Emulation Of Community Land Model Version 5 (Clm5) To Quantify Sensitivity Of Soil Moisture To Uncertain Parameters
JOURNAL OF HYDROMETEOROLOGY(2021)
摘要
Land surface models (LSMs) are limited in their ability to reproduce observed soil moisture partially due to uncertainties in model parameters. Here we conduct a variance-based sensitivity analysis to quantify the relative contribution of different model parameters and their interactions to the uncertainty in the surface and root-zone soil moisture in the Community Land Model 5.0 (CLM5). We focus on soil-texture-related parameters (porosity, saturated matric potential, saturated hydraulic conductivity, shape parameter of soil-water retention model) and organic matter fraction. A Gaussian process emulator is constructed based on CLM5 simulations and used to estimate soil moisture across the five-dimensional parameter space for sensitivity analysis. The procedure is demonstrated for four seasons across various U.S. sites of distinct soil and vegetation types. We find that the emulator captures well the CLM5 behavior across the parameter space for different soil textures and seasons. The uncertainties of surface and root-zone soil moisture are dominated by the uncertainties in porosity and shape parameter with negligible parametric interactions. However, relative importance of porosity versus shape parameter varies with soil textures (sites), depths (surface versus root zone), and seasons. At most of the sites, surface soil moisture uncertainty is attributed largely to shape parameter uncertainty, while porosity uncertainty is more important for the root-zone soil moisture uncertainty. All individual parameter and interaction effects demonstrate less variability across different soil textures and seasons for root zone than for surface soil moisture. These results provide scientific guidance to prioritize reducing the uncertainty of sensitive parameters for improving soil moisture modeling with CLM.
更多查看译文
关键词
North America, Soil moisture, Satellite observations, Sensitivity studies, Bayesian methods, Land surface model
AI 理解论文
溯源树
样例
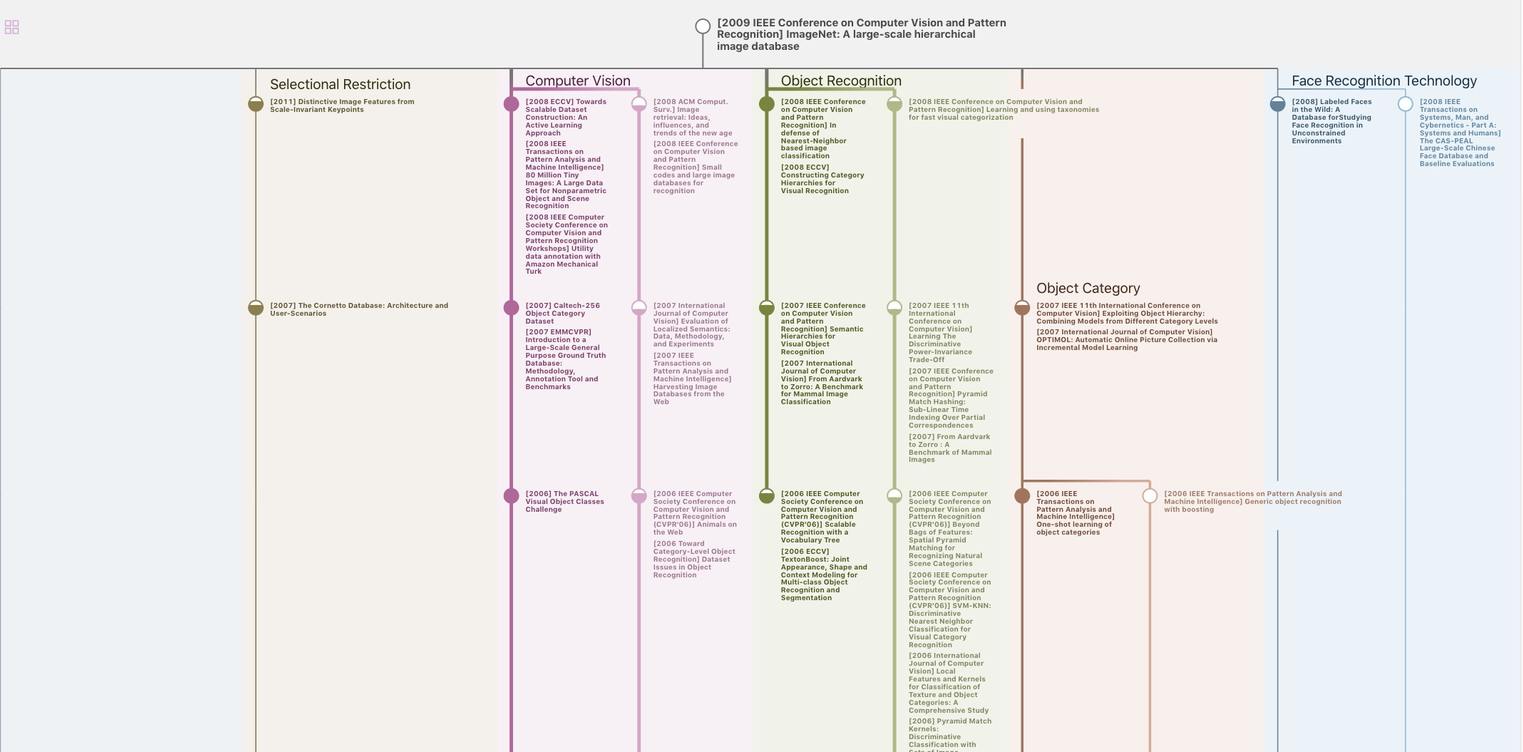
生成溯源树,研究论文发展脉络
Chat Paper
正在生成论文摘要