Semisupervised Multiscale Generative Adversarial Network for Semantic Segmentation of Remote Sensing Image
IEEE GEOSCIENCE AND REMOTE SENSING LETTERS(2022)
摘要
Semantic segmentation of remote sensing images based on deep neural networks has gained wide attention recently. Although many methods have achieved amazing performance, they need large amounts of labeled images to distinguish the differences in angle, color, size, and other aspects for small targets in remote sensing data sets. However, with a few labeled images, it is difficult to extract the key features of small targets. We propose a semisupervised multiscale generative adversarial network (GAN), which not only utilizes the multipath input and atrous spatial pyramid pooling (ASPP) module but leverages unlabeled images and semisupervised learning strategy to improve the performance of small target segmentation in semantic segmentation when labeled data amount is small. Experimental results show that our model outperforms state-of-the-art methods with insufficient labeled data.
更多查看译文
关键词
Image segmentation, Semantics, Remote sensing, Feature extraction, Generative adversarial networks, Gallium nitride, Training, Generative adversarial network (GAN), multiscale, remote sensing, semantic segmentation, semisupervised
AI 理解论文
溯源树
样例
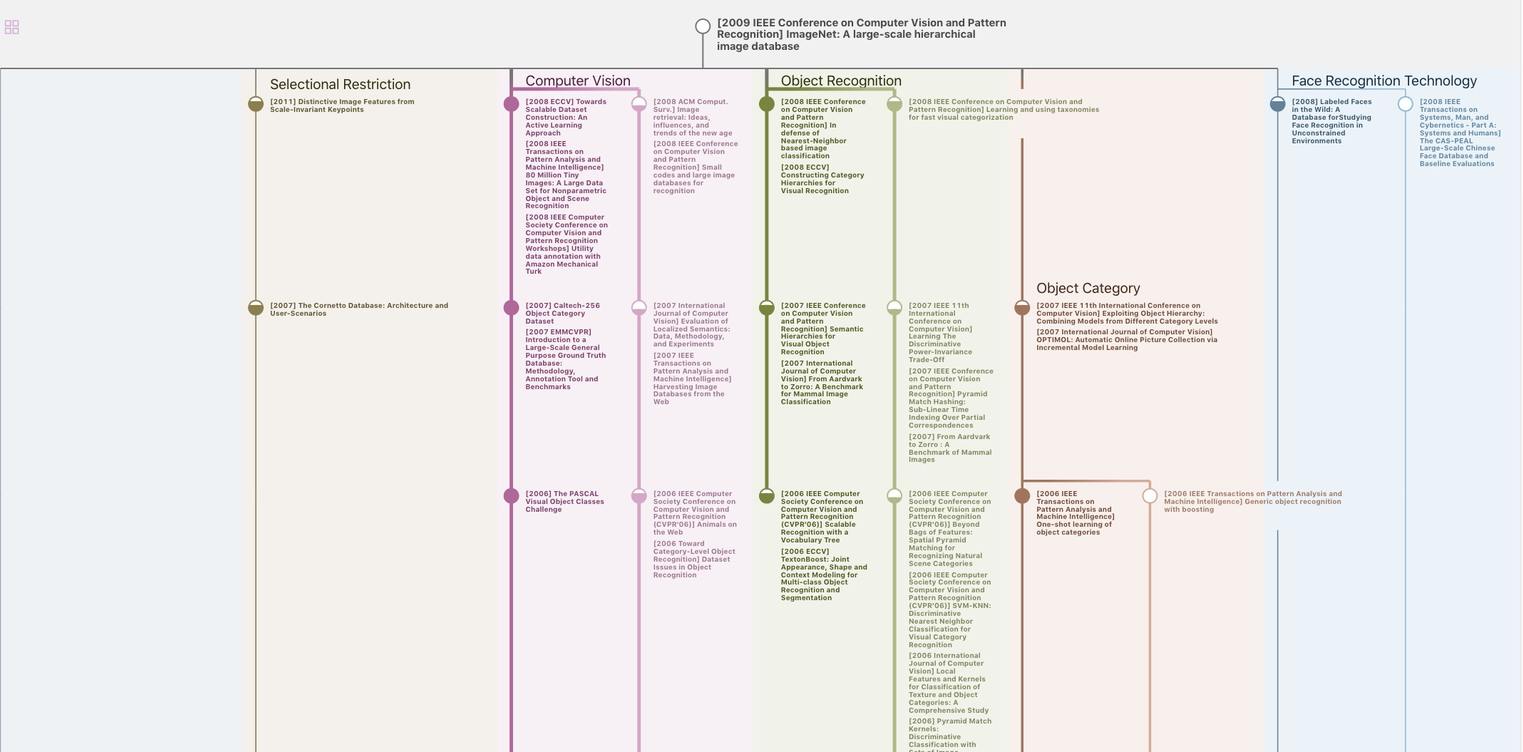
生成溯源树,研究论文发展脉络
Chat Paper
正在生成论文摘要