A Cost-Effective Method for Improving and Re-purposing Large, Pre-trained GANs by Fine-Tuning Their Class-Embeddings.
ACCV (4)(2020)
摘要
Large, pre-trained generative models have been increasingly popular and useful to both the research and wider communities. Specifically, BigGANs a class-conditional Generative Adversarial Networks trained on ImageNet---achieved excellent, state-of-the-art capability in generating realistic photos. However, fine-tuning or training BigGANs from scratch is practically impossible for most researchers and engineers because (1) GAN training is often unstable and suffering from mode-collapse; and (2) the training requires a significant amount of computation, 256 Google TPUs for 2 days or 8xV100 GPUs for 15 days. Importantly, many pre-trained generative models both in NLP and image domains were found to contain biases that are harmful to society. Thus, we need computationally-feasible methods for modifying and re-purposing these huge, pre-trained models for downstream tasks. In this paper, we propose a cost-effective optimization method for improving and re-purposing BigGANs by fine-tuning only the class-embedding layer. We show the effectiveness of our model-editing approach in three tasks: (1) significantly improving the realism and diversity of samples of complete mode-collapse classes; (2) re-purposing ImageNet BigGANs for generating images for Places365; and (3) de-biasing or improving the sample diversity for selected ImageNet classes.
更多查看译文
关键词
gans,cost-effective,re-purposing,pre-trained,fine-tuning,class-embeddings
AI 理解论文
溯源树
样例
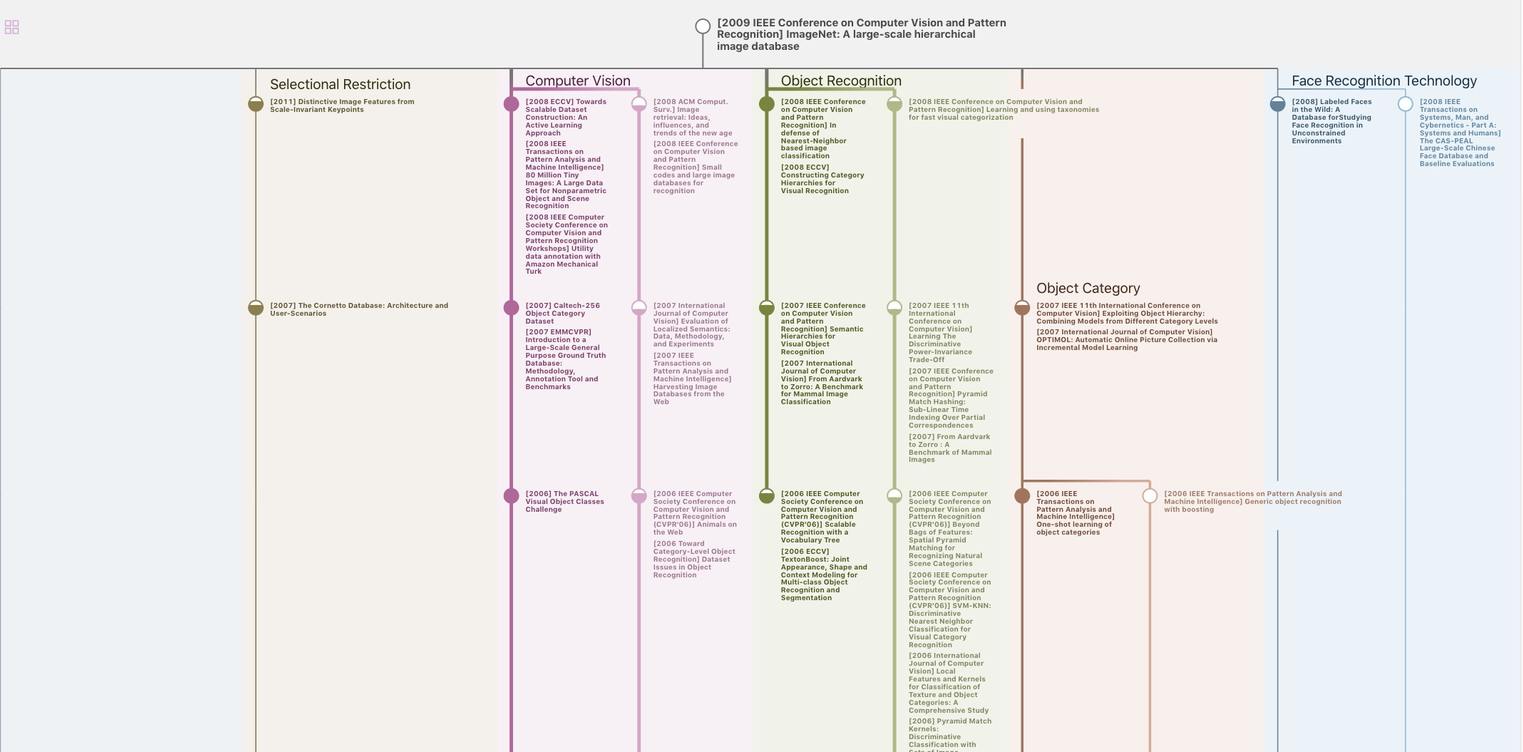
生成溯源树,研究论文发展脉络
Chat Paper
正在生成论文摘要