A Framework For Adapting Dnn Speaker Embedding Across Languages
IEEE-ACM TRANSACTIONS ON AUDIO SPEECH AND LANGUAGE PROCESSING(2020)
摘要
Language mismatch remains a major hindrance to the extensive deployment of speaker verification (SV) systems. Current language adaptation methods in SVmainly rely on linear projection in embedding space; i.e., adaptation is carried out after the speaker embeddings have been created, which underutilizes the powerful representation of deep neural networks. This article proposes a maximum mean discrepancy (MMD) based framework for adapting deep neural network (DNN) speaker embedding across languages, featuring multi-level domain loss, separate batch normalization, and consistency regularization. We refer to the framework as MSC. We show that (1) minimizing domain discrepancy at both frame- and utterance-levels performs significantly better than at utterance-level alone; (2) separating the source-domain data from the target-domain in batch normalization improves adaptation performance; and (3) data augmentation can be utilized in the unlabelled target-domain through consistency regularization. By combining these findings, we achieve an EER of 8.69% and 7.95% in NIST SRE 2016 and 2018, respectively, which are significantly better than the previously proposedDNNadaptation methods. Our framework alsoworks wellwith backend adaptation. By combining the proposed framework with backend adaptation, we achieve an 11.8% improvement over the backend adaptation in SRE18. When applying our framework to a 121-layer Densenet, we achieved an EERof 7.81% and 7.02% in NIST SRE 2016 and 2018, respectively.
更多查看译文
关键词
Neural networks, Adaptation models, Kernel, Task analysis, Speech recognition, Speech processing, NIST, Speaker verification (SV), domain adaptation, data augmentation, maximum mean discrepancy, transfer learning
AI 理解论文
溯源树
样例
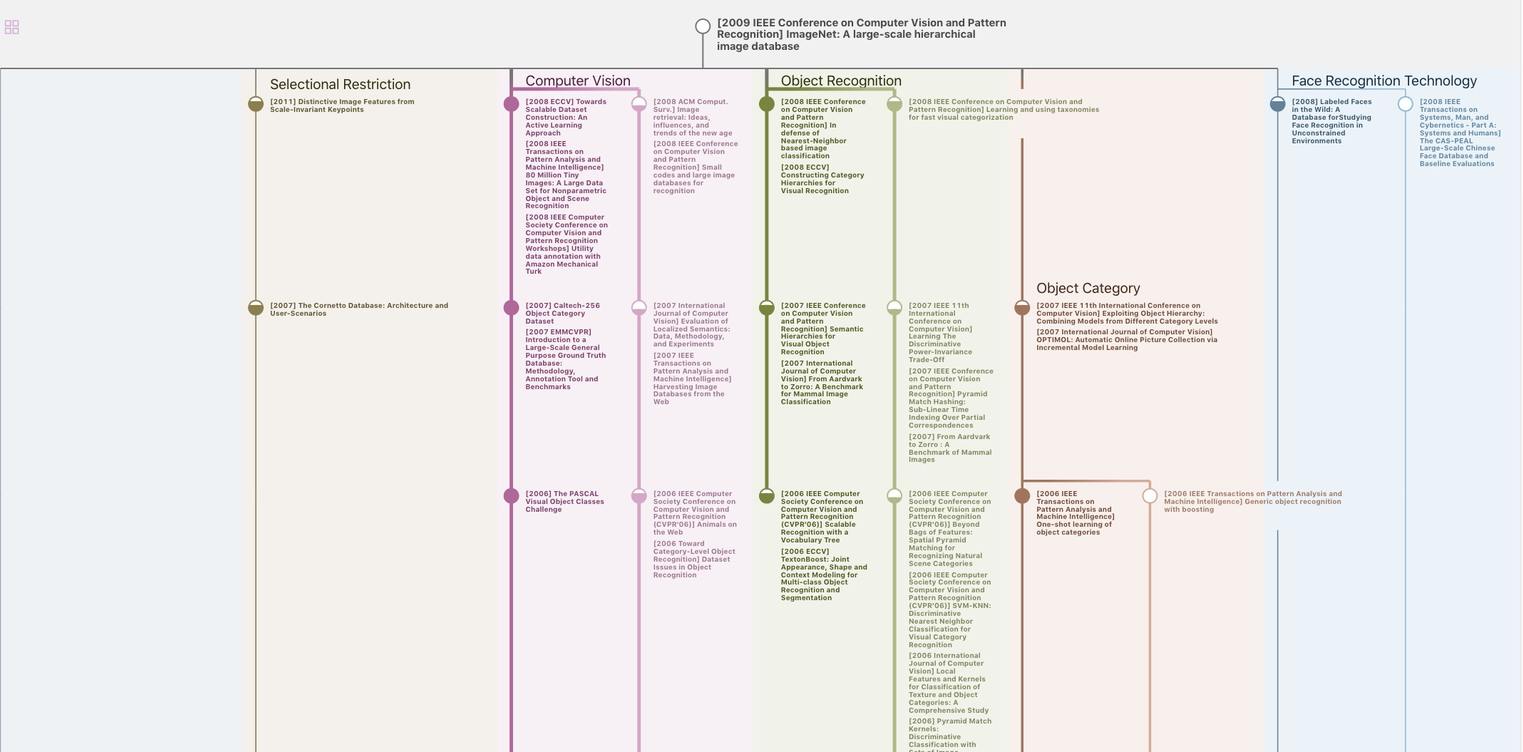
生成溯源树,研究论文发展脉络
Chat Paper
正在生成论文摘要