Faults Detection and Classification in Electrical Secondary Distribution Network Using Recurrent Neural Network
2020 6th IEEE International Energy Conference (ENERGYCon)(2020)
摘要
Deep learning approaches have provided relevant contributions to electrical network fault detection and classification. Nevertheless, few works of literature exist on fault detection and classification using deep learning approaches. Fault detection using deep learning is a challenge due to the presence of legacy monitoring devices in the transmission lines, which do not allow the direct deployment of the model. Deep learning involves training of the system, thus sufficient amount of data is required. The challenge arises in acquiring appropriate data from low voltage network that could accurately be used to model the network. This study provides an analysis of fault detection and classification in the electrical secondary distribution network using the Recurrent Neural Network approach. The model was developed using the dataset obtained from the utility company in Tanzania (TANESCO), between the year 2012 and 2019. The data was obtained from the Automatic Meter Reading installed in a low voltage distribution transformer where the voltage, current, and power values were captured after every 20 minutes. The results revealed that the accuracy is more than 90%, which proves the suitability and relevance of deep learning for fault detection and classifications in the secondary distribution network. Deep learning approaches are still in the industry; hence remain the focus for the new researches to explore its usefulness in different applications.
更多查看译文
关键词
Deep learning,fault classifications,faults detection,Secondary Distribution Network,IoT.
AI 理解论文
溯源树
样例
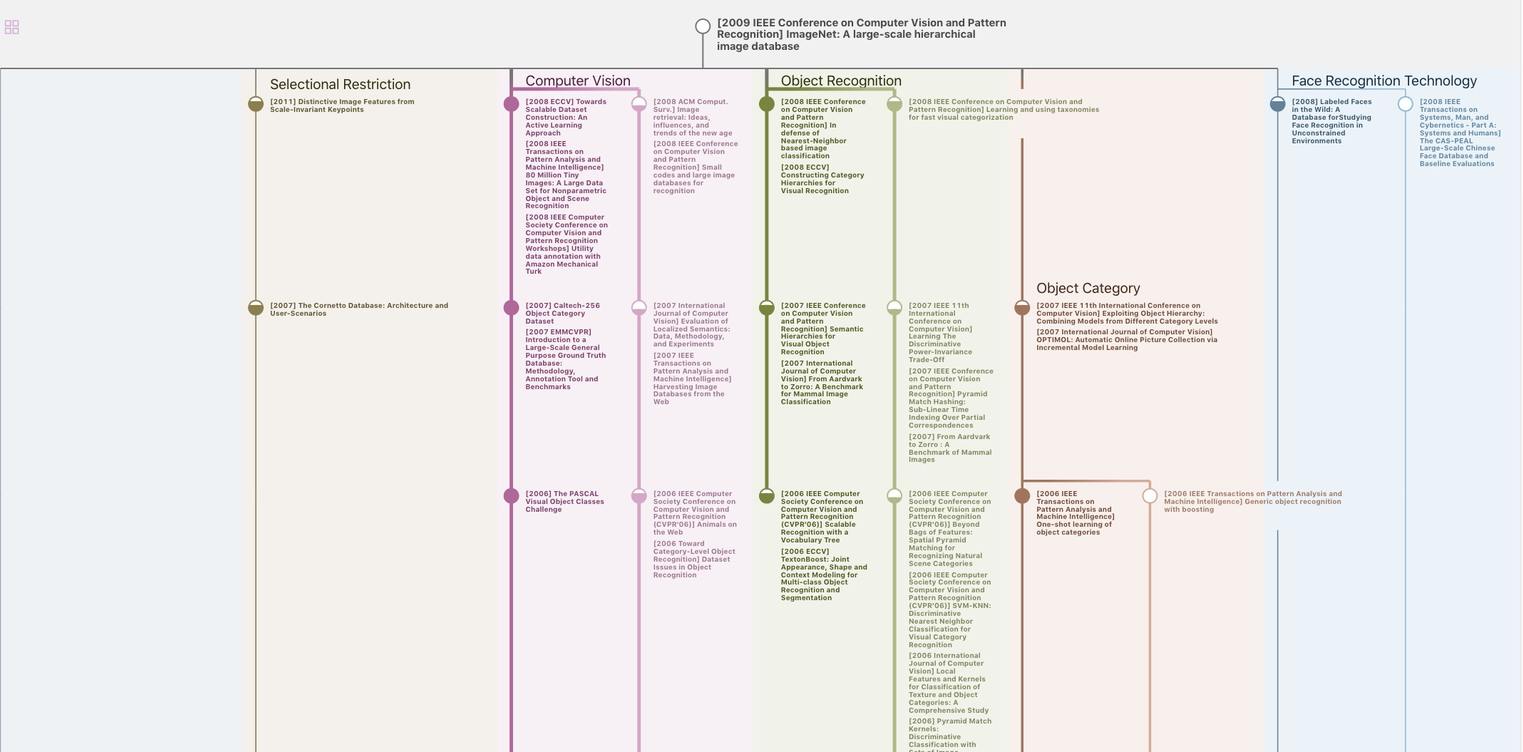
生成溯源树,研究论文发展脉络
Chat Paper
正在生成论文摘要