Machine Learning-Based Radio Coverage Prediction in Urban Environments
IEEE Transactions on Network and Service Management(2020)
摘要
AIM: Having a reliable prediction model of radio signal strength is an essential tool for planning and designing a radio network. Given a geographic region, and associated power estimates linked to the transmitter placements, our objective is to develop machine learning models to predict the strength of the radio signals. BACKGROUND: The propagation model is often used to determine the optimal location of radio transmitters in order to optimize the power coverage in a geographic area of interest. However, it is often a costly operation to obtain the exact power measurements over a region for a given set of transmitter locations. Therefore, fast prediction methods are needed to estimate the power values given limited data. METHODOLOGY: We consider a dataset consisting of simulated power at each point in an environment for a given set of transmitter locations. We experiment with various machine learning models, namely, generalized linear models (GLMs), neural networks (NNs), and k-nearest neighbor (KNN), to estimate the power values for a given transmitter placement. We investigate various feature engineering approaches to enhance the predictive performance of the machine learning models. RESULTS: We observe that employed feature engineering methods such as polynomial degrees and transmitter to cluster distances significantly improve the prediction accuracy. In particular, GLM model performance notably improves thanks to these extracted features, where mean absolute error (MAE) is reduced around 77% from 11.37 dB to 2.55 dB. We note that KNN with k = 2 and DNN models perform better than NN and GLM. KNN has the best performance with an average MAE of 0.65dB and also substantially faster to train than NN/DNN models. In addition, our analysis shows that, to train a well-performing machine learning model, it is sufficient to use a dataset consisting of measurements at a fraction of the potential transmitter locations in a given region. CONCLUSIONS: Machine learning methods are highly effective for the coverage prediction task. Using carefully engineered features, simple models such as GLMs and KNNs can be as effective as more complex ones, especially for small datasets.
更多查看译文
关键词
Machine learning,radio coverage,transmitter placement,k-nearest neighbor,generalized linear models,neural networks
AI 理解论文
溯源树
样例
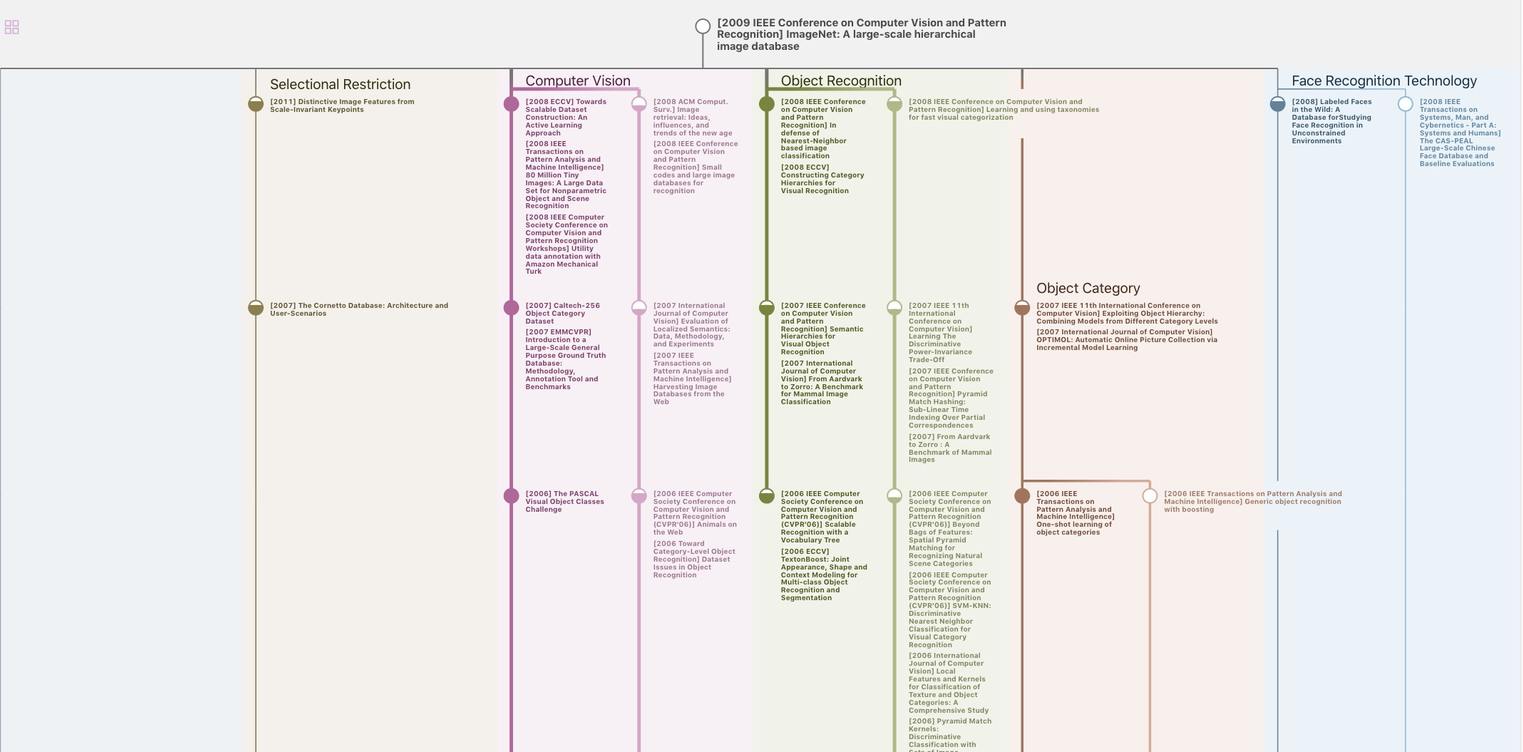
生成溯源树,研究论文发展脉络
Chat Paper
正在生成论文摘要