Sleep State Classification Using Power Spectral Density And Residual Neural Network With Multichannel Eeg Signals
APPLIED SCIENCES-BASEL(2020)
摘要
This paper proposes a classification framework for automatic sleep stage detection in both male and female human subjects by analyzing the electroencephalogram (EEG) data of polysomnography (PSG) recorded for three regions of the human brain, i.e., the pre-frontal, central, and occipital lobes. Without considering any artifact removal approach, the residual neural network (ResNet) architecture is used to automatically learn the distinctive features of different sleep stages from the power spectral density (PSD) of the raw EEG data. The residual block of the ResNet learns the intrinsic features of different sleep stages from the EEG data while avoiding the vanishing gradient problem. The proposed approach is validated using the sleep dataset of the Dreams database, which comprises of EEG signals for 20 healthy human subjects, 16 female and 4 male. Our experimental results demonstrate the effectiveness of the ResNet based approach in identifying different sleep stages in both female and male subjects compared to state-of-the-art methods with classification accuracies of 87.8% and 83.7%, respectively.
更多查看译文
关键词
EEG signals, deep learning, sleep stage, classification, machine learning
AI 理解论文
溯源树
样例
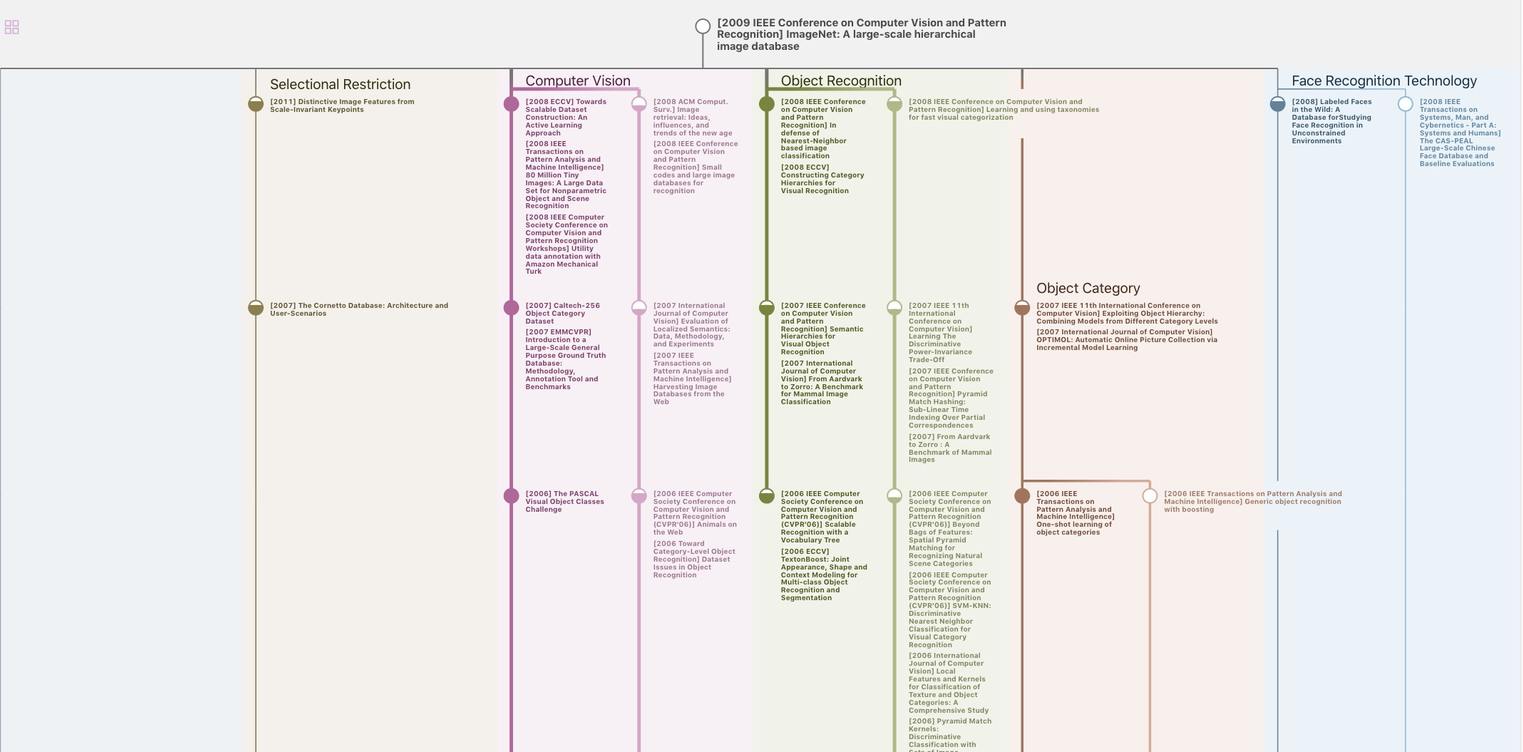
生成溯源树,研究论文发展脉络
Chat Paper
正在生成论文摘要