Using Machine Learning To Predict Self-Exclusion Status In Online Gamblers On The Playnow.Com Platform In British Columbia
INTERNATIONAL GAMBLING STUDIES(2021)
摘要
The identification of disordered gambling in the online environment may enable interventions to be targeted to those users experiencing harms. We tested the performance of machine learning in classifying online gamblers with and without a record of voluntary self-exclusion (VSE). We analyzed a one year dataset from PlayNow.com, the provincially owned online gambling platform in British Columbia, Canada. The primary model compared 2,157 gamblers with a record of VSE enrollment (6 months to 3 years) against 17,526 non-VSE controls, using 20 input variables of gambling behavior. Machine learning (random forest classifier) achieved an Area Under the Receiver Operating Characteristic curve (AUROC) of 0.75 (SD = 0.01). The input variable with the greatest predictive signal (based on feature importance values) was Variance in Money Bet per Session. Further analyses tested a logistic regression model as a benchmark, and tested the impact of key modeling decisions (including use of a balanced dataset, and data inclusion threshold). Across all models, machine learning algorithms were able to predict VSE status with performance between 0.65 and 0.76, using our behavioral inputs. These results provide proof-of-principle data for the applied use of behavioral tracking to identify disordered gambling, and highlight the importance of behavioral inputs reflecting betting variability.
更多查看译文
关键词
Online gambling, slot machines, gambling disorder, data science, machine learning, loss chasing
AI 理解论文
溯源树
样例
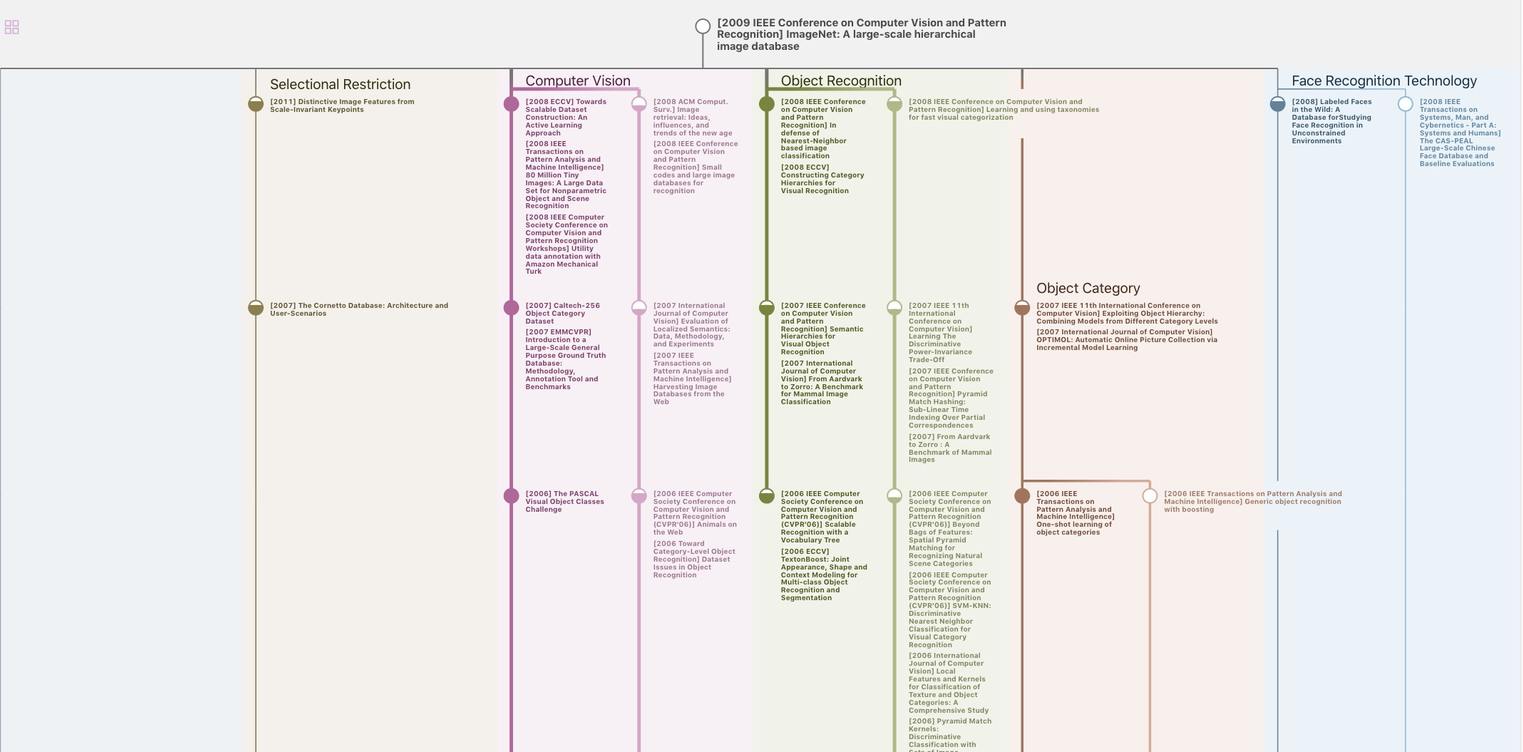
生成溯源树,研究论文发展脉络
Chat Paper
正在生成论文摘要