Robust SAR Automatic Target Recognition Via Adversarial Learning
IEEE Journal of Selected Topics in Applied Earth Observations and Remote Sensing(2021)
摘要
The traditional denoising methods in noise robust synthetic aperture radar (SAR) automatic target recognition research are independent of the recognition model, which limits the robust recognition performance. In this article, we present a robust SAR automatic target recognition method via adversarial learning, which could integrate data denoising, feature extraction, and classification into a unified framework for joint learning. Different from the common recognition methods of directly inputting the SAR data into the classifiers, we add a dual-generative-adversarial-network (GAN) model between the SAR data and the classifier for data translation from a noise-polluted style to a relatively clean style to reduce the noise from SAR data. In order to ensure the target information in the SAR data can be retained during the data style translation, reconstruction constraint and label constraint are also used in the dual-GAN model. Then, the more reliable transferred SAR data are fed into the classifier. The parameters of the dual-GAN and classifier are learned through joint optimization in our method. Thus, the data separability is guaranteed in the process of denoising and feature extraction, which greatly improves the recognition performance of the method. In addition, our method can be easily extended to a semisupervised method by using different objective functions for labeled and unlabeled training data, which is more suitable for practical application. Experimental results on MSTAR dataset and Gotcha dataset show that our method can get the encouraging performance in the case of low signal-to-noise ratio and small labeled data size.
更多查看译文
关键词
Adversarial learning,automatic target recognition (ATR),generative adversarial networks (GAN),noise robust,semisupervised learning,synthetic aperture radar (SAR)
AI 理解论文
溯源树
样例
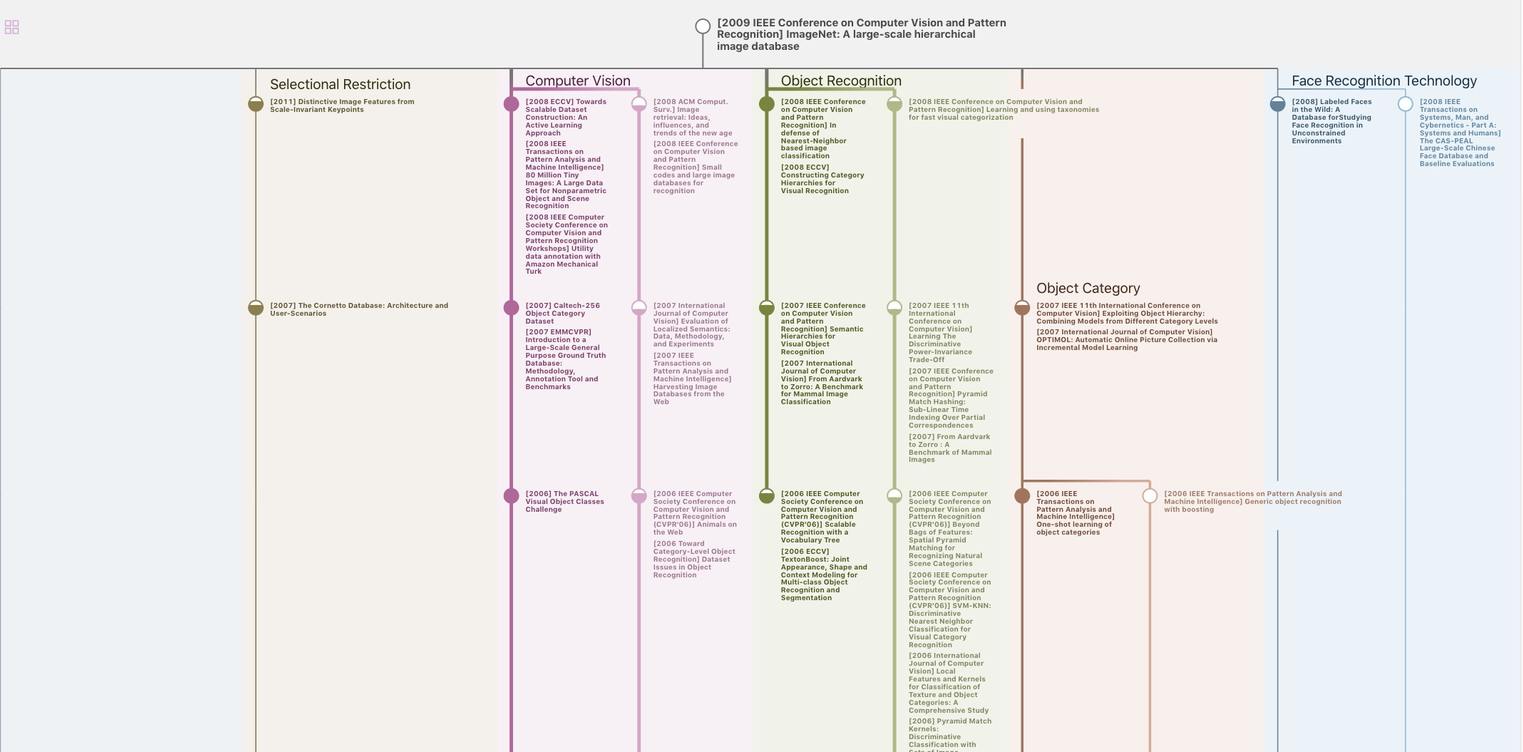
生成溯源树,研究论文发展脉络
Chat Paper
正在生成论文摘要