A Closer Look at Generalisation in RAVEN
European Conference on Computer Vision(2020)
摘要
Humans have a remarkable capacity to draw parallels between concepts, generalising their experience to new domains. This skill is essential to solving the visual problems featured in the RAVEN and PGM datasets, yet, previous papers have scarcely tested how well models generalise across tasks. Additionally, we encounter a critical issue that allows existing models to inadvertently ‘cheat’ problems in RAVEN. We therefore propose a simple workaround to resolve this issue, and focus the conversation on generalisation performance, as this was severely affected in the process. We revise the existing evaluation, and introduce two relational models, Rel-Base and Rel-AIR, that significantly improve this performance. To our knowledge, Rel-AIR is the first method to employ unsupervised scene decomposition in solving abstract visual reasoning problems, and along with Rel-Base, sets states-of-the-art for image-only reasoning and generalisation across both RAVEN and PGM.
更多查看译文
关键词
Visual reasoning, Representation learning, Scene understanding, Raven’s Progressive Matrices
AI 理解论文
溯源树
样例
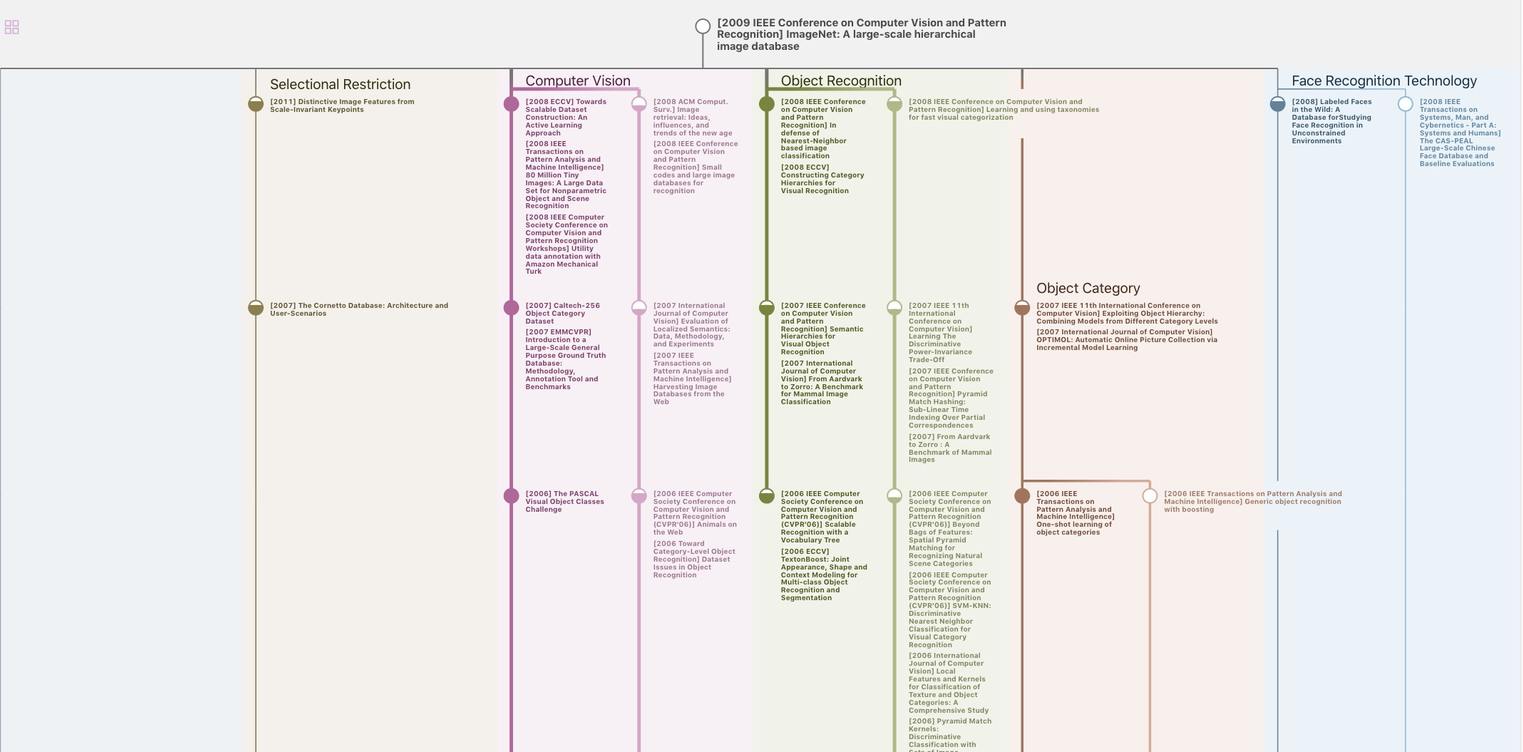
生成溯源树,研究论文发展脉络
Chat Paper
正在生成论文摘要