Complex Imaging Of Phase Domains By Deep Neural Networks
IUCRJ(2021)
摘要
The reconstruction of a single-particle image from the modulus of its Fourier transform, by phase-retrieval methods, has been extensively applied in X-ray structural science. Particularly for strong-phase objects, such as the phase domains found inside crystals by Bragg coherent diffraction imaging (BCDI), conventional iteration methods are time consuming and sensitive to their initial guess because of their iterative nature. Here, a deep-neural-network model is presented which gives a fast and accurate estimate of the complex single-particle image in the form of a universal approximator learned from synthetic data. A way to combine the deep-neural-network model with conventional iterative methods is then presented to refine the accuracy of the reconstructed results from the proposed deep-neural-network model. Improved convergence is also demonstrated with experimental BCDI data.
更多查看译文
关键词
machine learning, Bragg coherent X-ray diffraction, phase retrieval, single-particle imaging, deep neural networks
AI 理解论文
溯源树
样例
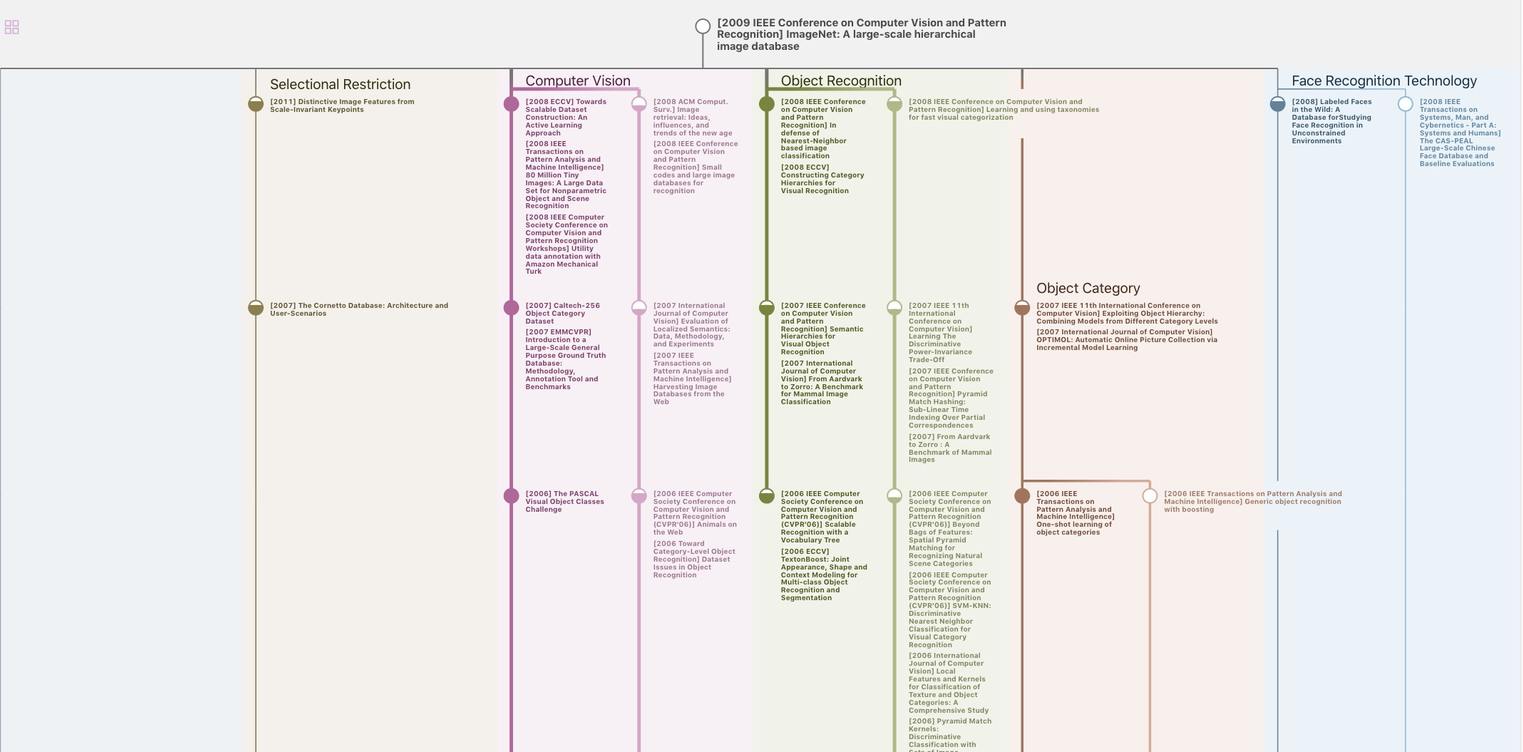
生成溯源树,研究论文发展脉络
Chat Paper
正在生成论文摘要