A Synthetic Penalized Logitboost to Model Mortgage Lending with Imbalanced Data
COMPUTATIONAL ECONOMICS(2020)
摘要
Most classical econometric methods and tree boosting based algorithms tend to increase the prediction error with binary imbalanced data. We propose a synthetic penalized logitboost based on weighting corrections. The procedure (i) improves the prediction performance under the phenomenon in question, (ii) allows interpretability since coefficients can get stabilized in the recursive procedure, and (iii) reduces the risk of overfitting. We consider a mortgage lending case study using publicly available data to illustrate our method. Results show that errors are smaller in many extreme prediction scores, outperforming a number of existing methods. Our interpretations are consistent with results obtained using a classic econometric model.
更多查看译文
关键词
Imbalanced, Boosting, Interpretation, Prediction, Binary
AI 理解论文
溯源树
样例
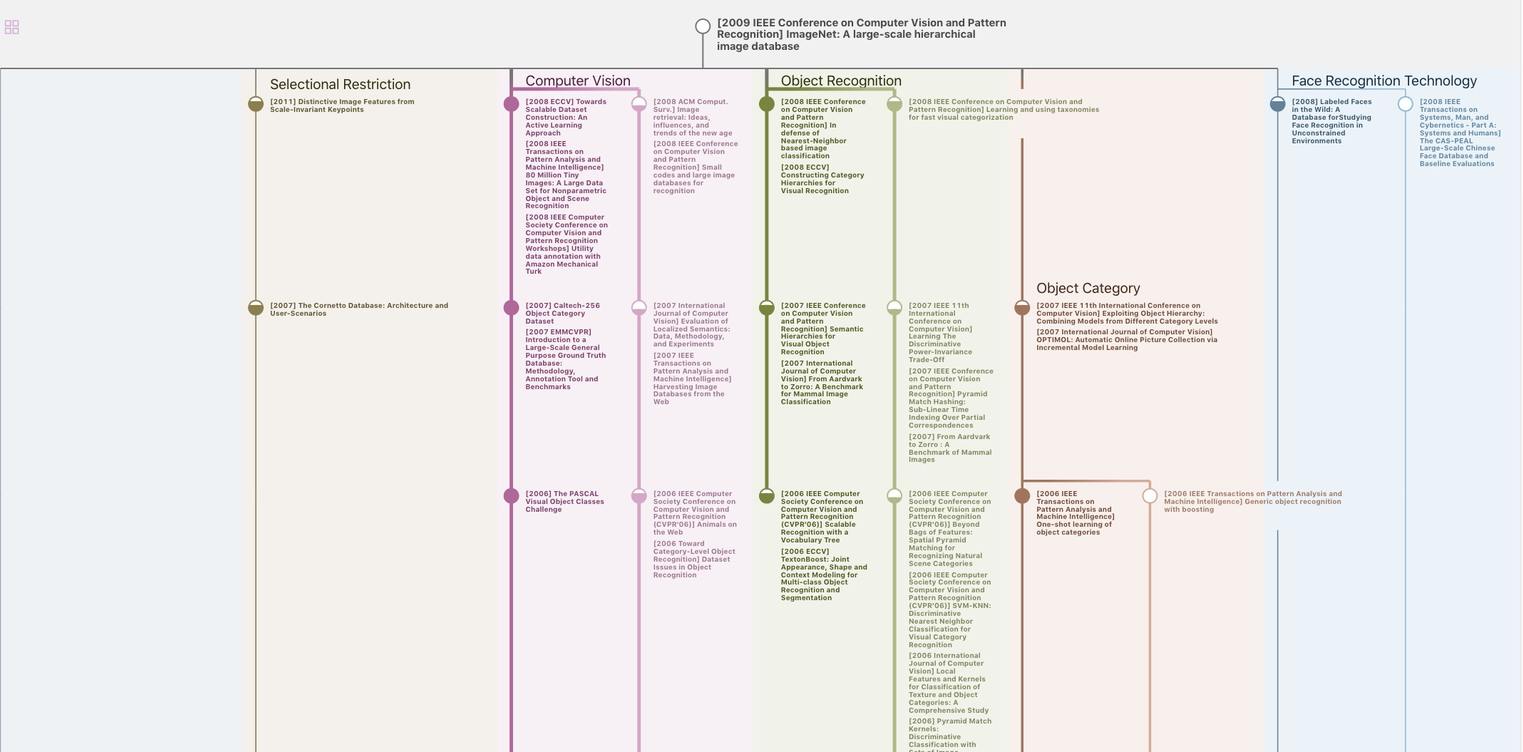
生成溯源树,研究论文发展脉络
Chat Paper
正在生成论文摘要