Deep Neural Networks With Distance Distributions For Gender Recognition Of 3d Human Shapes
IEEE ACCESS(2020)
摘要
Automatic human gender recognition is an important and classical problem in artificial intelligence. Most of the previous gender recognition works are based on vision appearance and biometric characteristics. However, there are fewer gender recognition approaches for 3D human shapes. In this article, we propose a novel deep neural network learning method for gender recognition of 3D human shapes. Firstly, we introduce effective descriptors to distinguish male and female of 3D human shapes via probability distributions of biharmonic distances among points. Secondly, the above distances-based low-level descriptors are fed into a fully connected neural network for gender recognition. Furthermore, we construct a larger 3D human shape dataset for evaluation of the proposed gender recognition method by collecting and labeling human shape models. Compared with previous works, our method obtains higher recognition accuracy and has more advantages, such as posture invariant, robust to noises, and no need of landmarks or pre-alignment process.
更多查看译文
关键词
Shape, Three-dimensional displays, Neural networks, Deep learning, Support vector machines, Probability distribution, Probability density function, Gender recognition, 3D human shape, neural network
AI 理解论文
溯源树
样例
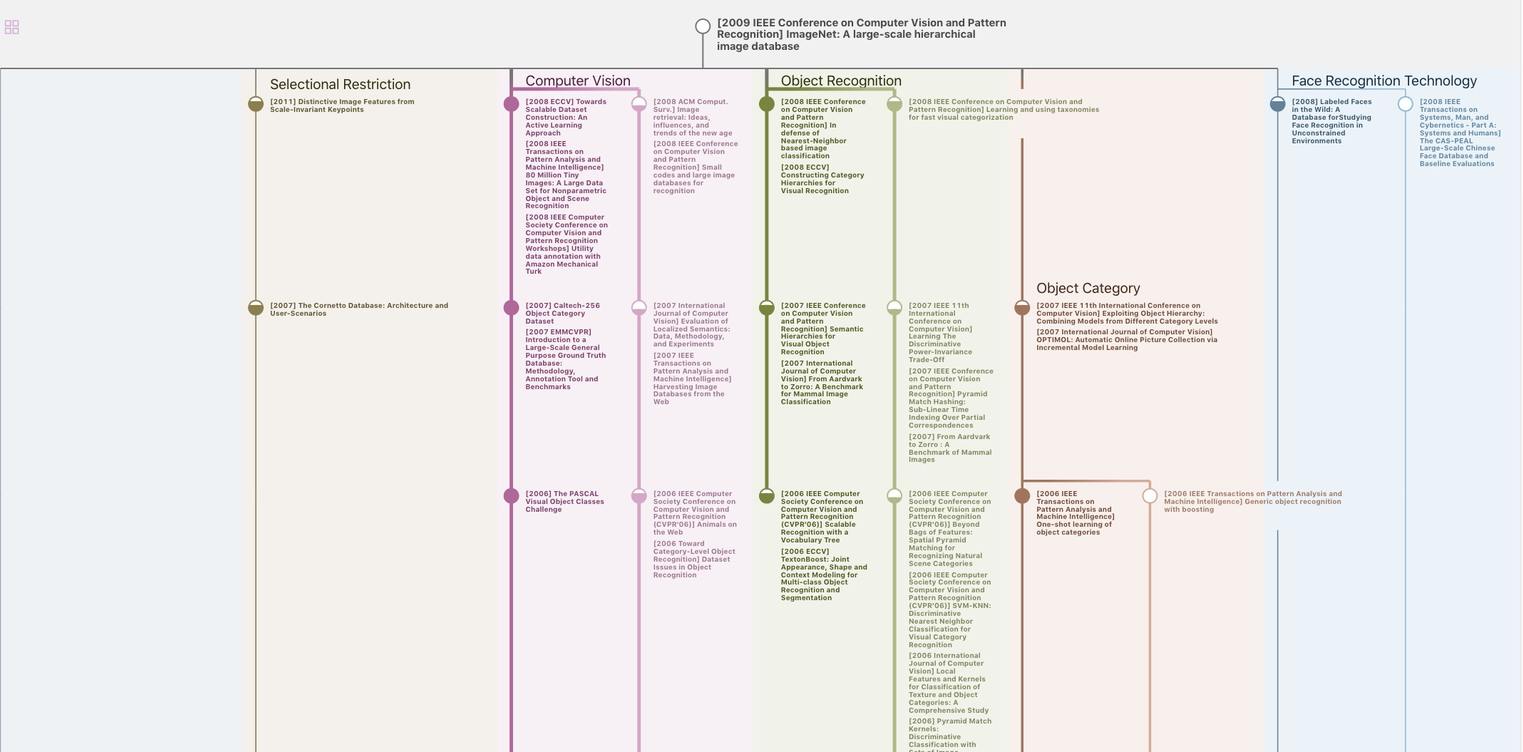
生成溯源树,研究论文发展脉络
Chat Paper
正在生成论文摘要