Robust hierarchical feature selection driven by data and knowledge
Information Sciences(2021)
摘要
Feature selection is facing great challenges brought by the enlarging label space and the inevitable noisy data. Flat feature selection methods fail to obtain a compact feature subset because of the numerous classes. In addition, these data-driven methods are sensitive to the data outliers. Fortunately, many practical tasks usually organize the classes by a hierarchical structure in a coarse-to-fine manner and can be solved by using the divide-and-conquer strategy. In this paper, we propose a hierarchical feature selection method driven by data and knowledge (HFSDK), which is robust to the data outliers and produces compact feature subsets by splitting the original large label space. Firstly, HFSDK decomposes a large-scale classification task into a group of small subclassification tasks with multiple granularities, which is driven by knowledge of the hierarchical class structure. Then, the corresponding datasets are constructed from the bottom to the top using the class labels of data, which is a data-driven process. Finally, robust and discriminative feature subsets are selected recursively for those subtasks by eliminating the data outliers and adding a semantic relation constraint. Experiments on six real-world datasets validate the superior performance of the proposed method.
更多查看译文
关键词
Feature selection,Hierarchical classification,Multi-granularity,Data-driven,Knowledge-driven
AI 理解论文
溯源树
样例
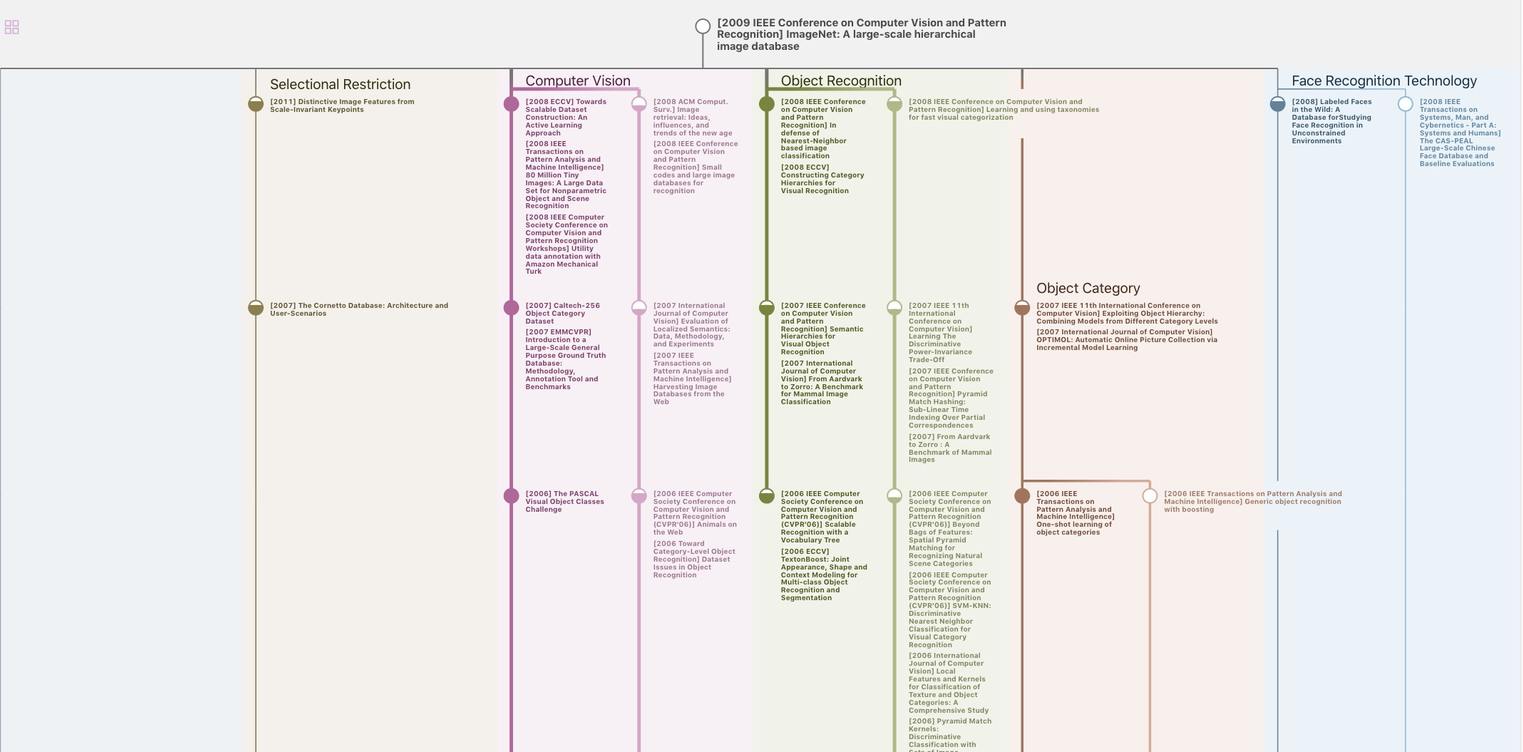
生成溯源树,研究论文发展脉络
Chat Paper
正在生成论文摘要