A class of smooth exact penalty function methods for optimization problems with orthogonality constraints
Optimization Methods & Software(2020)
摘要
Updating the augmented Lagrangian multiplier by closed-form expression yields efficient first-order infeasible approach for optimization problems with orthogonality constraints. Hence, parallelization becomes tractable in solving this type of problems. Inspired by this closed-form updating scheme, we propose a novel penalty function with compact convex constraints (PenC). We show that PenC can act as an exact penalty model which shares the same global minimizers as the original problem with orthogonality constraints. Based on PenC, we first propose a first-order algorithm called PenCF and establish its global convergence and local linear convergence rate under some mild assumptions. For the case that the computation and storage of Hessian is achievable, and we pursue high precision solution and fast local convergence rate, a second-order approach called PenCS is proposed for solving PenC. To avoid expensive calculation or solving a hard subproblem in computing the Newton step, we propose a new strategy to do it approximately which still leads to quadratic convergence locally. Moreover, the main iterations of both PenCF and PenCS are orthonormalization-free and hence parallelizable. Numerical experiments illustrate that PenCF is comparable with the existing first-order methods. Furthermore, PenCS shows its stability and high efficiency in obtaining high precision solution comparing with the existing second-order methods.
更多查看译文
关键词
Orthogonality constraint, Stiefel manifold, augmented Lagrangian method
AI 理解论文
溯源树
样例
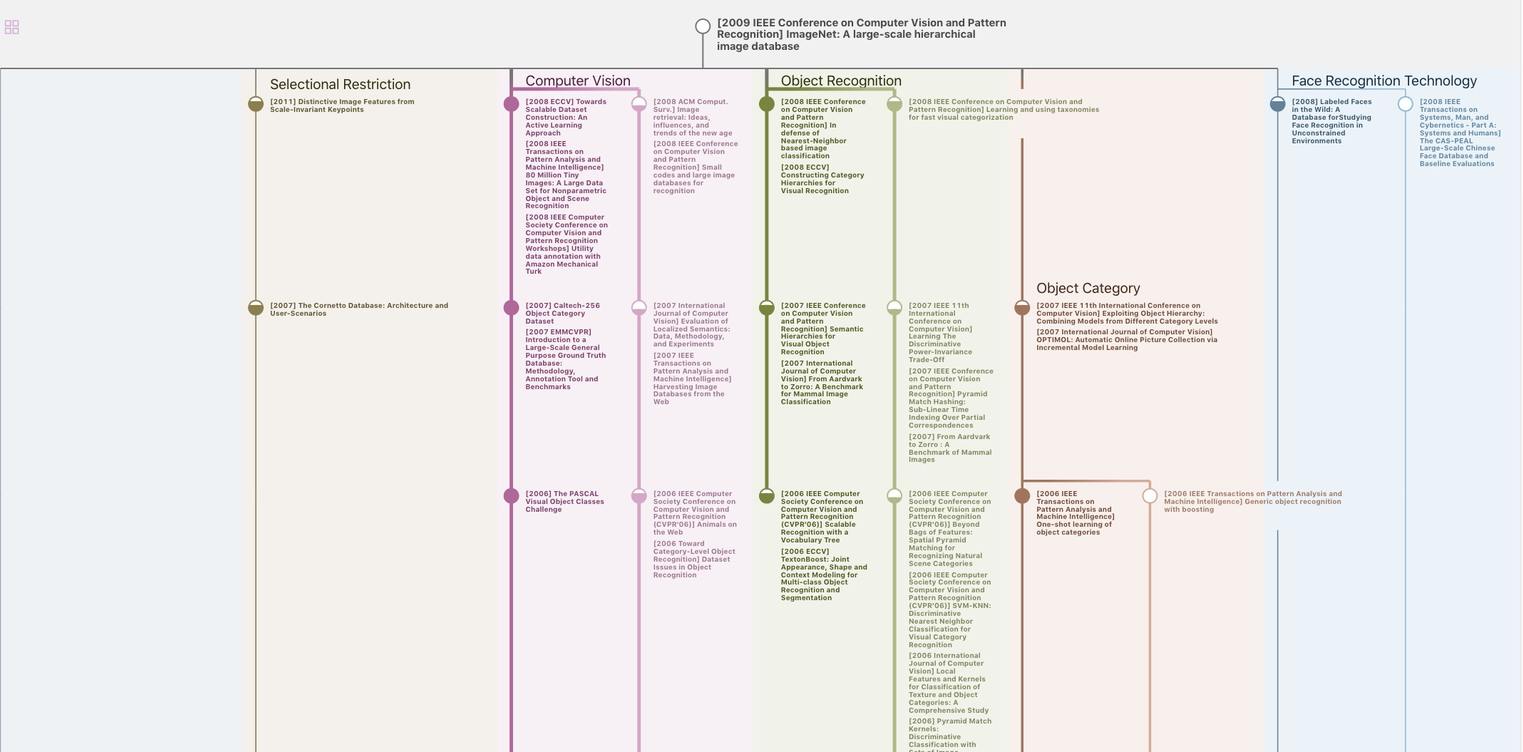
生成溯源树,研究论文发展脉络
Chat Paper
正在生成论文摘要