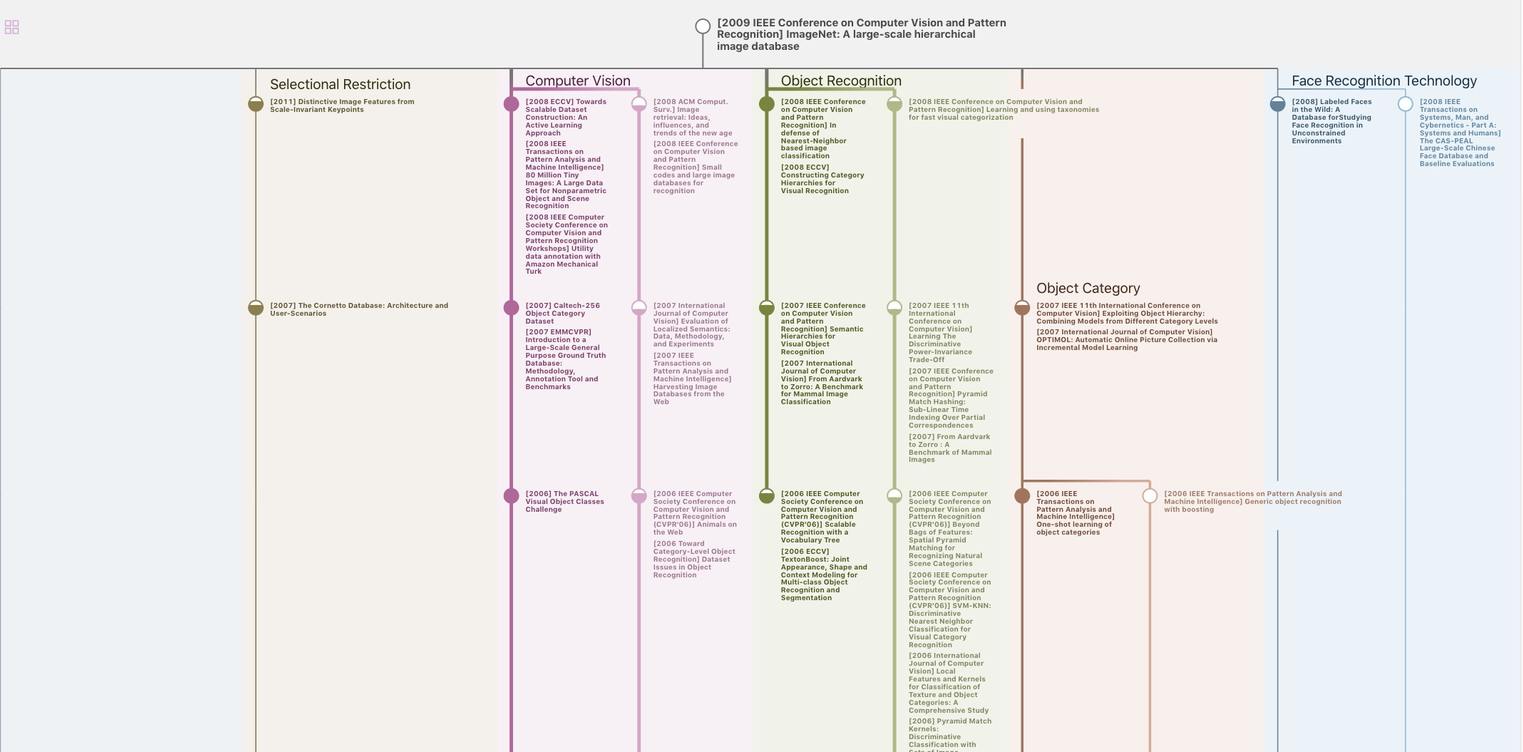
生成溯源树,研究论文发展脉络
Chat Paper
正在生成论文摘要