Minimization of the logarithmic function in sparse recovery.
Neurocomputing(2021)
摘要
In sparse information recovery, the key issue is to solve the l0-minimization which is NP-hard. Therefore we consider the logarithmic alternative function to replace 0-norm. In this paper, theoretical analysis of logarithmic alternative model is given in detail. First, we discuss the equivalence between l0-minimization and the logarithmic function minimization. It is proved that the solution of the alternative model also solves the l0-minimization in both noiseless and noisy cases. Second, the recovery condition of logarithmic minimization is presented. By this new condition and a new concept named Logarithms Null Space Constant (L-NSC), we demonstrate the advantages of this model over classical approaches. Third, we study the optimal properties of the alternative model including the analytic property of optimal solution, a fixed point iterative algorithm and the corresponding convergence conclusion. Finally, we use the logarithmic function and the proposed algorithm to solve the multi-source time difference of arrival (TDOA) location problem. Compared with classic approaches, the experimental results demonstrate the effectiveness of the proposed algorithm.
更多查看译文
关键词
L0-minimization,Sparse recovery,Equivalence,Iterative algorithms
AI 理解论文
溯源树
样例
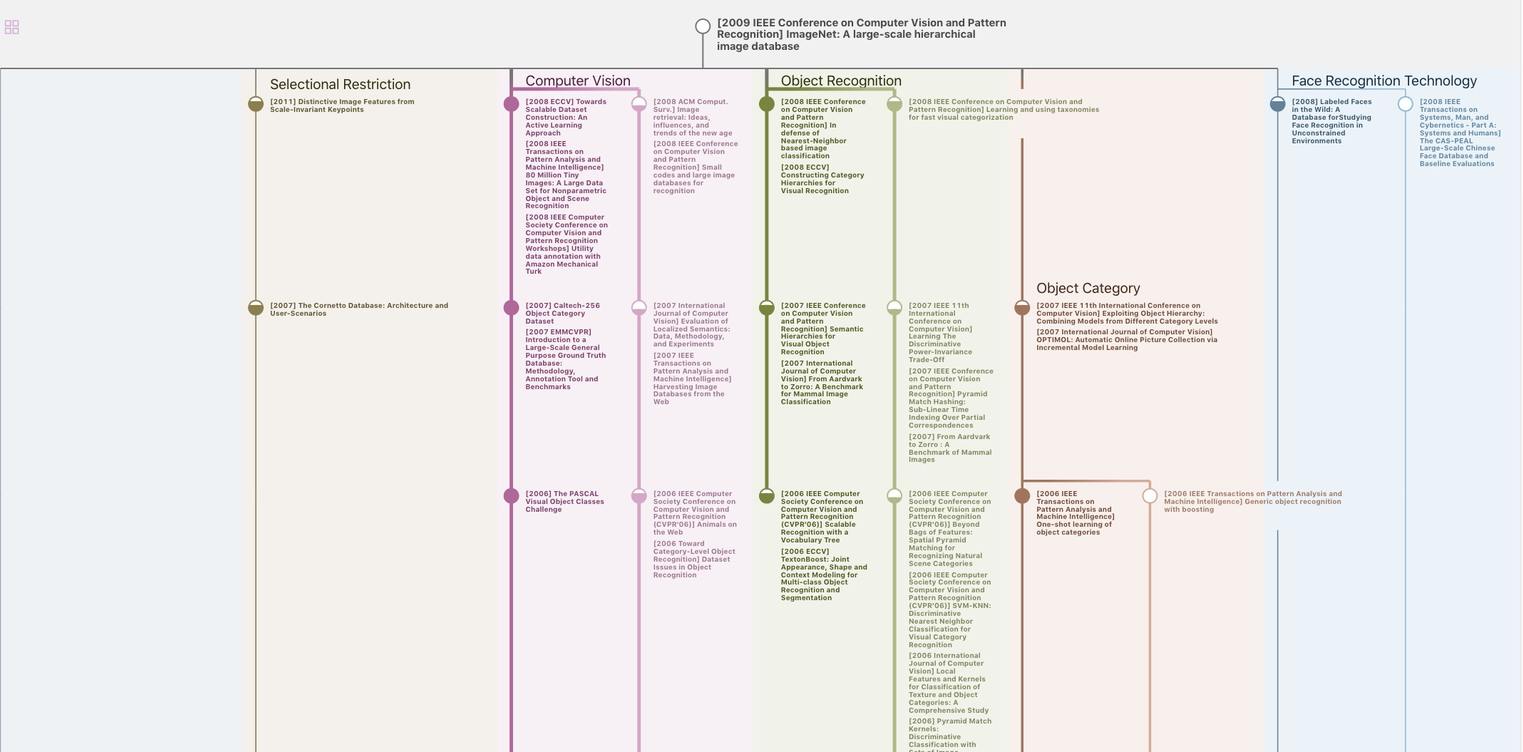
生成溯源树,研究论文发展脉络
Chat Paper
正在生成论文摘要