Adaptive Partial Multi-View Hashing for Efficient Social Image Retrieval
IEEE TRANSACTIONS ON MULTIMEDIA(2021)
摘要
Social networks allow users to actively upload images and descriptive tags, which has led to an explosive growth in the number of social images. Multi-view hashing is an efficient technique for supporting large-scale social image retrieval because of its desirable capabilities of encoding multi-view features into compact binary hash codes with extremely low storage costs and fast retrieval speeds. However, existing methods require multi-view features to be fully paired at both the offline model training and online query stages. This requirement cannot be easily satisfied for social image retrieval, where social images that lack descriptive tags are common in social networks. In this paper, we propose an Unsupervised Adaptive Partial Multi-view Hashing (UAPMH) method to handle the partial-view hashing problem for efficient social image retrieval. Specifically, the shared and view-specific latent representations of fully paired and partial-view images, respectively, are learned separately by an adaptive partial multi-view matrix factorization module within the identical semantic space. In particular, instead of adopting simple fixed view combination weights, we develop a parameter-free weight learning scheme to adaptively learn the weights to capture the view variations and the discriminative capabilities of different views. With such a design, our model can sufficiently exploit the available partial-view samples with separate hash code learning and effectively preserve the latent relations of images and tags in hash codes with semantic space sharing. Moreover, to avoid relaxing errors and improve the learning efficiency, binary hash codes are directly learned in a fast mode with simple and efficient operations. Finally, we extend UAPMH to the supervised learning paradigm as Supervised Adaptive Partial Multi-view Hashing (SAPMH) with the supervision of pair-wise semantic labels to further enhance the discriminative capability of hash codes. The experiments demonstrate the state-of-the-art performance of the proposed approaches on public social image retrieval datasets. Our source codes and testing datasets can be obtained at https://github.com/ChaoqunZheng/APMH.
更多查看译文
关键词
Image retrieval, Training, Semantics, Feature extraction, Kernel, Adaptation models, Binary codes, Social image retrieval, partial multi-view hashing, discrete optimization, adaptive weights
AI 理解论文
溯源树
样例
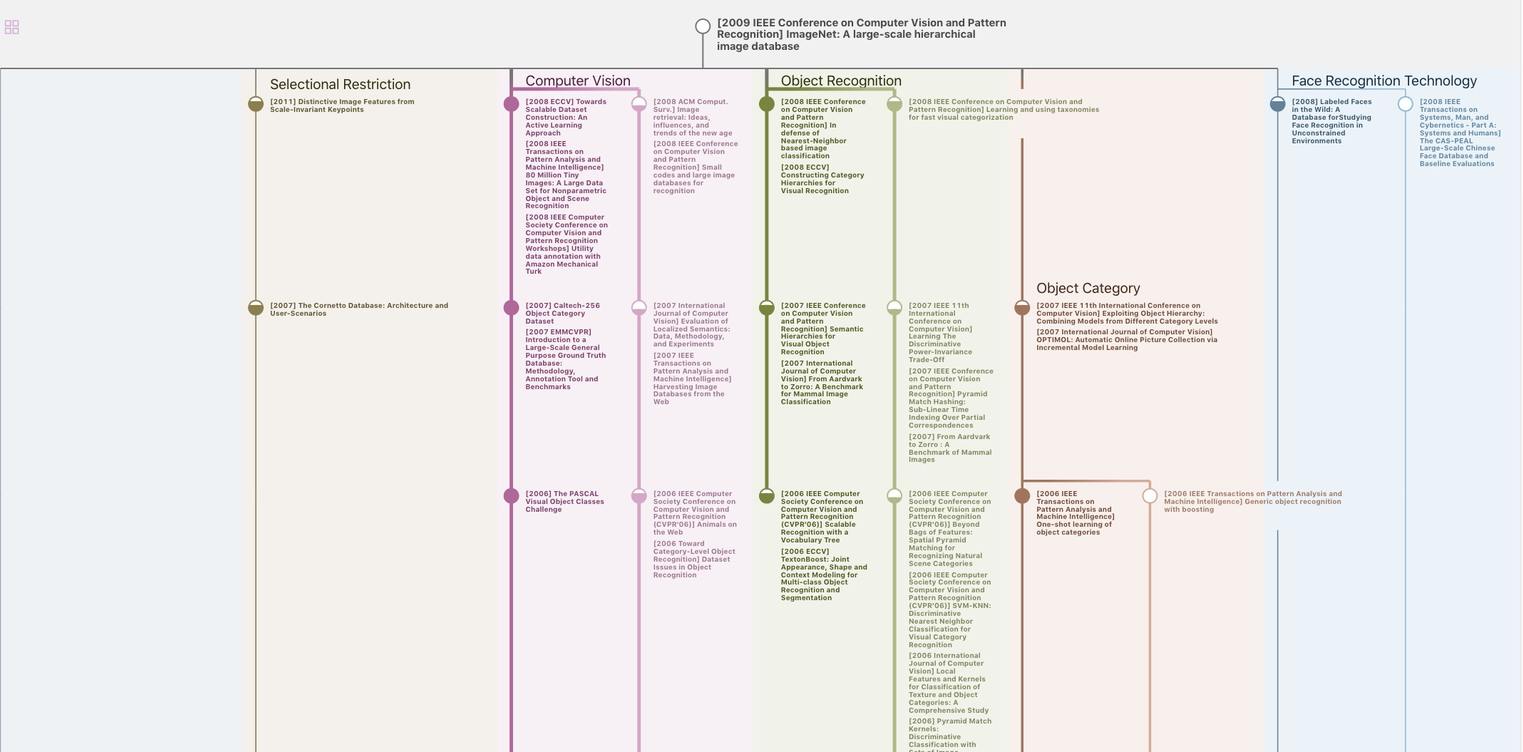
生成溯源树,研究论文发展脉络
Chat Paper
正在生成论文摘要