Learning Fundamental Visual Concepts Based on Evolved Multi-Edge Concept Graph
IEEE TRANSACTIONS ON MULTIMEDIA(2021)
摘要
In general, visual media comprises a set of elements of basic semantics, named fundamental visual concepts, that may not be semantically decomposed, such as objects, scenes and actions. This paper proposes a dynamic learning framework for fundamental visual concept learning from image-textual description paired data based on an evolved multi-edge concept graph (EMCG). First, we construct a multi-edge concept graph to represent the relationships between visual concept instances, in which we introduce two types of edges named visual edges and semantic edges to describe the connection strength in terms of visual appearance and semantic content. Second, we evolve the graph by updating connection strength based on the predicted results of concept learning. Finally, we present a growth algorithm for the multi-edge concept graph to handle cross-dataset concept learning. Driven by the predictions, the multi-edge concept graph can dynamically evolve over time by adjusting the connection strength to adapt better to the observations. In addition, our approach can be considered a weakly-supervised learning algorithm since no labeled concepts are employed for learning. Experimental results demonstrate that evolution can significantly improve the learning of fundamental visual concepts by 14.2%, 7.9% and 12.7% in terms of F1-score for the MSRC, VOC2012 and MSCOCO datasets, respectively, and that the proposed EMCG approach largely outperforms the compared approaches.
更多查看译文
关键词
Fundamental visual concepts, concept graph, model evolution, cross media
AI 理解论文
溯源树
样例
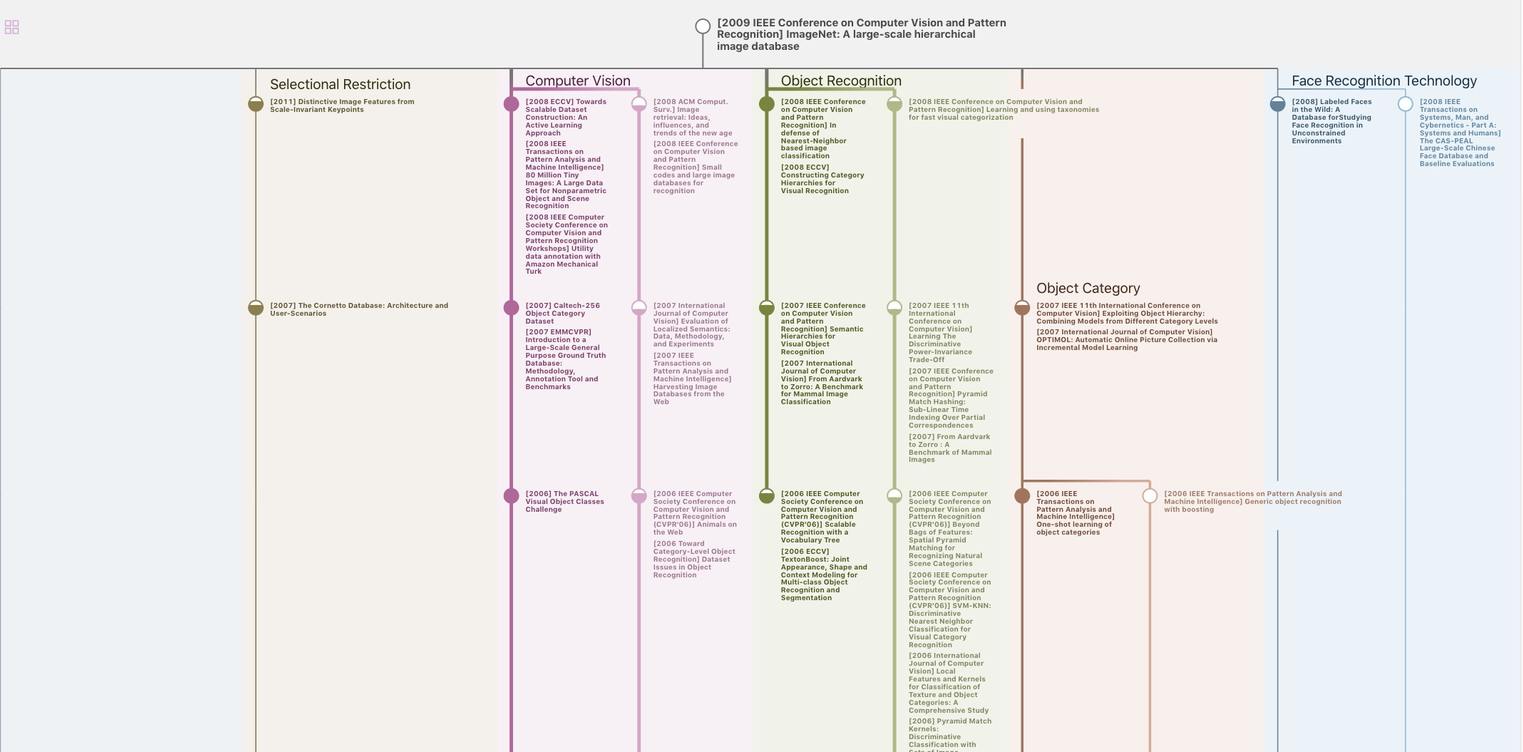
生成溯源树,研究论文发展脉络
Chat Paper
正在生成论文摘要