Leveraging ALOS-2 PALSAR-2 for Mapping Built-Up Areas and Assessing Their Vertical Component
IEEE JOURNAL OF SELECTED TOPICS IN APPLIED EARTH OBSERVATIONS AND REMOTE SENSING(2020)
摘要
Built-up areas extraction and characterization from remote sensing images is essential for monitoring urbanization and the associated challenges. This work presents a novel integrated classification framework building on the symbolic machine learning classifier and fully polarimetric Phased Array type L-band Synthetic Aperture Radar-2 (PALSAR-2) to derive both the extent and vertical components of built-up areas from the same scene. It also explores the complementarity between ascending and descending orbits of PALSAR-2 for built-up areas detection. The experimental results in Chicago and Tokyo cities with different landscape and characteristics of built-up areas demonstrate that the proposed generic method can achieve three main challenges of urban remote sensing: 1) enabling automated delineation of built-up areas at a spatial resolution of 5 m with a balanced accuracy of 85% using globally available low-resolution training data, 2) assessing the density of building height class with a root mean square error of 0.25, 0.034, and 0.032 for the low-rise, mid-rise, and high-rise building density class, respectively, and 3) dealing with the scattering components of buildings with different orientation angles by combining data from ascending and descending orbits for enhanced mapping of built-up areas.
更多查看译文
关键词
ALOS-2, built-up areas, Phased Array type L-band Synthetic Aperture Radar-2 (PALSAR-2), polarimetric SAR (PolSAR), symbolic machine learning, vertical component
AI 理解论文
溯源树
样例
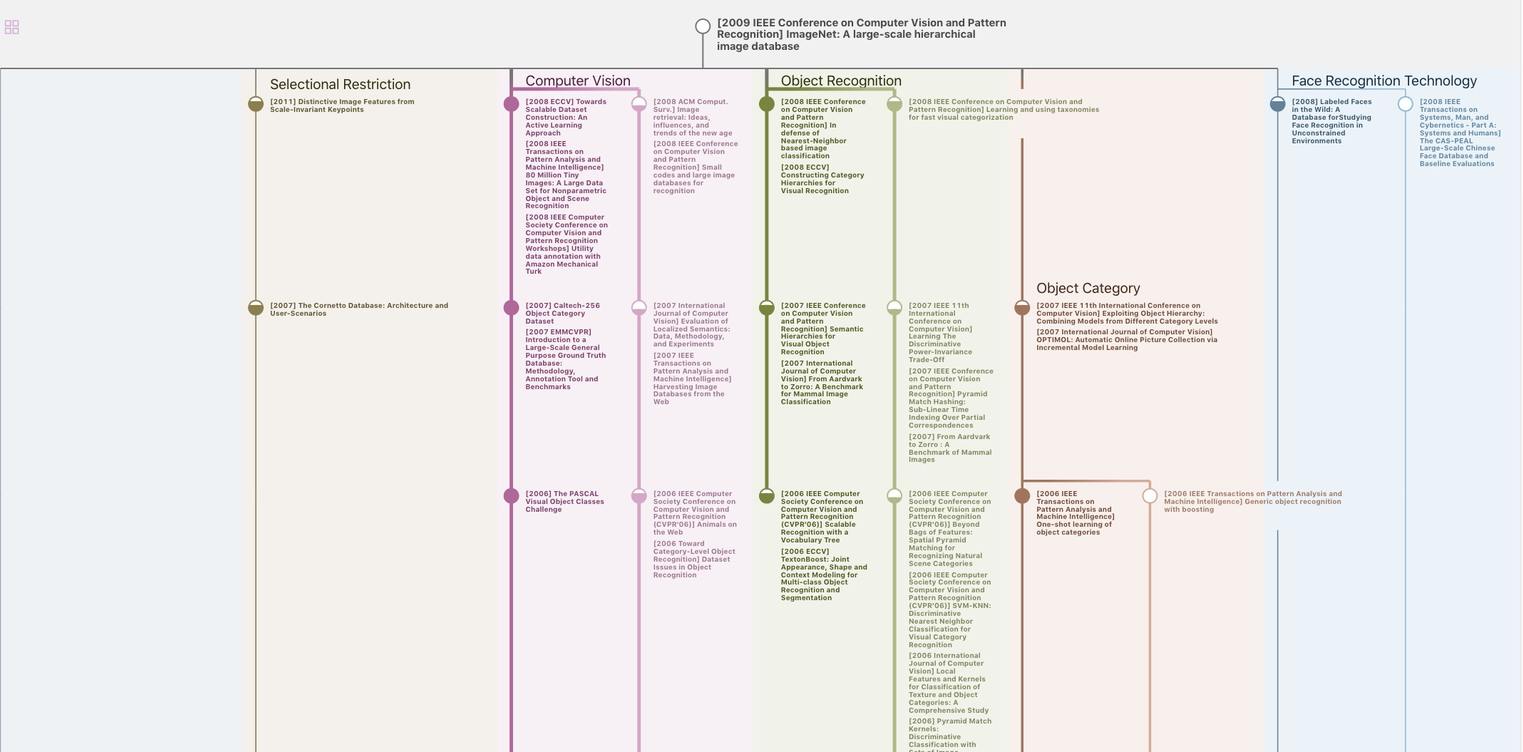
生成溯源树,研究论文发展脉络
Chat Paper
正在生成论文摘要