Influence Of The Receptive Field Size On Accuracy And Performance Of A Convolutional Neural Network
2020 VI INTERNATIONAL CONFERENCE ON INFORMATION TECHNOLOGY AND NANOTECHNOLOGY (IEEE ITNT-2020)(2020)
摘要
Convolutional neural networks (CNNs) have been successfully applied to many tasks such as digit and object recognition. In this paper we study the size of the receptive field of deep convolutional neural networks, in particular, we check the idea of a "redundant" receptive field. We run a set of experiments on two common CNN models - VGG16 and ResNet18 - in order to explore the influence of receptive field size on CNN's training time, accuracy, and performance. We run experiments using the MakiFlow framework on the CALTECH256 dataset. The experiments' results show that the optimization of neural networks (NNs) by reducing the size of the receptive field allows to reduce the NN's training time by 5-7% while maintaining the accuracy of the network.
更多查看译文
关键词
convolution, neural networks, convolutional network, receptive field, classification, ResNet18, VGG16
AI 理解论文
溯源树
样例
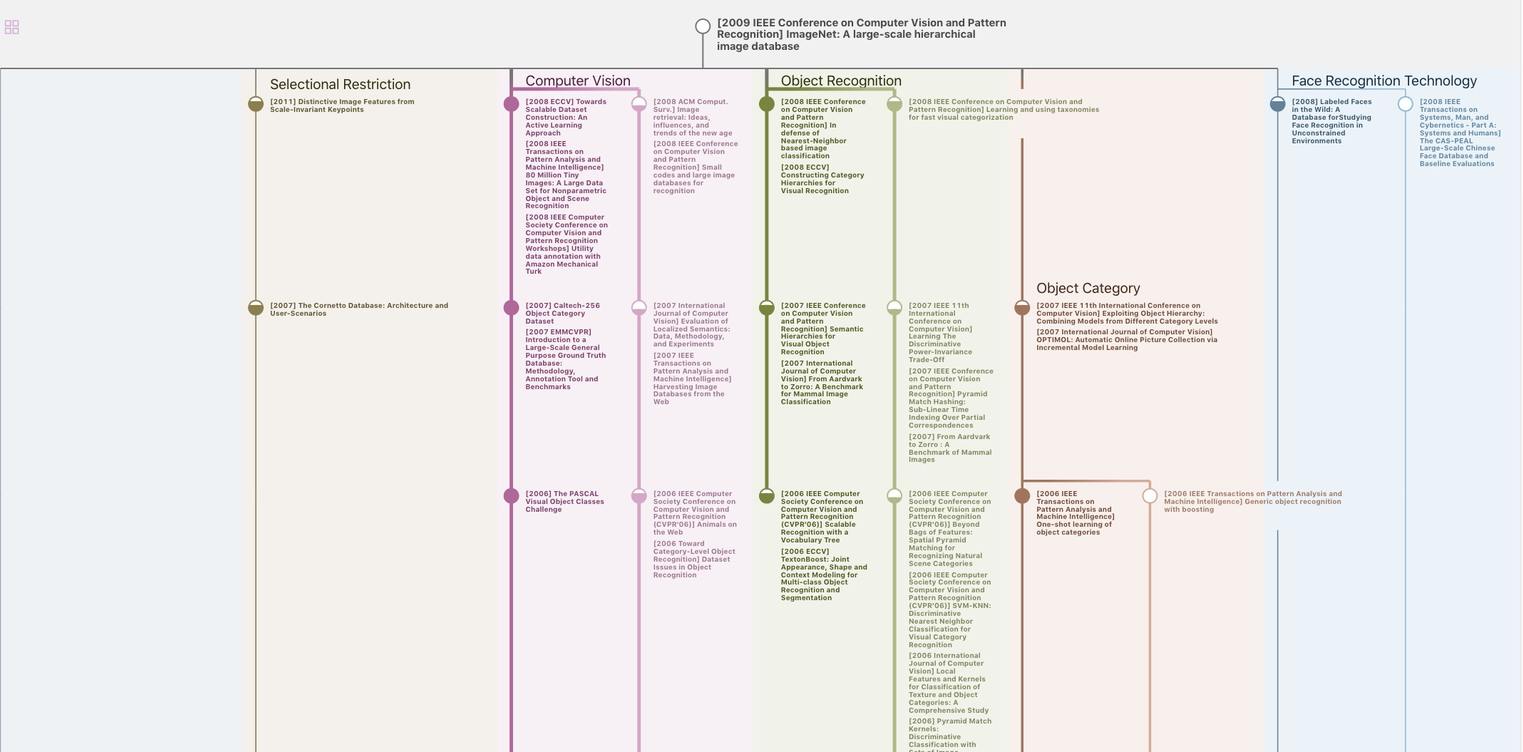
生成溯源树,研究论文发展脉络
Chat Paper
正在生成论文摘要