A Rough Set And Cellular Genetic Fusion Algorithm For Acute Critical Disease Prediction
INTERNATIONAL JOURNAL OF COMPUTERS COMMUNICATIONS & CONTROL(2020)
摘要
This study is to solve the problems of an overly-broad scale of medical indicators, lack of retrospective research samples, insufficient depth of data mining, and low disease prediction accuracy. In this paper, we propose an intelligent screening algorithm that combines a genetic algorithm, cellular automata, and rough set theory. This algorithm can achieve high accuracy in predicting patient outcomes with a small number of indicators. And we compare it with the traditional genetic algorithm. We built the prediction model with 64 indicators based on the logistic regression (AUC 0.8628), support vector machine (AUC 0.5319), Naive Bayes (AUC 0.7102), and AdaBoost algorithms (AUC 0.9095). Using the cellular genetic algorithm for attribute screening not only effectively reduces the number of indicators but also achieve almost the same accuracy of prediction with 8 indicators based on the logistic regression (AUC 0.8782), support vector machine (AUC 0.8525), Naive Bayes (AUC 0.8408), and AdaBoost algorithms (AUC 0.8770). Compared with the traditional scoring system, the predictive model established in this paper can more accurately predict rebleeding accidents based on physiological test indicators and continuous patient indicators.
更多查看译文
关键词
cellular genetic algorithm, key indicator, disease prediction, machine learning
AI 理解论文
溯源树
样例
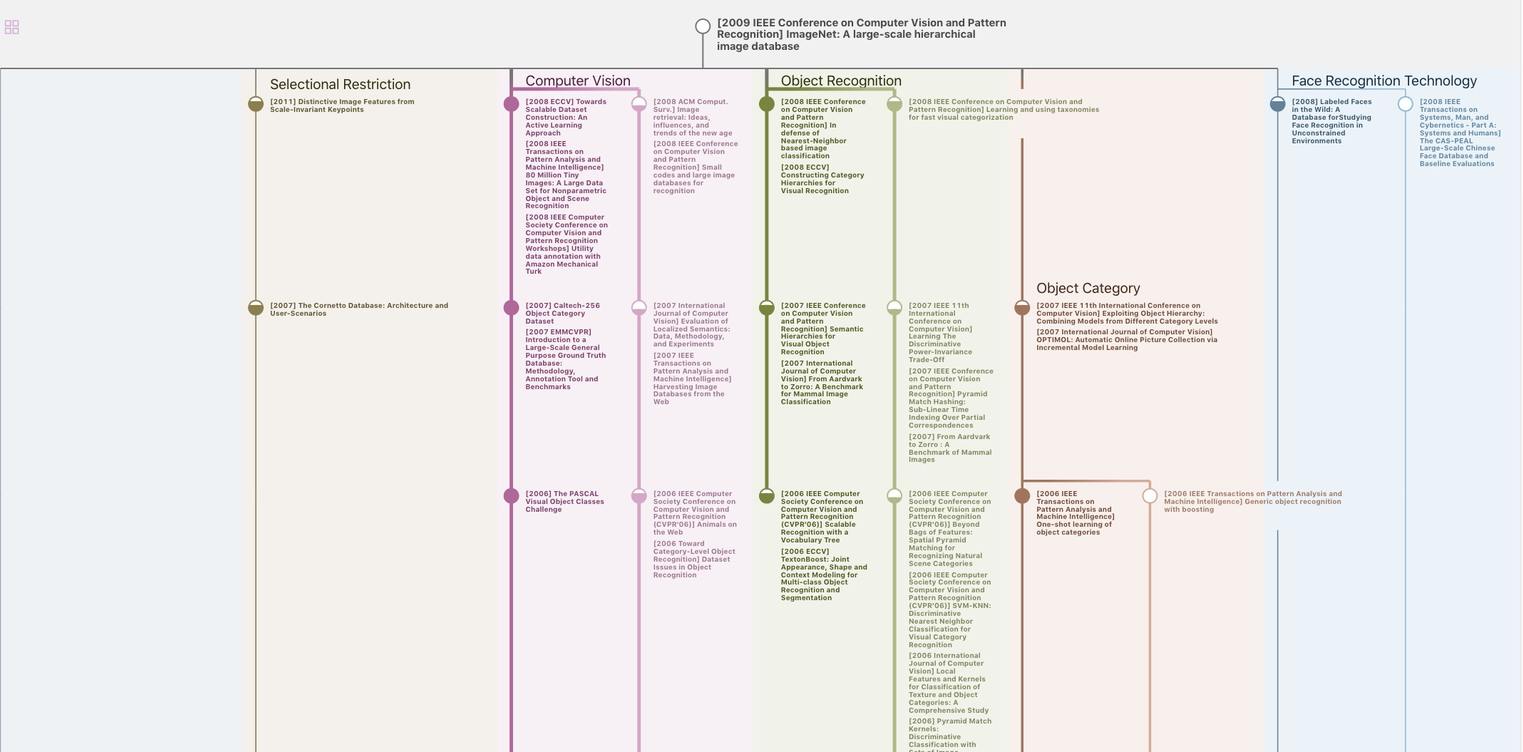
生成溯源树,研究论文发展脉络
Chat Paper
正在生成论文摘要