Power Optimization in Device-to-Device Communications: A Deep Reinforcement Learning Approach With Dynamic Reward
IEEE Wireless Communications Letters(2021)
摘要
Device-to-Device (D2D) communication can be used to improve system capacity and energy efficiency (EE) in cellular networks. One of the critical challenges in D2D communications is to extend network lifetime by efficient and effective resource management. Deep reinforcement learning (RL) provides a promising solution for resource management in wireless communication systems. This letter aims to maximise the EE while satisfying the system throughput constraints as well as the quality of service (QoS) requirements of D2D pairs and cellular users in an underlay D2D communication network. To achieve this, a deep RL based dynamic power optimization algorithm with dynamic rewards is proposed. Moreover, a novel algorithm with two parallel deep Q networks (DQNs) is designed to maximize the EE of the considered network. The proposed deep RL based power optimization method with dynamic rewards achieves higher EE while satisfying the system throughput requirements.
更多查看译文
关键词
Device-to-device communication,deep reinforcement learning,energy efficiency,power control
AI 理解论文
溯源树
样例
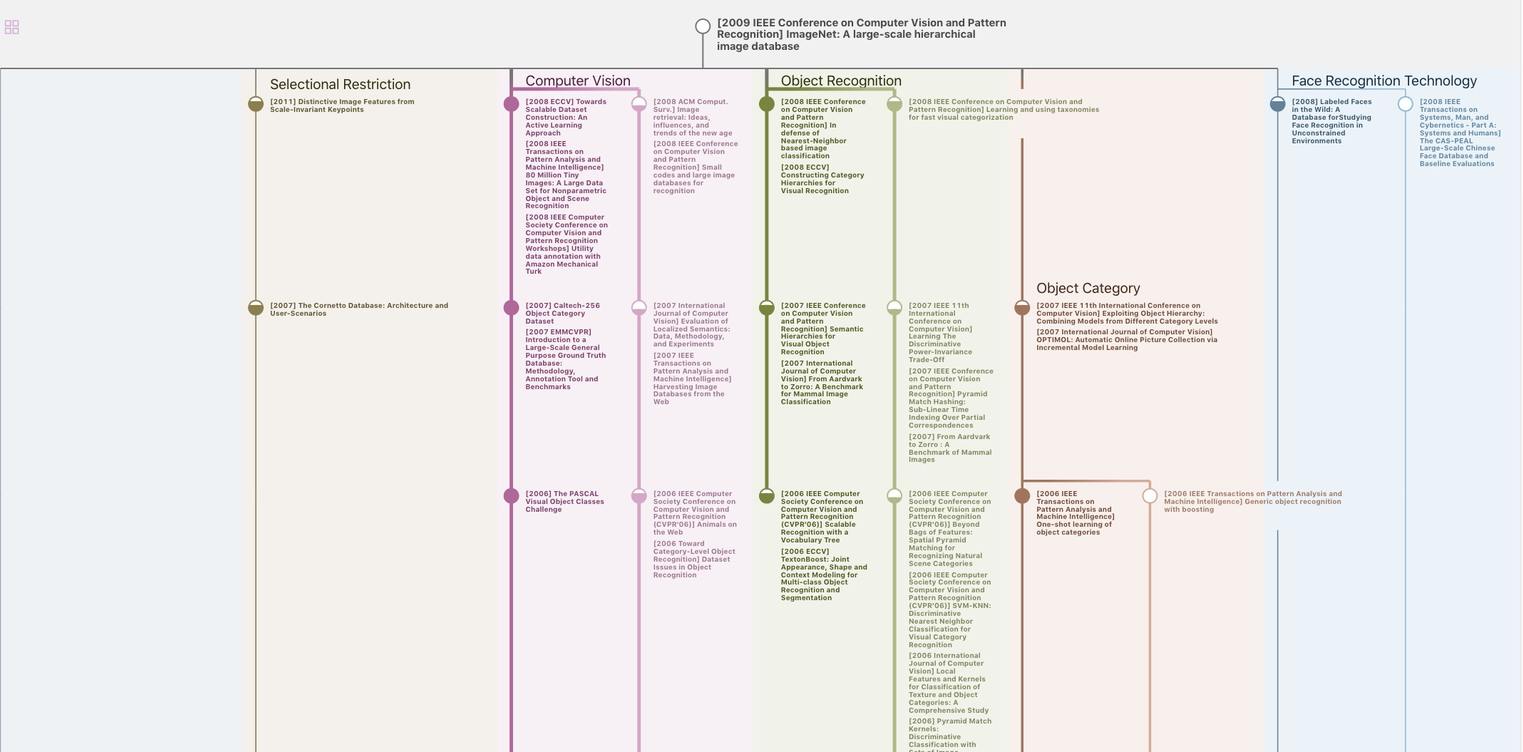
生成溯源树,研究论文发展脉络
Chat Paper
正在生成论文摘要