Fusion Of Amplitude And Complex Domains Based On Deep Neural Networks For Speech Enhancement
2020 28TH IRANIAN CONFERENCE ON ELECTRICAL ENGINEERING (ICEE)(2020)
摘要
Most of the recent works on speech enhancement has estimated the domain of clean spectrum and directly added noisy phase to the estimated domain without any processing. Nowadays, most of the phase-aware systems in speech processing are made up of using both real and imaginary parts of speech spectrum rather than the raw phase. In this paper, we propose a novel approach by the fusion of two deep methods for speech enhancement in the complex domain. This method combines the output of two deep neural networks (DNN) that estimate the complex ideal ratio mask (cIRM) and the amplitude of clean speech with a new logarithmic-based decision rule. This fusion rule which has been proposed according to psychoacoustics findings and spectrogram observations produces a complementary structure. Hence, it is capable of using the advantages of both amplitude and complex mask estimators in each time-frequency region. The above method, when evaluated on TIMIT corpus, outperforms the perceptual evaluation of speech quality (PESQ) compared to other approaches especially in unseen noise conditions showing the better generalization of the proposed architecture.
更多查看译文
关键词
speech enhancement, deep neural networks, complex ideal ratio mask
AI 理解论文
溯源树
样例
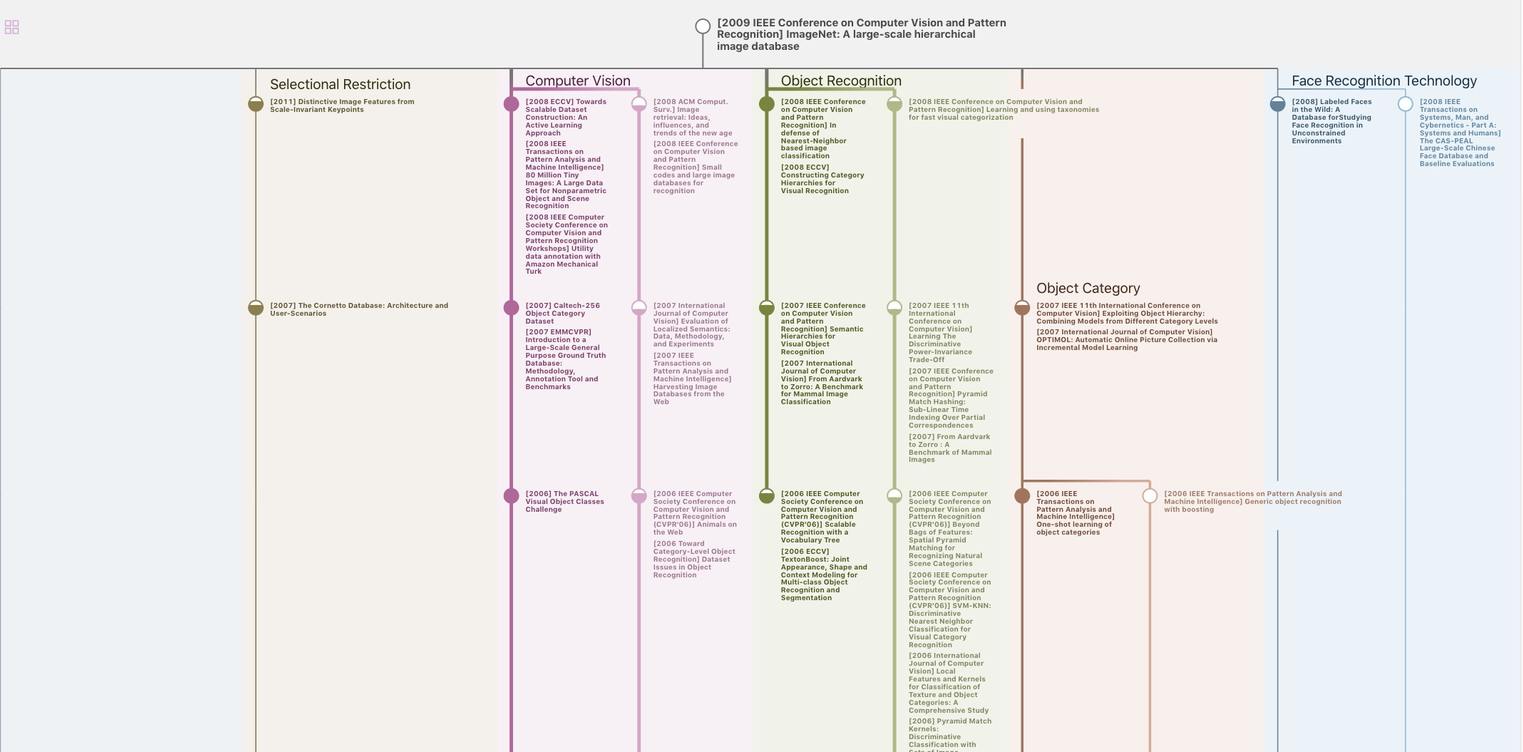
生成溯源树,研究论文发展脉络
Chat Paper
正在生成论文摘要