Resolution Improvement With A Fully Convolutional Neural Network Applied To Aligned Per-Channel Data
PROCEEDINGS OF THE 2020 IEEE INTERNATIONAL ULTRASONICS SYMPOSIUM (IUS)(2020)
摘要
The lateral resolution of ultrasound images is determined by the size of the imaging aperture. Computational techniques have been proposed to improve resolution beyond the diffraction limit (Minimum Variance and Compressive Beamforming), but they lack robustness in real-life scenarios and have high computational cost.We present and evaluate a beamforming paradigm wherein the "sum" part of the delay-and-sum algorithm is replaced by a neural network. Our hypothesis is that the neural network can learn what off-axis signals look like in the raw channel-time domain data and filter them out in the reconstruction, yielding better resolved images.We use a fully convolutional neural network and train it with simulations. The input of the network is per-channel aligned data from a sector array with N elements and the target is beam-summed data from an array of 2N elements. The network is tested on simulations, a resolution phantom, and in vivo cardiac datasets.The network output has a better resolution than the input from the N array, matching that of the target 2N array, as validated with the simulation and phantom datasets. In vivo datasets at network output show improved resolution and no visible artefacts. The speckle of the network output does not match that of the 2N array, possibly due to loss of correlation of per-channel data across the receive aperture. Inference is similar to 1s / frame on GPU, much faster than our experience of CPU-based adaptive beamforming.
更多查看译文
关键词
Beamforming, Convolutional Neural Network, resolution, superresolution
AI 理解论文
溯源树
样例
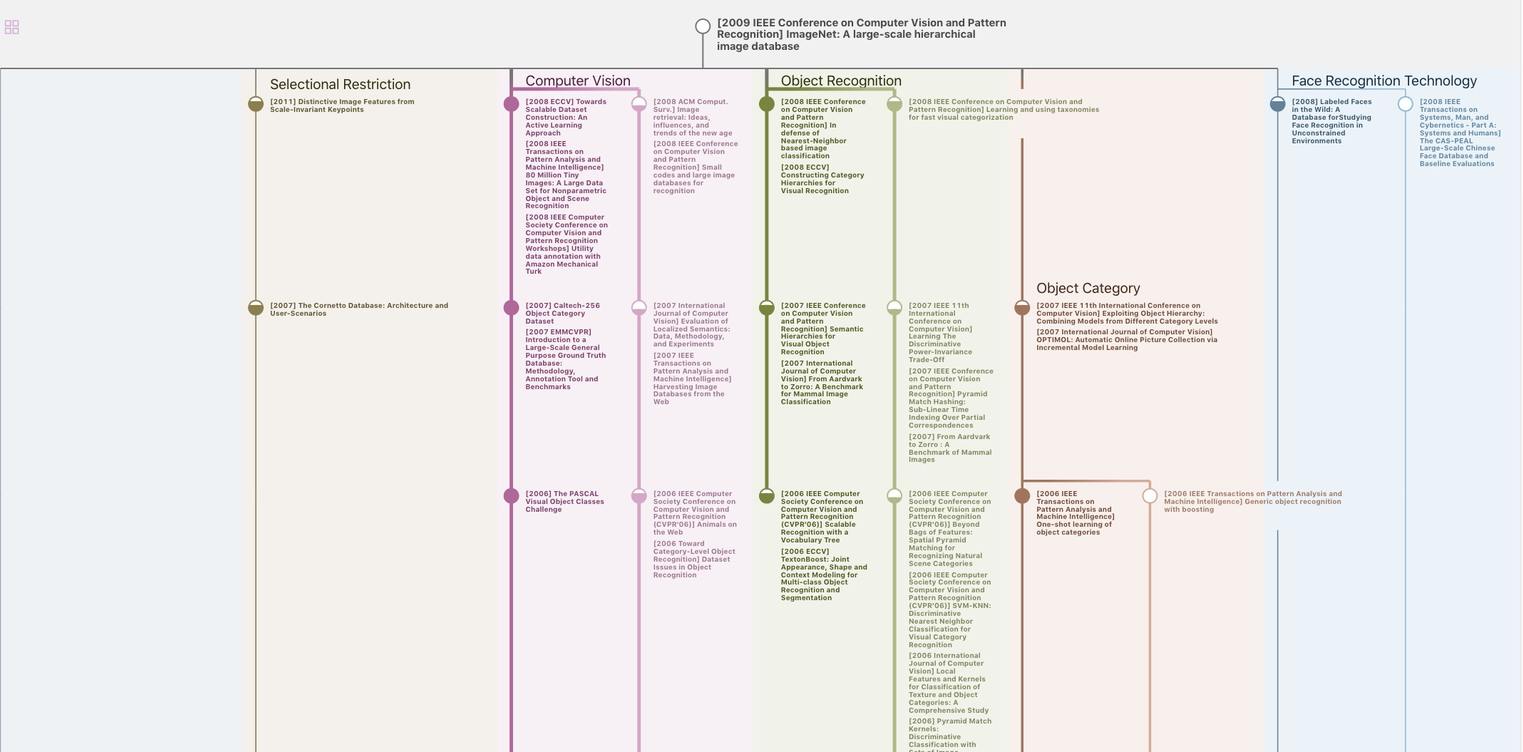
生成溯源树,研究论文发展脉络
Chat Paper
正在生成论文摘要