An estimation of pressure rise and heat transfer rate for hybrid nanofluid with endoscopic effects and induced magnetic field: computational intelligence application
The European Physical Journal Plus(2020)
摘要
This article reflects on the use of computational intelligence technique based on feed-forward nonlinear input–output artificial neural network for the prediction of pressure rise and heat transfer coefficient in peristaltic pumping of Ree–Eyring hybrid nanofluid through an endoscope. Gold nanoparticles are dispersed in Al 2 O 3 /blood nanofluid for hybridization with 0.02% and 0.05% volume fraction of Al 2 O 3 and Au, respectively. Estimation parameters include Ree–Eyring fluid parameter (0.05–0.2), magnetic parameter (1.0–3.0), radius ratio (0.7–0.9), and Brinkman number (0.2–0.6). Homotopy analysis method is employed to achieve experimental data from proposed mathematical model. Computational results for profiles of Δ P and Z are illustrated graphically, and it is found that M and α lead to amplify pumping while more pumping is needed in the case of rising χ . Moreover, heat transfer coefficient enhances with the increase in Br and χ . Experimental data are then validated using artificial neural network model. Levenberg–Marquardt backpropagation training algorithm is used in supervised learning. The degree of fitting the data is assessed on the bases of optimized decision threshold of mean square error. Network outputs are presented using error histogram, time-series responses, performance, and train-state plot which show that proposed technique is able to provide robust and very accurate output with least mean square error, strong correlation between target and network output with R = 1, trained with optimized weights at decreasing gradient, and a better convergence with small mu at each corresponding epochs. Further, comparison of analytical results with previously published literature shows a good agreement.
更多查看译文
AI 理解论文
溯源树
样例
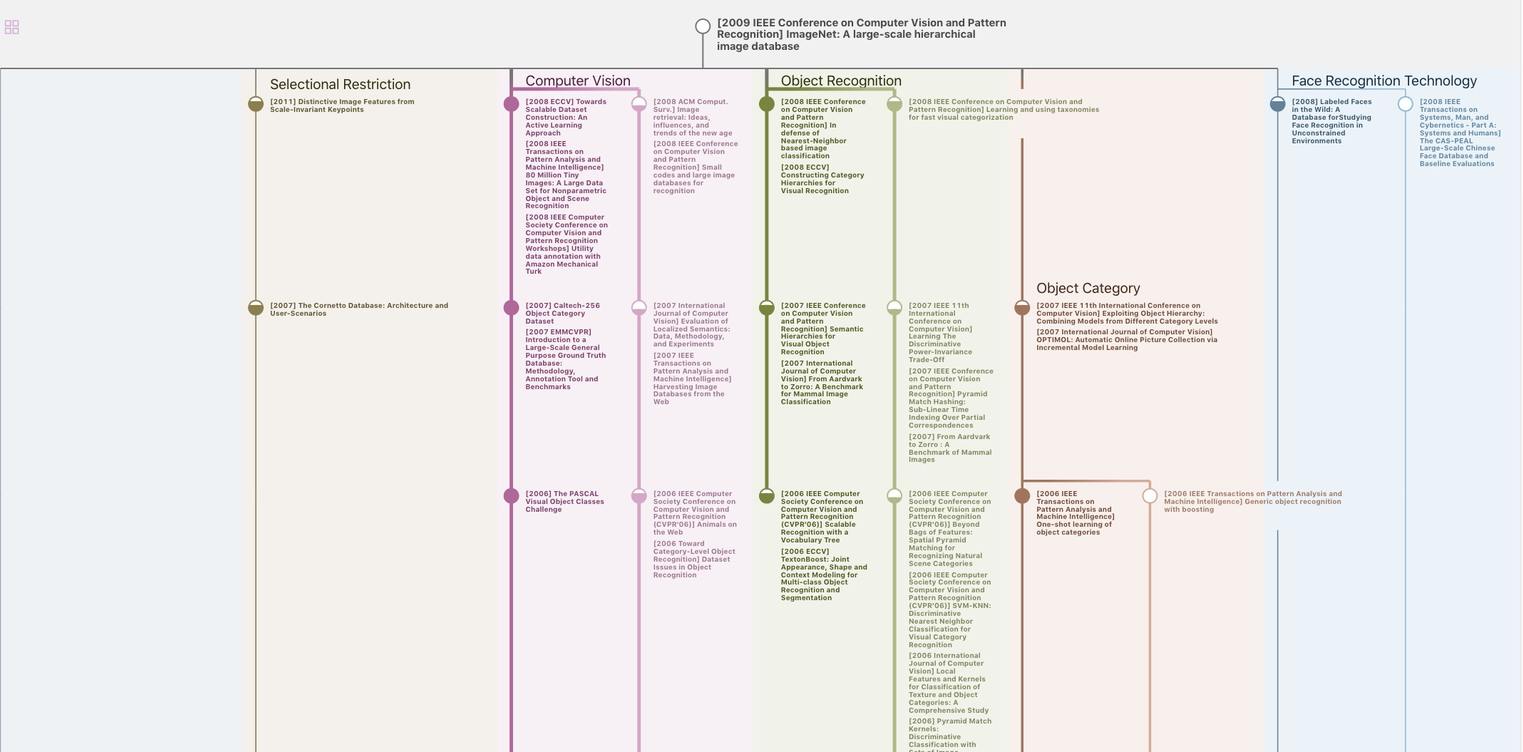
生成溯源树,研究论文发展脉络
Chat Paper
正在生成论文摘要