Managing Complex Datasets To Predict Bactrocera Oleae Infestation At The Regional Scale
Computers and Electronics in Agriculture(2020)
摘要
Predicting the occurrence of B. oleae infestation is needed in sustainable olive tree growing, for the application of preventive control strategies. In this study, machine learning (ML) algorithms were tested to predict the occurrence of B. oleae infestation. Regional-based and long-term (2002-2019) dataset on infestation collected by trained technicians in the sampling network (Tuscany, central Italy) was related to geographic variables, bioclimatic indices and the frequency of infestation in the previous year. Five ML algorithms were compared. Data collected from 2002 to 2017 were divided with an 80/20 proportion in "training" and "test 20%", considering the group "farm ID x year". In addition, data collected in 2018 and 2019 ("test 18-19") were also tested.The algorithm C5.0 was selected as the most suitable algorithm (AUC = 0.9) and used to predict the occurrence of B. oleae infestation. The following results were obtained: (i) a negative effect on the probability of infestation of the distance from the sea of the olive groves and of the frequency of infestation of the previous year and (ii) a positive effect of doy (day of the year), cumulative degree days and mean air temperature of the previous winter.The occurrence of infestation was predicted with an accuracy of 0.8 on "test 20%" and of 0.7 on "test 18-19". The potential of this methodology to predict the occurrence of B. oleae infestation in a short-time step, e.g., one week, needs to be tested in a real application, running the model weekly.
更多查看译文
关键词
Olive fruit fly, Machine learning, Decision support, IPM, ERA5-Land
AI 理解论文
溯源树
样例
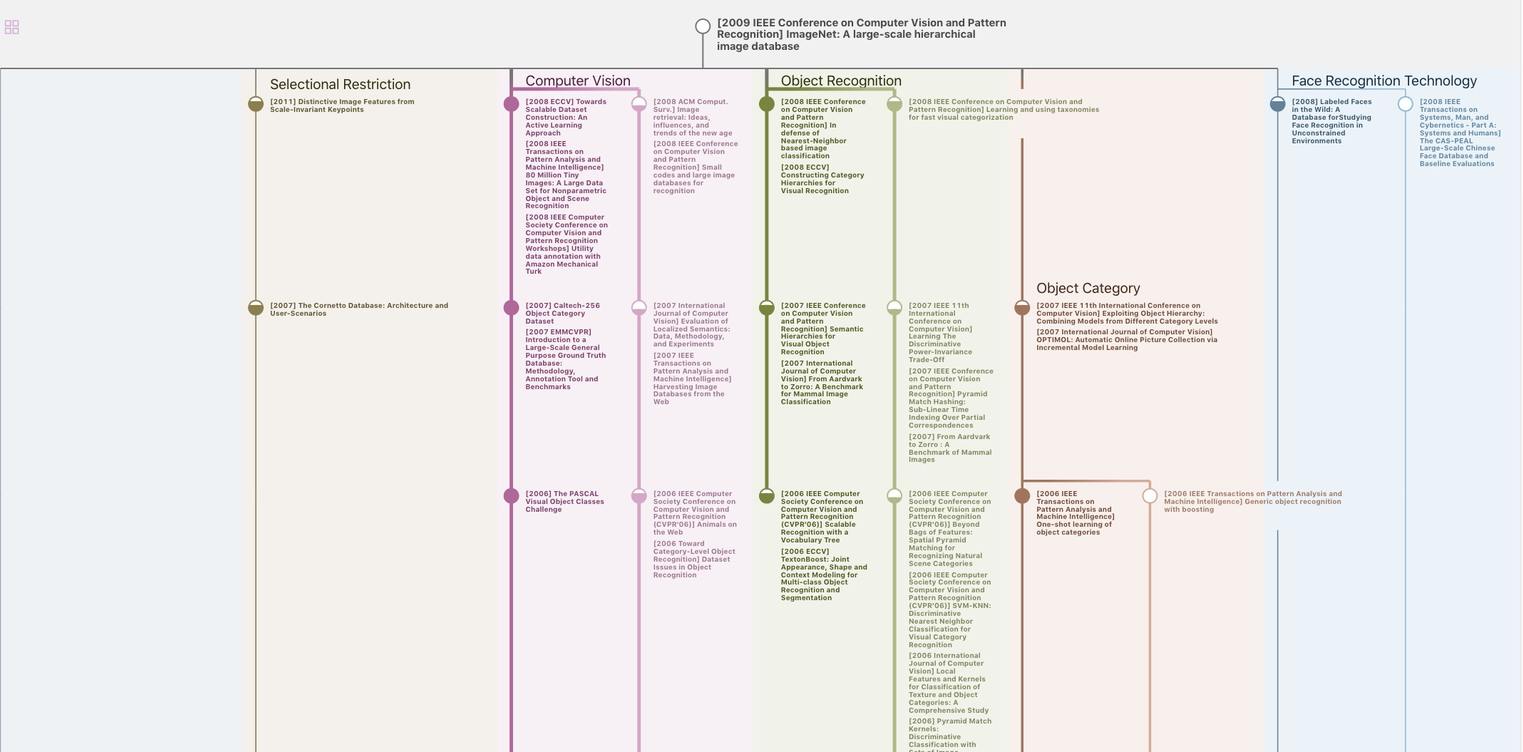
生成溯源树,研究论文发展脉络
Chat Paper
正在生成论文摘要