Graph-Temporal LSTM Networks for Skeleton-Based Action Recognition
chinese conference on pattern recognition(2020)
摘要
Human action recognition is one of the challenging and active research fields. Recently, spatio-temporal graph convolutions for skeleton-based action recognition have attracted much attention. Several strategies, such as temporal downsampling, convolution striding, and temporal pooling, are used to handle long action sequences. Recurrent neural networks are typically used for the processing of sequential data. In this paper, we propose a deep architecture that combines spatio-temporal graph convolution and graph-temporal long short-term memory (GT-LSTM) for skeleton-based human action recognition. Initially, topology-learnable spatio-temporal graph convolutions are applied to learn the local spatio-temporal features of graph nodes and adaptively evolve graph topologies. Then, GT-LSTM successively performs the spatio-temporal feature fusion with the node sequence and the temporal dimension, for the final recognition. Experimental results on the NTU RGB+D and Kinetics-Skeleton datasets demonstrate that the proposed architecture can effectively perform graph node information aggregation, graph topology evolution, and spatio-temporal graph feature fusion. liu2017skeleton.
更多查看译文
关键词
lstm,networks,action,graph-temporal,skeleton-based
AI 理解论文
溯源树
样例
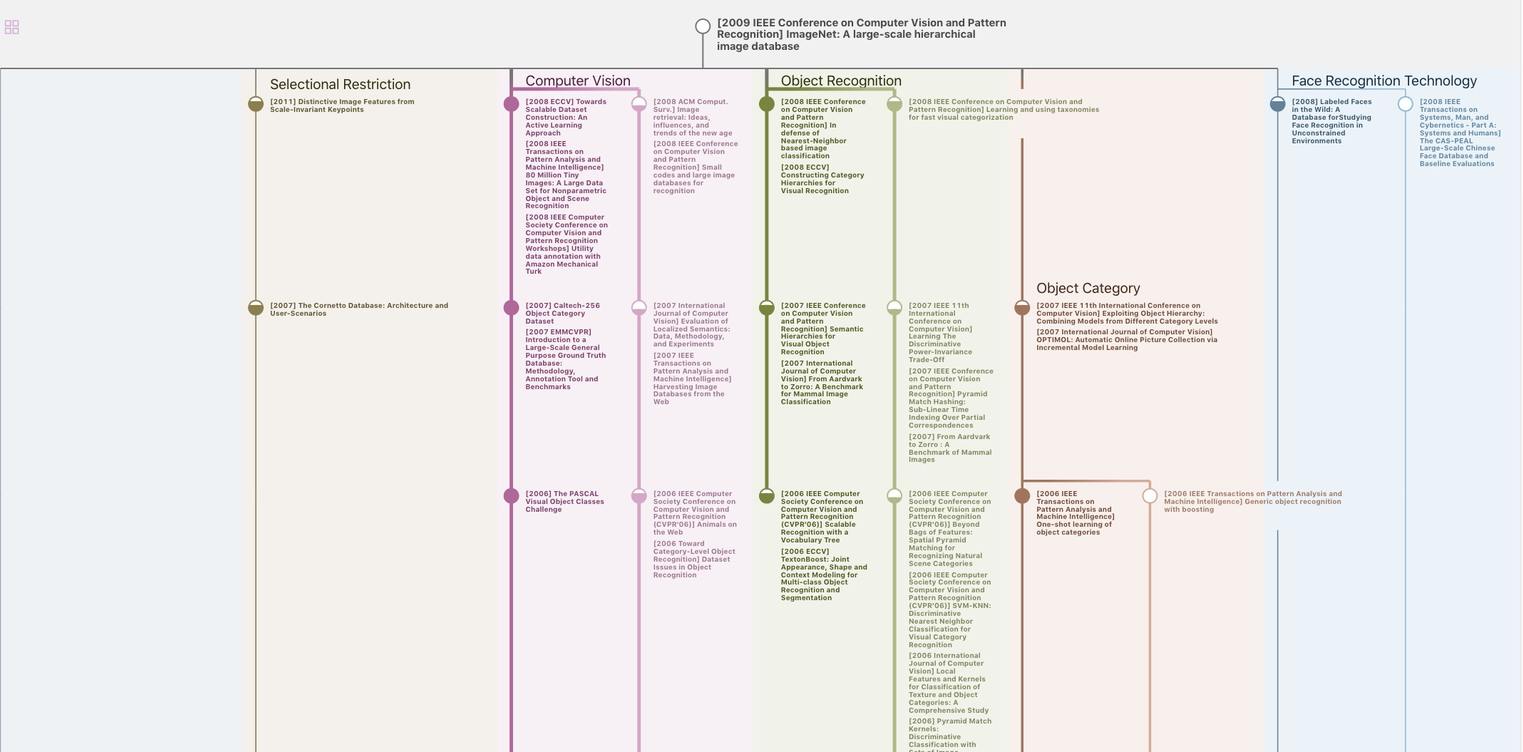
生成溯源树,研究论文发展脉络
Chat Paper
正在生成论文摘要