An Integrated Framework For Automatic Ontology Learning From Unstructured Repair Text Data For Effective Fault Detection And Isolation In Automotive Domain
COMPUTERS IN INDUSTRY(2020)
摘要
A real-life hierarchical classification system is developed to automatically extract a domain ontology from repair data collected during the warranty period of an original equipment manufacturer (OEM), e.g. automotive. Given the complex nature of products, failures may occur impacting consumers and to limit their adverse effect it is critical to react through effective fault detection and isolation. The key fault signals, such as failed parts, their associated symptoms, and the repair actions are latent in repair data. The overwhelming size of real-life data makes it impossible to read and curate a domain ontology manually in a reasonable time. In our work, a hierarchical classification system firstly classifies key phrases into technical and non-technical classes. Secondly, the technical phrases are classified into part, symptom, or action classes. Our system is deployed as a prototype and its performance is validated using real-life data. The system achieved the average F1 score of 0.82 and the new ontology is used in fault detection and isolation in seven different fault models. (c) 2020 Elsevier B.V. All rights reserved.ABSTRACT A real-life hierarchical classification system is developed to automatically extract a domain ontology from repair data collected during the warranty period of an original equipment manufacturer (OEM), e.g. automotive. Given the complex nature of products, failures may occur impacting consumers and to limit their adverse effect it is critical to react through effective fault detection and isolation. The key fault signals, such as failed parts, their associated symptoms, and the repair actions are latent in repair data. The overwhelming size of real-life data makes it impossible to read and curate a domain ontology manually in a reasonable time. In our work, a hierarchical classification system firstly classifies key phrases into technical and non-technical classes. Secondly, the technical phrases are classified into part, symptom, or action classes. Our system is deployed as a prototype and its performance is validated using real-life data. The system achieved the average F1 score of 0.82 and the new ontology is used in fault detection and isolation in seven different fault models. (c) 2020 Elsevier B.V. All rights reserved.
更多查看译文
关键词
Ontology learning, Automotive, Supervised machine learning, Decision support
AI 理解论文
溯源树
样例
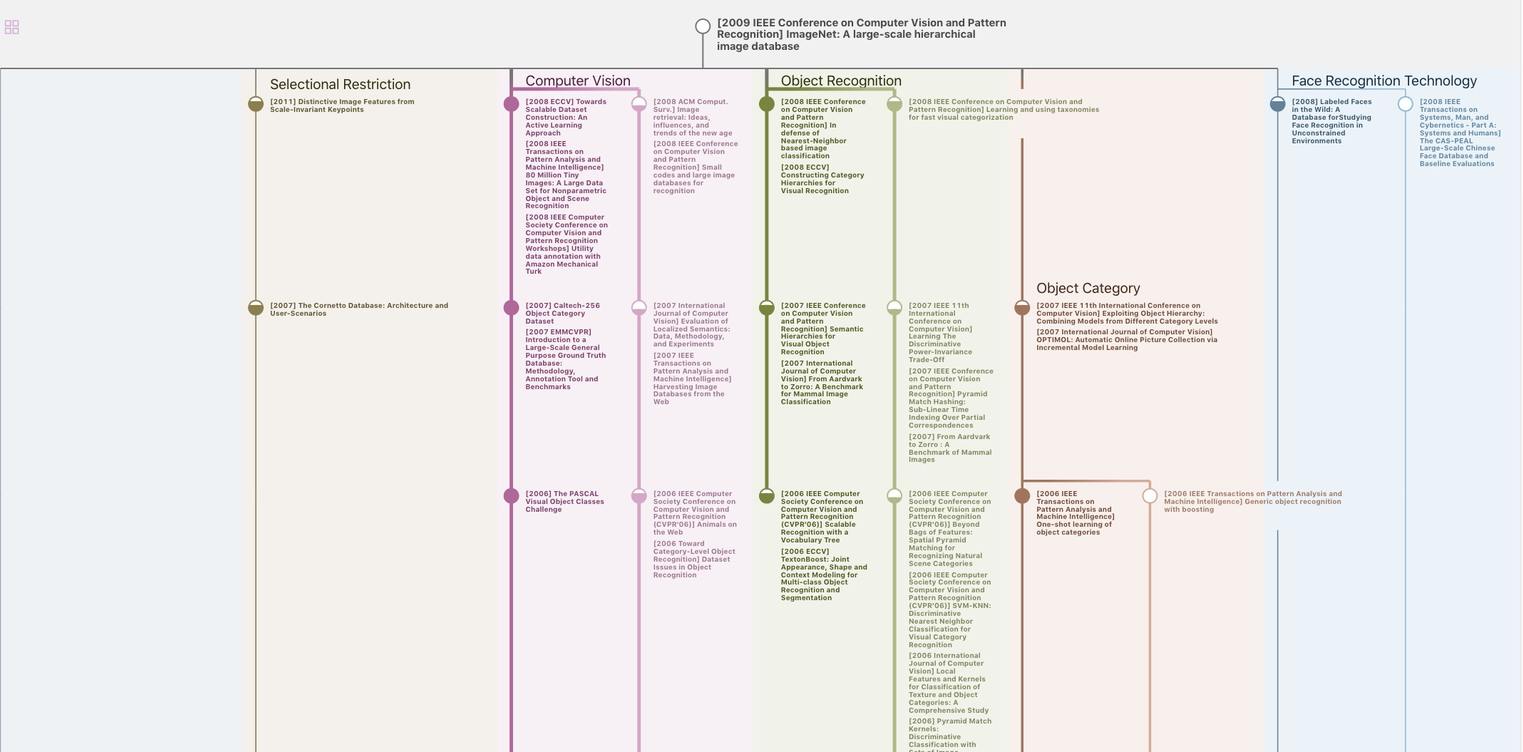
生成溯源树,研究论文发展脉络
Chat Paper
正在生成论文摘要