NeuroTIS: Enhancing the prediction of translation initiation sites in mRNA sequences via a hybrid dependency network and deep learning framework
Knowledge-Based Systems(2021)
摘要
Translation initiation site prediction is crucial to understand the mechanisms of gene expression and regulation. Many computational approaches have been proposed and achieved acceptable prediction accuracy. Although recent Convolutional Neural Network-based method effectively learn consensus motifs and shows remarkable prediction performance, this method could not fully exploit coding features which have been proved significant to the identification of translation initiation sites. Indeed, coding features often exhibit higher-order distant interactions among nucleotides and learning this kind of feature from uncharacteristic mRNA sequences without any explicit biological knowledge is difficult. This situation gets worse when given no coding labels. In viewing of these shortcomings, we propose a novel method for translation initiation sites prediction in mRNA sequences based on a hybrid dependency network and deep learning framework (NeuroTIS) which explicitly model label dependencies among coding region, between coding region and translation initiation site. Meanwhile, a Bidirectional Recurrent Neural Network and a Convolutional Neural Network are employed for effective learning and inference. The experimental results show that the proposed framework yields an excellent prediction performance on two benchmark gene datasets, which significantly outperforms existing state-of-the-art methods.
更多查看译文
关键词
Deep learning,Bioinformatics,Translation initiation sites prediction,Dependency network,Label dependency
AI 理解论文
溯源树
样例
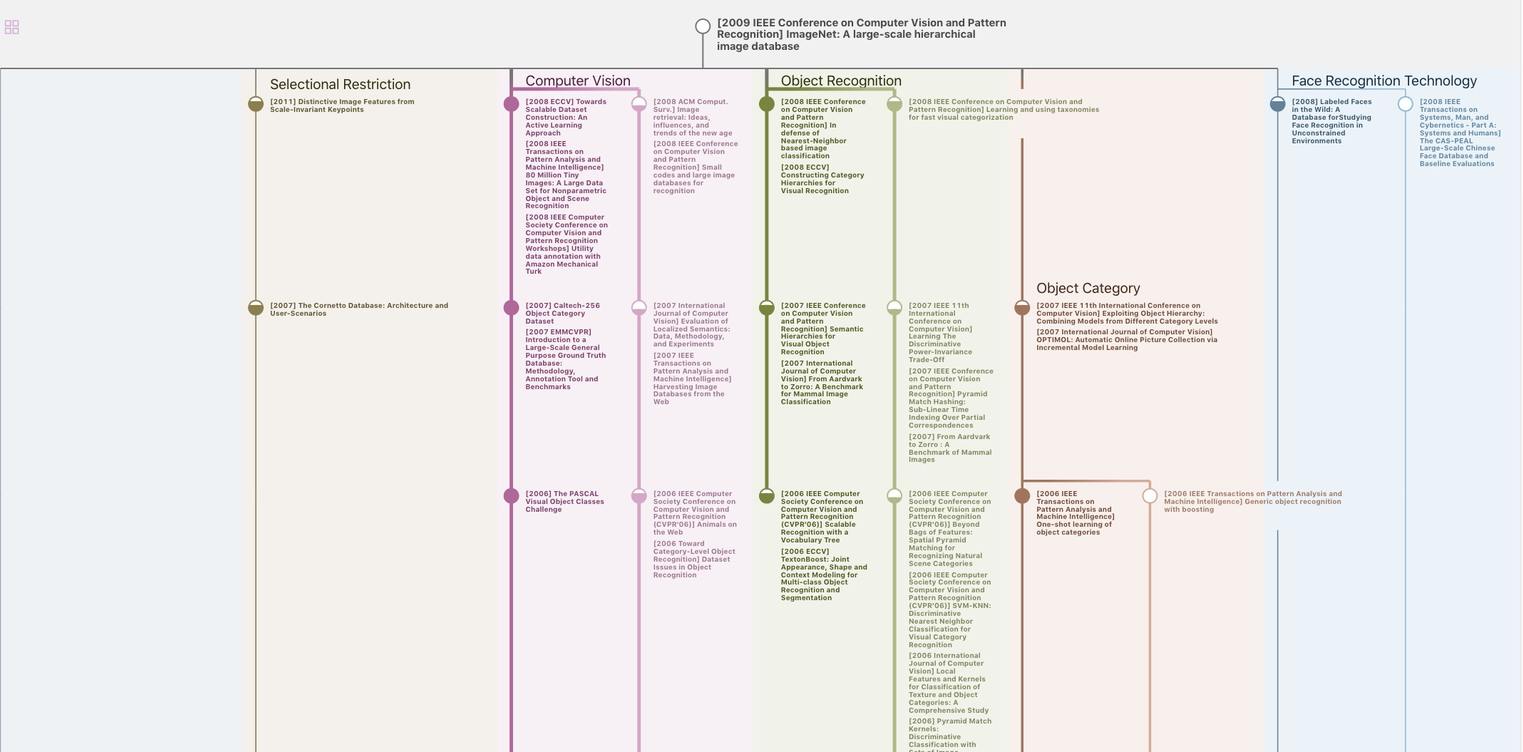
生成溯源树,研究论文发展脉络
Chat Paper
正在生成论文摘要