Hyperspectral image classification based on local binary pattern and broad learning system
INTERNATIONAL JOURNAL OF REMOTE SENSING(2020)
摘要
In the hyperspectral classification, the combination of spectral information and spatial information has received more attention. Especially in the deep learning methods, massive spatial-spectral features which are helpful for improving the classification performance can be extracted. However, these methods suffer from time-consuming training process because of a great number of network parameters. In this paper, a novel architecture based on locality preserving projection (LPP), local binary pattern (LBP) and broad learning system (BLS) (LPP_LBP_BLS) for hyperspectral image (HSI) classi?cation is proposed, which mainly consists of three parts. First, LPP is applied to preserve the inherent local structure during dimensionality reduction of HSI in order to remove the redundant information in the spectral domain. Second, LBP is performed to extract the local grey-scale and rotation invariant texture features in each spectral reflectance band of the reduced-dimensional pixel in the spatial domain. It can fully utilize the spatial information of HSIs. Finally, BLS calculates the predictive sample labels according to the mapped feature nodes, enhancement nodes, and optimal connecting weights which are achieved through the normalized optimization of L2-norm solved by ridge regression approximation. LPP_LBP_BLS is beneficial for classification by combining spectral signatures with spatial information effectively. Experimental results demonstrate that the proposed architecture achieves above 99% classi?cation accuracy on Indian Pines dataset and Salinas dataset and above 97% classi?cation accuracy on Pavia University dataset, which outperforms other deep learning and traditional classification approaches.
更多查看译文
AI 理解论文
溯源树
样例
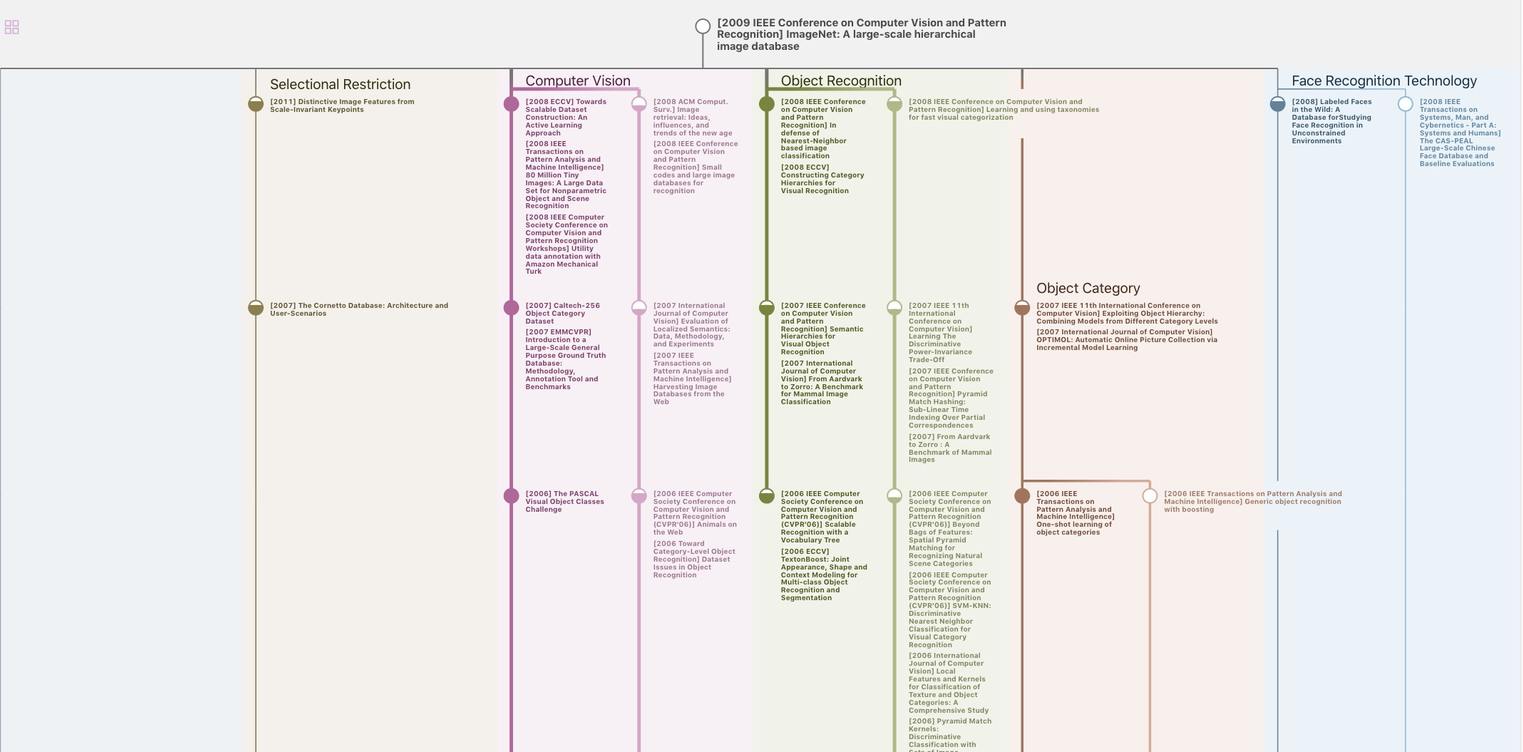
生成溯源树,研究论文发展脉络
Chat Paper
正在生成论文摘要