Framework To Diagnose The Metabolic Syndrome Types Without Using A Blood Test Based On Machine Learning
APPLIED SCIENCES-BASEL(2020)
摘要
Metabolic Syndrome (MetS) is a set of risk factors that increase the probability of heart disease or even diabetes mellitus. The diagnosis of the pathology implies compliance with at least three of five risk factors. Doctors obtain two of those factors in a medical consultation: waist circumference and blood pressure. The other three factors are biochemical variables that require a blood test to determine triglyceride, high-density lipoprotein cholesterol, and fasting plasma glucose. Consequently, scientists are developing technology for non-invasive diagnostics, but medical personnel also need the risk factors involved in MetS to start a treatment. This paper describes the segmentation of MetS into ten types based on harmonized Metabolic Syndrome criteria. It proposes a framework to diagnose the types of MetS based on Artificial Neural Networks and Random undersampling Boosted tree using non-biochemical variables such as anthropometric and clinical information. The framework works over imbalanced and balanced datasets using the Synthetic Minority Oversampling Technique and for validation uses random subsampling to get performance evaluation indicators between the classifiers. The results showed an excellent framework for diagnosing the 10 MetS types that have Area under Receiver Operating Characteristic (AROC) curves with a range of 71% to 93% compared with AROC 82.86% from traditional MetS.
更多查看译文
关键词
metabolic syndrome, Quine–, McCluskey algorithm, SMOTE, artificial neural networks, random undersampling boosted tree, diagnostic non-invasive, harmonized the metabolic syndrome criterion, random subsampling validation
AI 理解论文
溯源树
样例
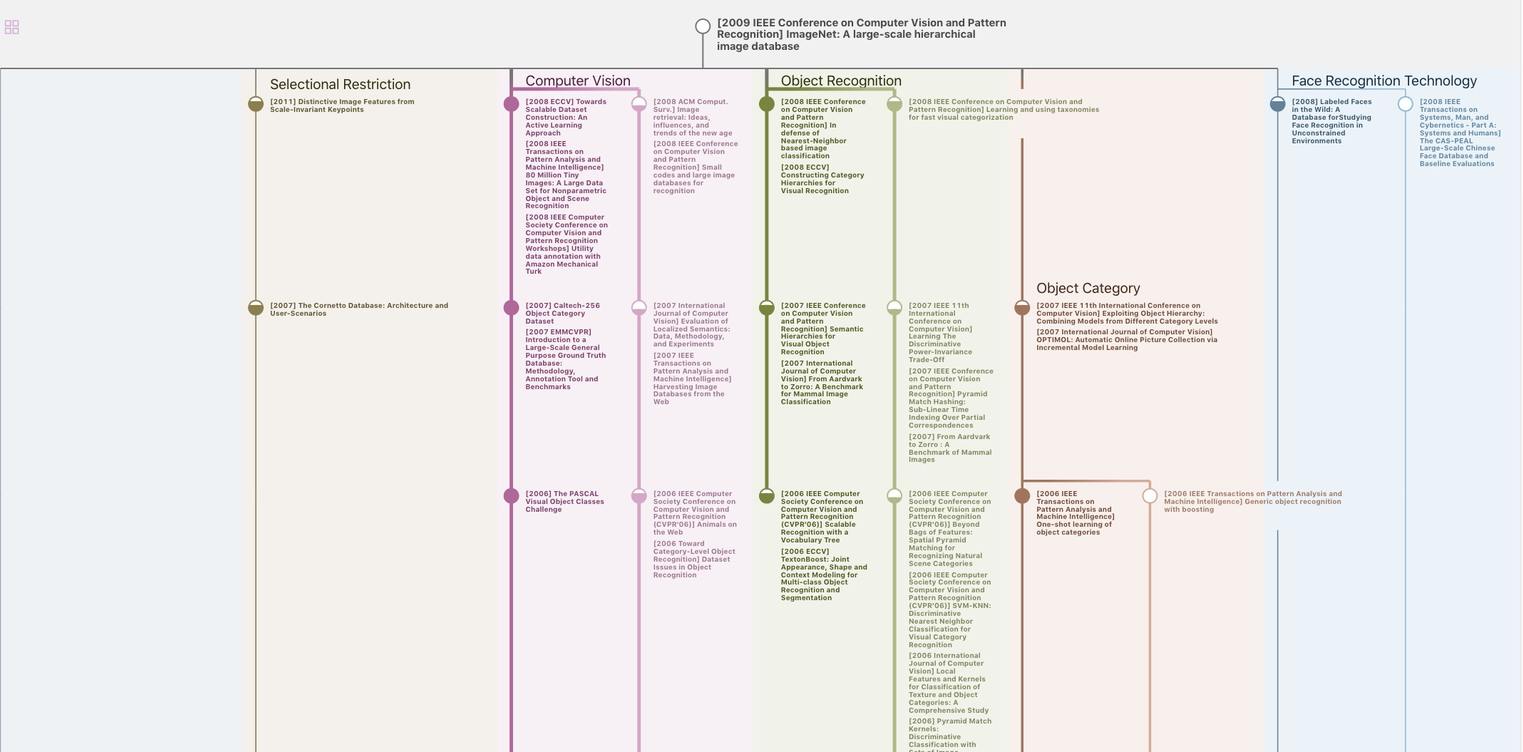
生成溯源树,研究论文发展脉络
Chat Paper
正在生成论文摘要