Improved Genetic Algorithm Approach Based On New Virtual Crossover Operators For Dynamic Job Shop Scheduling
IEEE ACCESS(2020)
摘要
The realtime manufacturing system is subject to different kinds of disruptions such as new job arrivals, machine breakdowns, and jobs cancellation. These different disruptions affect the original schedule that should be updated to maintain the system's performance. An effective re-scheduling is required in this situation to make better utilization of the system resources. This paper studies the dynamic job shop scheduling problem. The problem is known as strongly NP Hard optimization problem where new jobs are unconditionally arrived at the system. Hence, to deal with system changes and performing hard tasks scheduling, we propose an evolutionary genetic algorithm based on virtual crossover operators. Experimental results are compared with state-of-the-art heuristics and metaheuristics dedicated for evaluating large scale instances. Simulation results show the efficiency of the proposed virtual crossover operators integrated into the genetic algorithm approach.
更多查看译文
关键词
Dynamic scheduling, Job shop scheduling, Heuristic algorithms, Schedules, Genetic algorithms, Task analysis, Production, Dynamic job shop scheduling problem, genetic algorithm, crossover operators, makespan, dispatching rules, metaheuristics
AI 理解论文
溯源树
样例
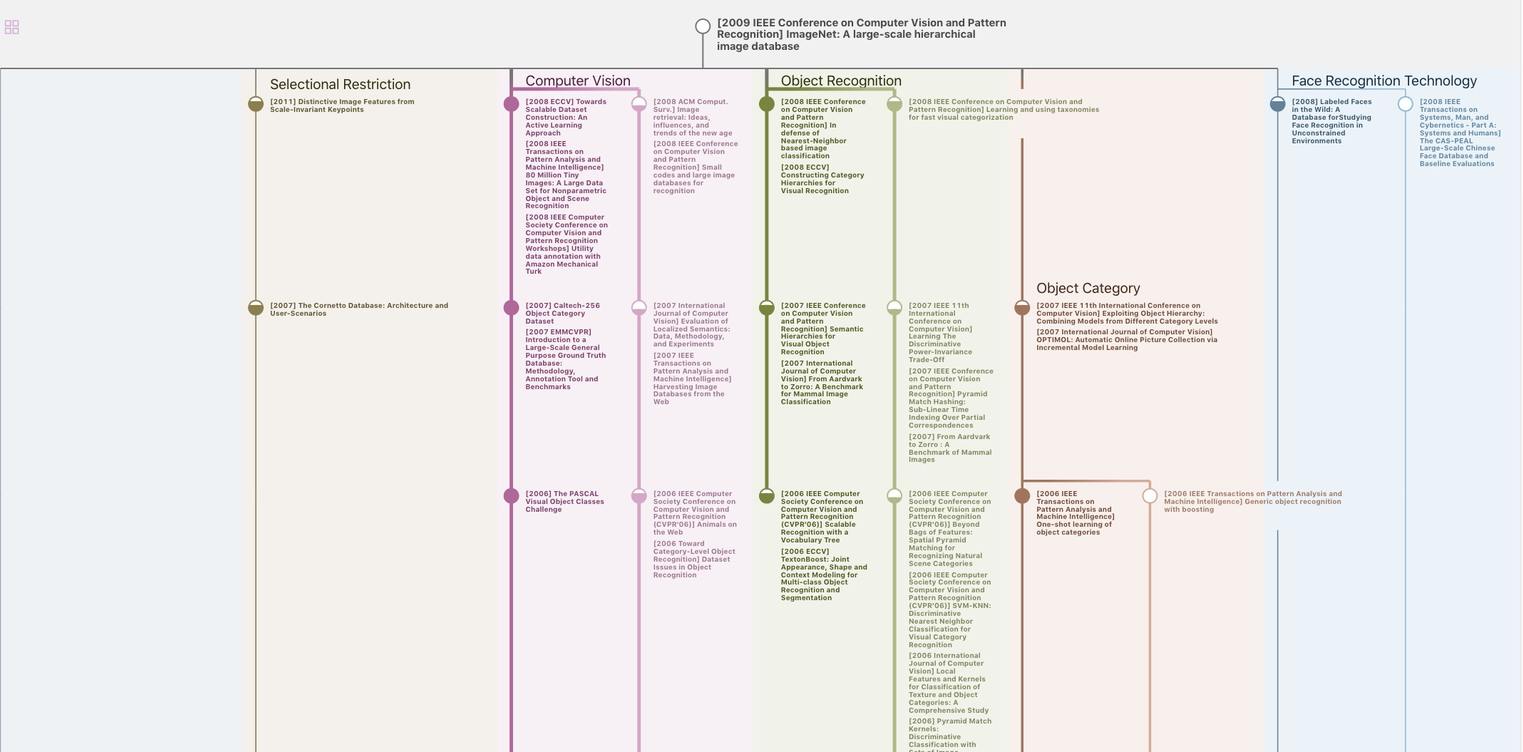
生成溯源树,研究论文发展脉络
Chat Paper
正在生成论文摘要