Deep Learning Model To Detect Various Synchrophasor Data Anomalies
IET GENERATION TRANSMISSION & DISTRIBUTION(2020)
摘要
High-density synchrophasors provide valuable information for power grid situational awareness, operation and control. Unfortunately, due to factors including communication instability and hardware failure, their data quality can be greatly deteriorated by anomalies. Since the anomalies can impact the performance of the synchrophasor applications, it is of paramount significance to propose a model to detect anomalies in synchrophasor. In this study, a convolutional neural network model is established to detect and classify the anomalies in the synchrophasor measurements. Four types of anomalies observed in actual synchrophasors including erroneous patterns, random spikes, missing points and high-frequency interferences are considered in this study. The proposed model is extensively evaluated via field-collected measurements from the synchrophasor network in Jiangsu grid, China. The superior performance of the proposed model indicates the great potential of using deep learning for the detection of abnormal synchrophasor measurements.
更多查看译文
关键词
learning (artificial intelligence), phase measurement, power system measurement, phasor measurement, power grids, power engineering computing, pattern classification, convolutional neural nets, synchrophasor data anomaly detection, high-density synchrophasors, communication instability, hardware failure, data quality, convolutional neural network model, synchrophasor network, abnormal synchrophasor measurements, deep learning, Jiangsu grid, China, synchrophasor data anomaly classification
AI 理解论文
溯源树
样例
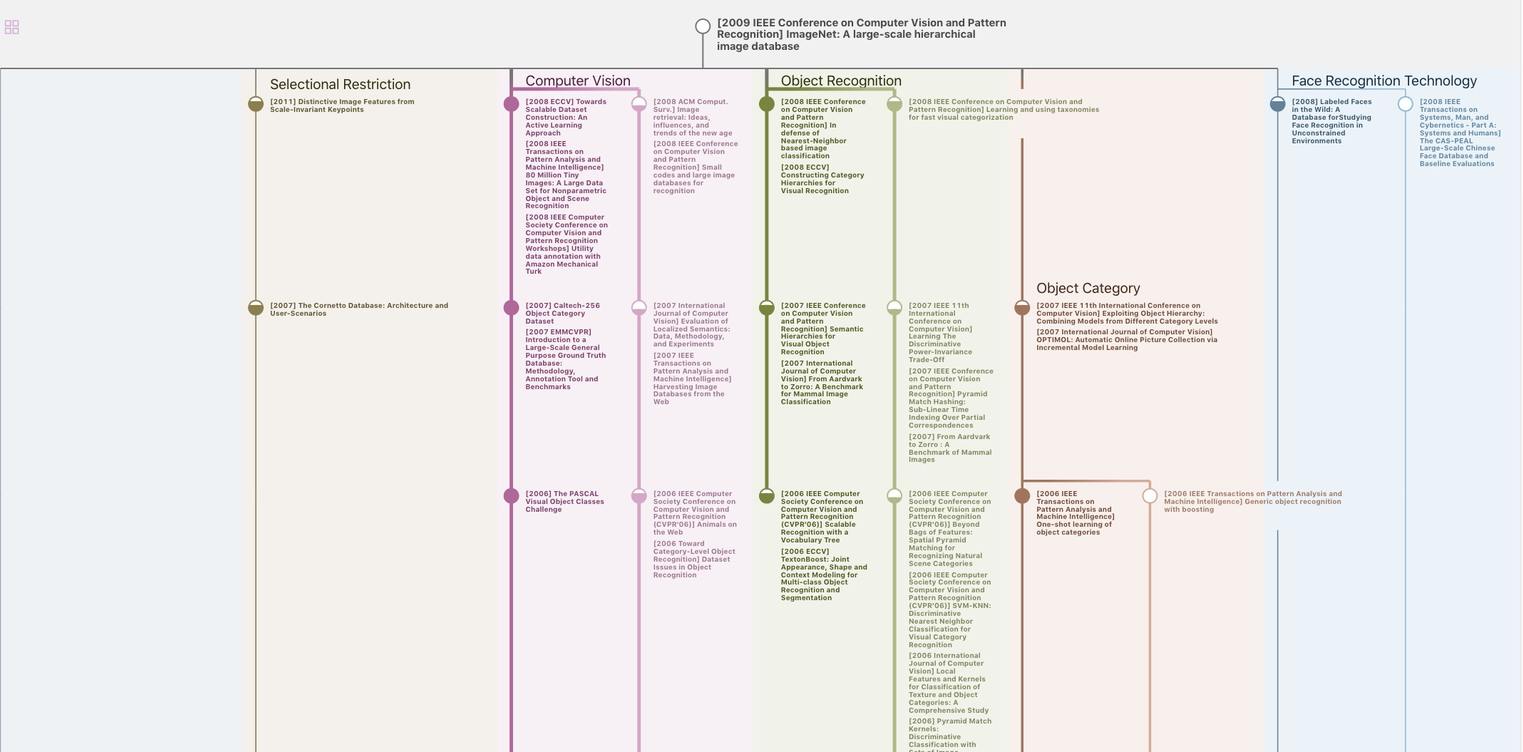
生成溯源树,研究论文发展脉络
Chat Paper
正在生成论文摘要