Topic attention encoder: A self-supervised approach for short text clustering
JOURNAL OF INFORMATION SCIENCE(2022)
摘要
Short text clustering is a challenging and important task in many practical applications. However, many Bag-of-Word-based methods for short text clustering are often limited by the sparsity of text representation, while many sentence embedding-based methods fail to capture the document structure dependencies within a text corpus. In considerations of the shortcomings of many existing studies, a topic attention encoder (TAE) is proposed in this study. Given topics derived from corpus by the techniques of topic modelling, the cross-document information is introduced. This encoder assumes the document-topic vector to be the learning target and the concatenating vectors of the word embedding and corresponding topic-word vector to be the input. Also, a self-attention mechanism is employed in the encoder, which aims to extract weights of hidden states adaptively and encode the semantics of each short text document. With captured global dependencies and local semantics, TAE integrates the superiority of Bag-of-Word methods and sentence embedding methods. Finally, categories of benchmarking experiments were conducted by analysing three public data sets. It demonstrates that the proposed TAE outperforms many document representation benchmark methods for short text clustering.
更多查看译文
关键词
Global dependency, local semantic, self-attention, short text clustering, text representation, topic attention encoder
AI 理解论文
溯源树
样例
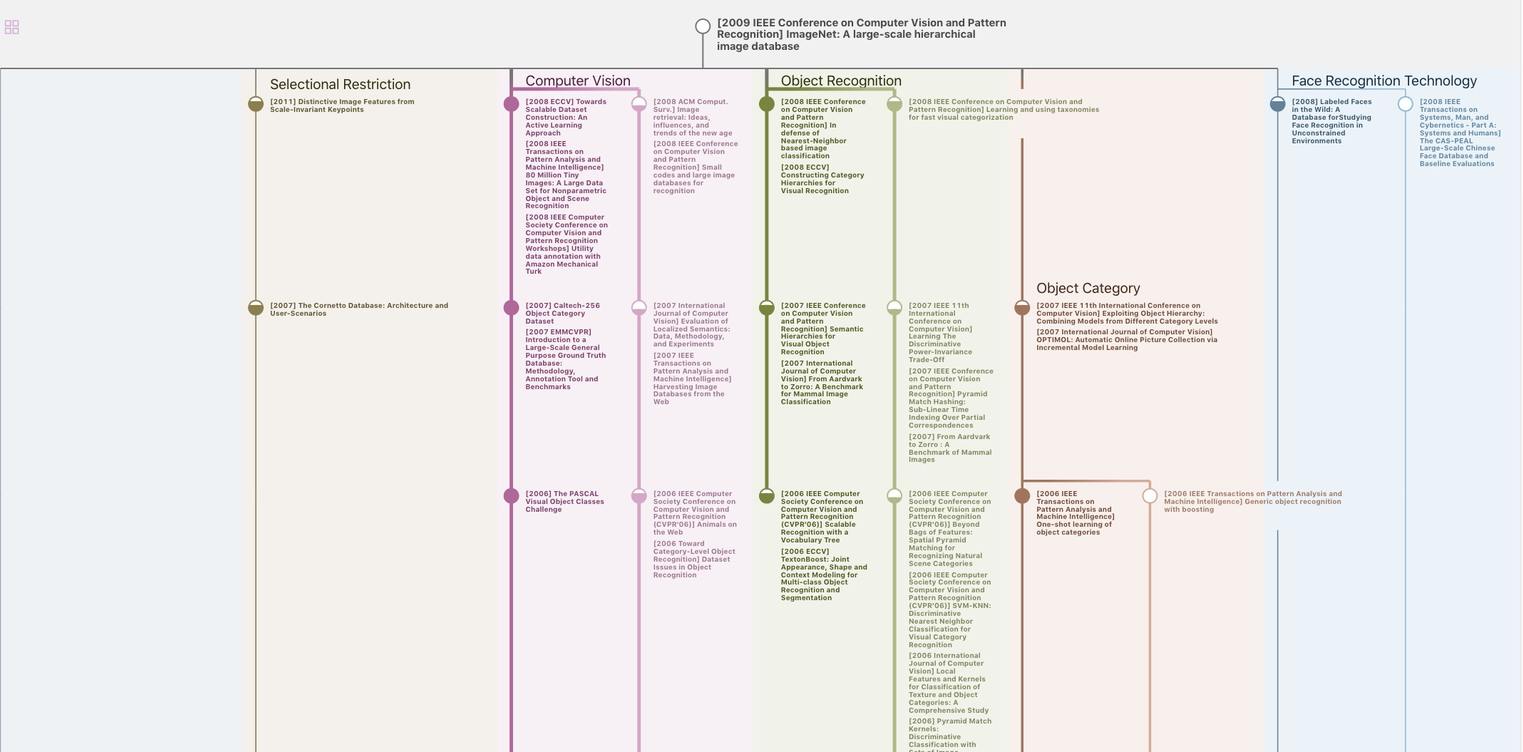
生成溯源树,研究论文发展脉络
Chat Paper
正在生成论文摘要