MULTI-ARMED BANDITS WITH COVARIATES: THEORY AND APPLICATIONS
STATISTICA SINICA(2021)
摘要
"Multi-armed bandits" were introduced as a new direction in the thennascent field of sequential analysis, developed during World War II in response to the need for more efficient testing of anti-aircraft gunnery, and later as a concrete application of dynamic programming and optimal control of Markov decision processes. A comprehensive theory that unified both directions emerged in the 1980s, providing important insights and algorithms for diverse applications in many science, technology, engineering and mathematics fields. The turn of the millennium marked the onset of a "personalization revolution," from personalized medicine and online personalized advertising and recommender systems (e.g. Netflix's recommendations for movies and TV shows, Amazon's recommendations for products to purchase, and Microsoft's Matchbox recommender). This has required an extension of classical bandit theory to nonparametric contextual bandits, where "contextual" refers to the incorporation of personal information as covariates. Such theory is developed herein, together with illustrative applications, statistical models, and computational tools for its implementation.
更多查看译文
关键词
Contextual multi-armed bandits, c-greedy randomization, personalized medicine, recommender system, reinforcement learning
AI 理解论文
溯源树
样例
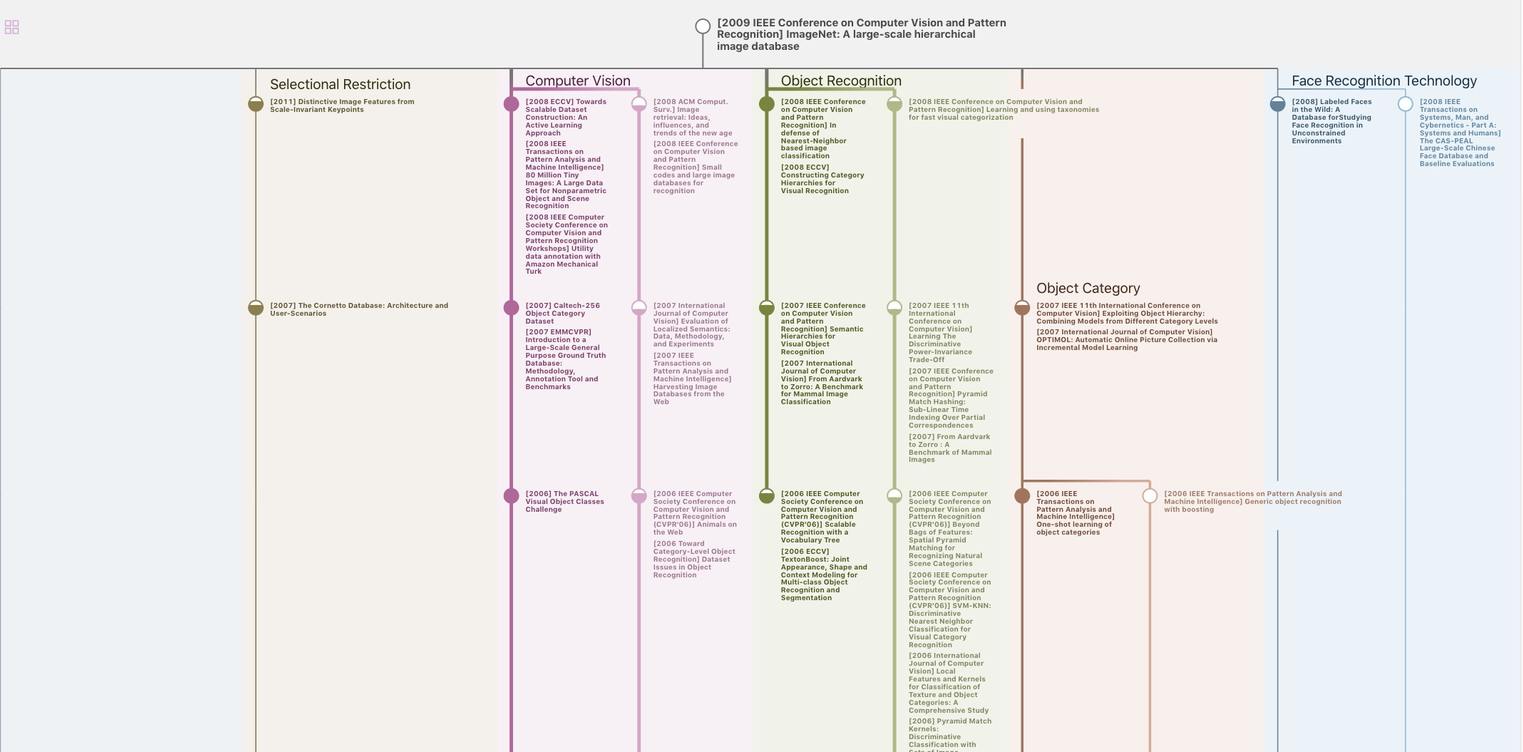
生成溯源树,研究论文发展脉络
Chat Paper
正在生成论文摘要